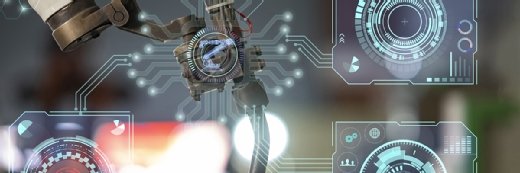
Getty Images/
Survey: Enterprise generative AI adoption ramped up in 2024
Nearly a third of organizations are now using generative AI in production. But data quality, the AI skills gap and an evolving regulatory landscape continue to present hurdles.
Generative AI is no longer just an experiment. For many organizations, it's part of everyday operations.
2024 saw notable progress in organizations' generative AI adoption, according to Enterprise Strategy Group's recent survey of over 800 IT and business decision-makers across industries and business sizes. Especially in areas like software development and IT operations, organizations are finding that generative AI tools can increase productivity, improve efficiency and automate workflows.
This year's report builds on ESG's previous research into the generative AI market. Compared with the 2023 survey, the 2024 data shows several new trends: More organizations are moving generative AI into production, early generative AI use cases are emerging, and there's a clear emphasis on productivity and efficiency -- but additional challenges are surfacing, too.
Organizations move beyond proofs of concept
Generative AI adoption has come far in the past year. In 2023, most organizations were just getting started with the technology. Only 18% had deployed generative AI in production, while 24% were engaged in pilot projects.
This year, almost all respondents (92%) said generative AI use in their organization had increased over the previous 12 months. Nearly a third of businesses are now running generative AI in production, and the share with mature generative AI initiatives has doubled, from 4% to 8%.
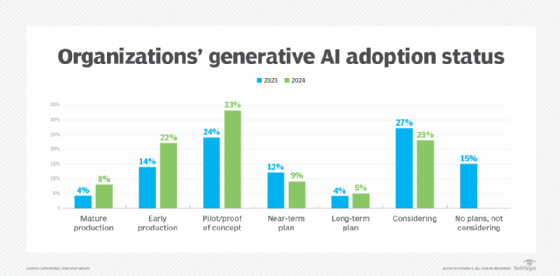
Of course, generative AI isn't as universal as the hype sometimes might imply. If 30% of businesses are running generative AI in production, that means 70% aren't there yet -- and "production" can mean different things to different organizations.
Still, the acceleration in generative AI deployment is noteworthy, given the sometimes-glacial pace of enterprise IT innovation. For many businesses, the question seems to be not whether to integrate generative AI, but how quickly they can roll it out.
Budget allocations reflect this shift. In 2023, less than a year into the generative AI boom, organizations were cautious in their spending. Only around half had dedicated AI budgets and those that did kept their AI investments fairly modest.
However, the 2024 survey shows an uptick in enterprise AI spending, likely reflecting confidence in AI initiatives' ROI and fears about falling behind. Just 1% of respondents expected their generative AI budgets to decrease over the next 12 months, while almost 70% anticipated an increase.
Major use cases for generative AI begin to emerge
As more businesses adopt generative AI, they're developing clearer understandings of where it's best applied. In some areas -- especially technical roles -- its use has grown, while in others, such as sales and marketing, it's actually declined since 2023.
Software development and IT operations are among the most popular generative AI use cases, consistent with broader market trends. Developers are using the technology to write code, troubleshoot problems and automate testing. A separate Enterprise Strategy Group survey of application developers found that 63% used generative AI in production, citing faster code creation and improved customer support as top benefits.
IT ops teams -- often burdened with manual, reactive work -- have long been skeptical of traditional AIOps tools, which frequently have overpromised and underdelivered. But these teams have largely given generative AI tools a more positive reception. In a separate survey of IT ops professionals, Enterprise Strategy Group found overwhelmingly positive experiences with generative AI. Top use cases included natural language chatbots, automated ticket triaging and incident response.
Due to the nature of their work, developers and IT teams usually are more familiar with and open to emerging technologies. They're more likely to have the skills and technical foundation necessary to experiment with generative AI tools -- and, importantly, to recognize and troubleshoot their limitations. These roles often also face more pressure from leadership to stay ahead of the curve in technology, compared with organizational peers whose roles don't require tech expertise.
Generative AI also tends to yield efficiency gains more quickly in technical workflows, where structured data, such as enterprise codebases, IT tickets and performance logs, is more readily available for fine-tuning generative AI models. Less technical departments often deal with messier data and more subjective, harder-to-define goals.
This could explain why sales and marketing have proven more challenging to automate than initially expected. Feeding a model a consistently formatted history of performance logs to teach it how to identify anomalies might be relatively straightforward. But building a model for a task such as writing marketing materials in a brand's voice is much more subjective. It's also more likely to involve onerous data-related tasks, such as collecting historical marketing collaterals and preparing them in a format that a generative AI model can use as training data.
Businesses hope for increased productivity, but challenges remain
Changes technical departments have seen explain why many organizations are drawn to generative AI. In 2024, increased productivity, improved operational efficiency, and process improvements or automation were the most commonly reported generative AI benefits.
But these advantages are accompanied by a growing awareness of the technology's challenges and limitations. Organizations' top three concerns -- employee expertise or skill, data quality, and ethical or legal considerations -- remained the same as last year, but higher percentages of respondents flagged these issues in 2024.
Lack of expertise remains the biggest challenge, with 41% of businesses struggling to find employees to support their generative AI initiatives. There's a longstanding skills gap in AI and machine learning, and generative AI's popularity and rapid evolution have only made it more difficult to hire AI experts. Competition for the limited existing talent pool has intensified, making it particularly difficult for smaller or less tech-centric businesses to attract AI professionals.
Data quality is another enduring obstacle to generative AI success, and it tends to become more important as generative AI initiatives mature. A model that affects real end users and business outcomes needs to be trained on accurate, reliable and representative data. Although many organizations might technically have the data needed for training or fine-tuning, there's a good chance it's not centralized, well organized or stored in a way that a model can easily access.
New challenges have also emerged in 2024 as generative AI initiatives progress. This year, higher percentages of respondents reported encountering issues around integration with legacy systems and regulatory compliance.
Last year, many organizations were in the early stages, just starting to plan their generative AI initiatives or working on proof-of-concept projects. These projects are often small, standalone applications that can run in an isolated environment with a narrowly tailored data set and limited interaction with other parts of the organization's IT ecosystem.
Yet, as organizations start deploying and scaling generative AI, they encounter older systems that weren't designed for modern AI applications. Frequently, these legacy systems are built on outdated infrastructure or in older programming languages, which can make it difficult or impossible to work with new AI tools. Around 65% of respondents said they needed to change or modernize their IT infrastructure to support generative AI applications.
And as generative AI is deployed in real-world contexts where it can affect end users, regulatory compliance concerns are also on the rise. In 2024, organizations have been confronted with new, AI-specific statutes, such as the EU AI Act. At the same time, they need to interpret existing regulations, such as American copyright law and the EU's GDPR, to understand how these statutes could apply to generative AI systems.
Roughly three-quarters of respondents expressed concerns about generative AI's data privacy and security risks. In the coming year, organizations -- especially those in highly regulated sectors, such as finance and healthcare -- will need to not only comply with existing laws but also think ahead, anticipating possible future legal developments.
Lev Craig covers AI and machine learning as the site editor for TechTarget Editorial's Enterprise AI site. Craig graduated from Harvard University with a bachelor's degree in English and has previously written about enterprise IT, software development and cybersecurity.