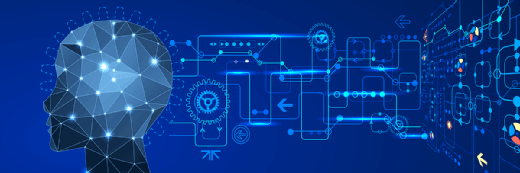
The AI accelerator chip can make AI accessible to all
Specialized AI chips released by companies like Amazon, Intel and Google tackle model training efficiently and generally make AI solutions more accessible.
Artificial intelligence products are technically complicated to train and run, but the development of specialized AI accelerator chips is making these more effective and efficient. Typically, the cost and time required to train and run machine learning models, as well as the need for a highly technical team, have limited some companies from applying AI to their specific needs.
The introduction of these AI accelerator chips to the market is broadening access to AI and has made running AI models much more efficient. This technology is capable of greater efficiency in model training and execution and these chips are finding their way into various industries around the globe. With this development, the industry is one step closer to bringing AI into the hands of the everyday consumer.
AI chips could make AI adoption more widespread
Within these specialized AI chips, custom microprocessors and math-optimized processing units are applied to handle AI tasks faster and use less power than traditional chips. Over the past decade, the use of GPUs has brought raw math calculating horsepower to the challenge of training large and complicated neural networks.
These GPUs boast the ability to speed up model training time from 50- to 100-times faster than a typical, commodity CPU. However, GPUs are power hungry and expensive. Companies are looking for additional approaches to expedite model training and inference without it eating into their wallets and power consumption.
An increasing number of specialized chips are being built to handle the specific needs of complex AI algorithms systems and analytics and bringing their power to various devices which mostly live on the edge of networks and do not always have a reliable connection to the internet. Condensing this kind of technology to specialized chips is allowing companies in various industries to effectively utilize the power of AI while avoiding expensive and power-hungry setups. So far, AI chips are being used in multiple ways throughout the automotive, healthcare, aerospace, defense and consumer electronics industries.
Currently, a variety of big players are working on AI accelerator chips. One of the more recent developments is Amazon's Inferentia, a machine learning inference chip that was recently announced. Intel has joined the market through one of its subsidiaries, Movidius. IBM has brought their own AI chip and Google's Tensor Processing Unit is in its third iteration, which is aimed to reduce its dependency on chipmakers like Nvidia.
In addition to the companies listed above, Alphabet, Google's parent company is working on specialized AI processors in several different sectors. Apple is also continuing to improve upon AI chip technology and ARM Holdings is producing chips that are finding increasing adoption for AI training and inferencing.
Benefits of specialized AI chips
Depending on what the user or company values, AI chips might be a viable alternative to traditional AI technology. The biggest benefit that comes with AI chips is focused around security. Traditional AI involves taking data and securely transporting it to the cloud for evaluation, which comes with risks or restrictions of data access and movement.
Further, it costs valuable time and, in some circumstances, cuts off users from the system if there is a problem with the internet. AI chips, on the other hand, are localized. This means that the data stays on the device, which drastically lowers the likelihood that it will be compromised, since most devices can be remotely erased when stolen. Additionally, it means that the processes can continue offline which can be incredibly important for certain applications or if the device is expected to work without access to the internet.
Other benefits surrounding the use of AI chips include low latency, low power consumption and easy business integration. Unlike AI systems powered by CPUs and many popular GPUs, which are known for consuming lots of energy, AI chips make it easy to get computational ability with much lower power consumption. The speed of the AI system is dramatically improved, making it possible to do the same amount of work with less power and higher efficiency. This is an obvious benefit, particularly for systems where immediate results are incredibly important.
Moving AI forward
With more and more AI being pushed to edge devices, it's no surprise that chipmakers are seeing the potential for this market. In fact, the AI chip industry -- edge and data center combined -- is expected to grow from about $6 billion in 2018 to more than $90 billion in 2025 according to a recent Research and Markets report.
As is the case with any new rendition of technology, AI accelerator chips have their own list of respective benefits and drawbacks that one might expect. Some are concerned that the proliferation of high-powered, low-price chips will make AI pervasive in ways that we might not expect.
As bigger names begin to enter the market, we can only speculate about the capacity of these chips as they continue to improve on efficiency, power and size. As organizations continue to improve upon existing AI chips, these specialized processors will likely shrink down in size, require even less power and operate more efficiently. From that, additional possibilities and use cases will only continue to grow.