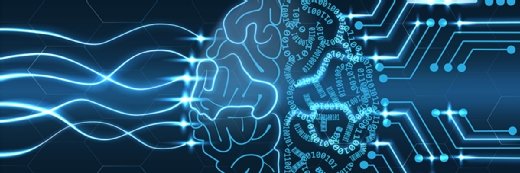
Laurent - stock.adobe.com
Where are we with machine translation in AI?
Machine translation has received a boost from cutting-edge technology like deep learning but continues to struggle with the complexities and nuances of human languages.
One of the challenges that artificial intelligence has been increasingly capable of addressing is machine translation -- the process of using an artificial intelligence to translate from one human language to another. Also known as MT, automatic translation or computer translation, the ultimate goal of machine translation in AI is being able to smoothly, automatically and accurately translate one spoken or written language to another.
While the concepts behind machine translation are easy to grasp, however, the process of creating fully automated language translation is extraordinarily complex. Human languages have a wide variety of nuances, slang and similar words. Languages even have single words that have multiple meanings. A computer must be able to process and understand all these intricacies in order to provide an accurate translation.
In order to make this happen, a variety of technologies need to come together, including cutting-edge technology such as deep learning, artificial intelligence, big data and linguistic analysis. Some of the technology behind MT has existed for a while, such as cloud computing, cloud storage and web APIs, and these are all being used with the new technology to help power translation.
The latest evolution of machine translation
Today there are three main types of machine translation available: rule-based machine translation (RBMT), statistical machine translation and hybrid systems that combine the two. As the name implies, rule-based machine translation uses a set of rules to determine how something should be translated. Essentially it takes two dictionaries and uses them both to create a translation. Over the past few decades, most implementations of machine translation in AI use rule-based machine translation as it was the first to achieve reliable results. It is great for technical work as it provides literal translations and has a well-established history.
However, dictionaries can only provide so much translation as there are plenty of words that don't translate well from one language to another. To help AI learn to translate better, a new translation method was introduced, known as Statistical Machine Translation. Instead of using just dictionaries to translate, these computers learn translations by examining bilingual texts.
With the evolution of AI, RBMT approaches no longer dominate the field. More implementations have moved toward statistical machine translation or hybrid systems as they provide translation based off real documents instead of pre-input dictionaries. Hybrid machine translation combines these two approaches together. Dictionaries serve as the basis for the translation while the computer is also able to learn from bilingual texts to get the nuances of human language.
As machine learning-based translation is powered by large amounts of data, it should be little surprise that large cloud vendors are leading the way with powerful machine translation technology. Amazon, Google, Microsoft, Facebook and others have built powerful machine translation capabilities that leverage the limitless conversations happening on their platforms in a wide range of languages. Google translate has seen widespread usage, although with significant accuracy challenges. Facebook unveiled an unsupervised learning approach to machine translation that has shown increased accuracy. Amazon also released machine translation on their Amazon Web Services (AWS) platform.
In addition to the big cloud vendors, there are at least 45 machine translation companies operating around the world. Some vendors focus on translation services aimed at translating professional documents. Other firms leverage the use of humans-in-the-loop to accentuate machine translation or handle cases where accuracy is below acceptable thresholds. Services that provide machine translation for companies and professional purposes have become increasingly popular. These fully automated services allow organizations to save a lot of money and time when it comes to getting content translated. Machine translation in AI can handle over three times the amount of work than a human is able to handle. This even includes having a human editor go over the machine's work.
Limitations of machine translation
Notably, machine translation still is unable to handle the nuances of everyday speech, especially around specialized language used in areas such as legal or medical documents. These are documents where an incorrect translation can cause serious problems. For example, medical terms can be very hard to translate from one language to another and many concepts in mental health don't have an exact translation between various languages.
Another area that currently can't use machine translation is on literary writing and works of fiction. When writing these, authors use more imagery than straightforward writing. A computer has a hard time grasping ideas such as humor or sarcasm and can't take that into account when translating a document. That means that while ML may be fairly accurate at translating a workplace policy manual, it would have a hard time providing an accurate translation of novels. Slang and culture-specific dialects can also cause problems for machine learning as it doesn't know how to translate these accurately from one language to another. Sometimes there aren't even ways to translate slang, as it doesn't carry over to another language. Calling someone an octopus in Japanese is offensive, but in America you would probably just think the person is weird.
While machine translation systems are getting increasingly better, those with experience call for humans to remain in the loop for any translation activities that have business or personal impact. Humans should still review machine-facilitated translations and find the specific areas where translation hasn't quite matched and correct it. The plus side of having a human in the loop is that AI-enabled SMT approaches can use this human feedback as part of the machine learning system so that it can improve translations.
Machine translation in AI has come a long way since the first days of rules-based translators. With AI, we are now able to take far more information into account when providing translations. New technologies and learning methods will help machine translation improve as time goes on. As mentioned earlier, translation is a hard problem, but machines are increasingly becoming better at it.