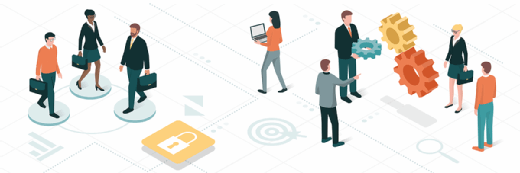
The future of data science and AI points to automatic tools
The relationship between data scientists and companies using AI is evolving rapidly, shifting from a focus on trained professionals to experienced employees with automated tools.
Emerging about a decade ago from roots in statistical modeling and data analysis, data scientists are employed to help companies adopt data-centric approaches to their organizations. Since data scientists have a comprehensive understanding data, they work well in moving organizations towards machine learning, deep learning and AI adoption because they generally have the same data-driven goals.
Data scientists help companies figure out how to extract useful information from a sea data to help analyze and optimize their organizations based off of the findings. Data scientists focus on analyzing data, asking data-centric questions and applying mathematics and statistics in order to find relevant results.
Data scientists have backgrounds in advanced math and statistics, advanced analytics and increasingly in machine learning and AI. For companies who are looking to run an AI project, having a data scientist on the team is very beneficial to get the most out of their data, customize algorithms and weigh in on data-centric decisions.
Challenges in hiring data scientists
Since the field of data science is still fairly new, many organizations are playing catch-up with finding and hiring the right skill sets for this position. There simply aren't enough people in the job pool who have the data science skill sets needed. Unfortunately, there are more companies looking to hire data scientists than there are data scientists to fill these positions, causing a talent crunch.
Many universities have only recently in the past few years a data science program, so collegiate-level talent is still nascent. Further, many people who are established in data science are working for very large organizations that can pay top dollar such as Google, Facebook, LinkedIn and major enterprise employers such as Capital One and American Express. There simply are not enough data scientists to go around, particularly for mid- and lower-level companies that can't afford the high salaries.
When it comes to hiring a data scientist, there are a few key skills companies will want to look for. The is that prospective data scientists must have backgrounds in advanced math and statistics, advanced analytics and perhaps machine learning and AI. These individuals need to extract value out a company's data, so if they don't know how to understand that data in the place, they won't be very successful.
Another skill set that's important -- and may not be something people initially look for -- is the ability to be a creative thinker. Creative problem solving is required to be a successful data scientist, and it is creativity that you want driving the thought process behind successful AI solutions. Innovation in data science can result in giving a company a competitive edge.
In addition to being creative, data scientists should have experience with various popular coding languages such as R or Python. While programming is not a core part this role, analytical-focused programming knowledge provides tools needed to run advanced analysis on data.
Do you really need a data scientist?
Just because a company can't find or afford a team data scientists doesn't mean it needs to its data science goals or lose sight advanced machine learning or AI opportunities. Depending on what a company is interested in pursuing with its AI strategy, it may or may not even need a team data scientists.
For companies with very large datasets and complex use cases or a large-scale approach, it is likely that a company will certainly need more than one data scientist in order to carry out the project in a reasonable amount time. However if a company is planning on pursuing many smaller efforts, it can be just as valuable to have only one or two data scientists per team to work alongside other members a team. Depending on the need, the data scientist might be able to work closely with developers in order to reach an end goal rather than requiring everyone on the team to have that specific skill set. Data scientists can also work alongside and train members the existing team to perform as citizen data scientists.
As the relevancy artificial intelligence continues to grow and the talent crunch around data scientists grows with it, many companies are wondering if they can go without one. It can be difficult to find a good data scientist, and their salaries are often steep. It is possible to move towards an AI future without having a data scientist on board, but it really depends on the projects you're looking to run.
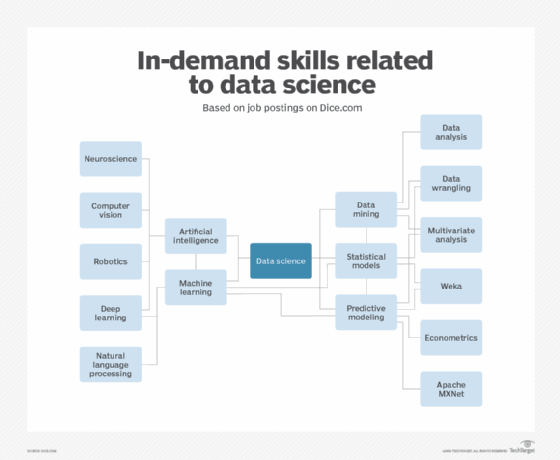
Advanced tooling for citizen data scientists
As the popularity of AI continues to grow, a number of companies are creating tools to help reduce dependence on data scientists. One such tool is autoML, offered by a number of vendors who are creating tools and dashboards that automate parts of the data science workflow. The goal of automated machine learning tools is to automate the processes of algorithm selection, hyper parameter tuning, iterative modeling, model assessment and even elements of data preparation to speed up the overall process, and take out some of the more complicated aspects for setup that have previously needed skilled data scientists. Once an organizations' data is run through autoML systems, it produces a machine learning model which can be used directly or analyzed by a worker. Usually, these post-autoML activities can be accomplished by employees with far less training than data scientists, or existing employees who have been trained in new skills.
Additionally, organizations can make use of machine learning models that have already been trained for the problem at hand. They can use these models directly, or extend them using transfer learning. This requires significantly less resources than would be otherwise required to build these models from scratch. Pre-trained models have already been trained on relevant data, and provide the required classification, regression, clustering or prediction as needed by the end user. Developers and line of business analysts with limited machine learning expertise can train high-quality models specific to their business needs. With an ever growing list of pre-trained models appearing, companies are able to use this for sentiment analysis, text and image classification without the need for large well-labeled datasets and data science resources required to train a complex model. Increasingly, vendors are offering models as a service, which can be used on public or private cloud infrastructure, allowing smaller companies access to large, complicated, and well-trained models without having their own large datasets. All of this reduces the need for data science roles within an organization.
As the talent gap for data scientists continues to widen, there is no doubt that we will see new tools -- created out of necessity -- that allow non-technical and business employees to run, test and analyze data. Business managers will begin to learn basic data science to help manage and move AI projects forward. Traditional data scientists will still be needed to run very complex analysis of data, but for the most part, basic analysis will move to citizen data scientist roles due to increasingly easy-to-use tools.