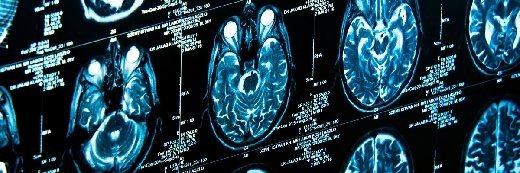
svedoliver - Fotolia
The uses of AI in medical imaging
Medical imaging is being deployed with the assistance of AI in order to reduce the strain on medical professionals and hopefully improve patient care.
From the invention of the basic X-ray in 1895 to the suite of medical imaging tools we have available today, technology has played a big part in advancing the medical field. As consumer trust grows, medical professionals are turning to technology to help enhance the quality of care that patients receive. AI assistants and technology can act as a second set of eyes for various tasks and help professionals perform increasingly complex procedures. One of the top procedures getting a boost from technology is medical imaging.
How does AI help with medical imaging?
In recent years, AI has been used in multiple ways to help with medical imaging of all kinds. Currently, one of the biggest use cases for AI is to assist in the analysis of images. This includes X-ray images, ultrasounds, MRI, positron emission tomography and computerized tomography. Until recently, medical professionals needed to sort and look through every medical image, which was both time-consuming and prone to errors. To assist with this task, AI in medical imaging is being trained to sift through the data and images and make initial observations about possible fractures, tumors or other potential issues.
The process of training an AI to read medical images and get to near-human levels of accuracy is becoming easier and more accessible thanks to vast amounts of data, pretrained networks and transfer learning. For a hospital, medical system or similar institution to get started, it needs access to some amount of data. Then, in a supervised learning approach, the AI is presented with many labeled medical images of normal anatomy. In addition, the AI is fed with multiple images of abnormalities that are found in medical images, such as cancer cells, heart conditions, breaks or tears. A computer is shown multiple examples of medical images that contain each abnormality so it can learn over time what each abnormal image looks like from multiple angles. Once trained, these systems of AI in medical imaging can perform single narrow recognition tasks.
IBM researchers estimated that medical images make up more than 90% of all existing medical data available today, and this number is only expected to grow. This overwhelming amount of image data necessitates assistance from technology in order to prevent medical professionals from being overwhelmed. AI is helping to fill this gap and is currently being developed and applied for various medical imaging needs. AI is helping with neurological scans of brain images. AI is also helping medical professionals determine the best imaging settings during capture to reduce radiation and increase the accuracy of images. It's also being applied post-image capture to help enhance images, as well as remove and reduce artifacts and abnormalities caused by the capture process and environment that would otherwise make an image hard to read.
AI in medical imaging is also improving early breast cancer diagnosis. Imperial College London in partnership with Google Health trained an AI on X-rays utilizing images from 29,000 women. After a period of training, the AI is now outperforming trained radiologists when reading mammograms and diagnosing breast cancer. The National Health Service in the U.K. currently has two radiologists look at and analyze each X-ray to confirm they come to the same conclusion. The results of the AI model showed that it was just as good as the current double-reading system of two doctors and better than a single doctor check. While promising, AI systems are currently still being used as an augmented intelligence tool and a second set of eyes -- at least in the U.S. as the Food and Drug Administration has not approved these AI systems for making medical diagnoses.
The risks and challenges of AI in medical imaging
While there are many positive use cases and scenarios where AI can play a vital role in the field of medical imaging, there are also potential risks, challenges and concerns. One obvious concern that many people have is a potential misdiagnosis by the AI. Even if only a few people are misdiagnosed, this could have serious -- potentially deadly -- outcomes. Humans, for the foreseeable future, will still be needed as a part of the process of reading medical images. At some point in time, we may reduce the number of doctors needed to read a scan, but that is a long way off.
Another area that needs to be addressed is trust. AI is still an emerging technology, and certain algorithms lack explainability. If an AI does misread an image, people want an explanation as to why the AI came to that conclusion, and currently, this isn't always possible. By working with doctors, medical professionals, administrators and even patients during the development process, this helps start to educate everyone on what AI can and can't do and will hopefully be the start of building trust. High success rates for AI in medical imaging will also work to build trust.
At the end of the day, the best way to keep the risks, challenges and concerns at bay is to keep humans in the loop. For medical imaging, AI is currently an augmented intelligence tool that is meant to work with doctors and help verify what they have already found or didn't find. When it comes to medical imaging, AI has already shown great potential. More work is still needed before it can be a generally essential tool, but it is bound to happen.