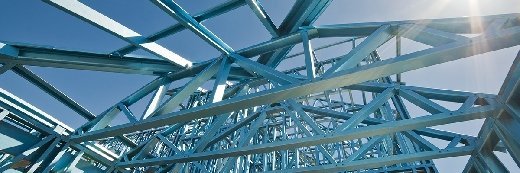
Lev - Fotolia
Steel producer reduces costs using AI in manufacturing
The largest long steel producer in Latin America used data and machine learning predictions to save money, while maintaining the same level of production quality.
Gerdau, the largest producer of long steel in Latin America, had a lot of data coming in from its numerous manufacturing machines but struggled to use that data.
The company, headquartered in Brazil, wanted to lower production costs, while maintaining the same quality steel. While providing some cost-saving predictions, the traditional tools it used couldn't make real-time adjustments and had trouble evaluating multiple nonlinear relationships between the data.
The predictions the traditional tools made were low-accuracy, and Gerdau wanted to improve accuracy by adding AI in manufacturing to the process.
The steel producer turned to Fero Labs. The 2016 startup sells a platform that uses machine learning to optimize factory predictions.
It's not easy
Using machine learning to reduce production costs can be difficult.
"The initial challenges are around working out what to measure, working out how to measure it, working out what it means and then working out what to do about it," explained Forrester Research analyst Paul Miller.
Gerdau worked on its machine learning models in Fero Labs' platform for weeks, throwing all kinds of data into it and seeing what results the models produced, said Jena Kreuzer, a process engineer at Gerdau North America.
That data included the chemical and physical properties of the production materials and the final steel product. With steel, for example, data included what the thickness of the final product will be and what the temperatures are during various parts of the manufacturing process.
The right data
Speaking broadly, Miller said, it is difficult to obtain, and then use, the correct data.
While new machines arrive from their manufacturers already fitted with sensors that can capture important data, most machines on the factory floor are older, Miller said.
"Many may have been in use for a decade or more, and they either have no sensors at all or they have proprietary sensors that send commercially sensitive data in proprietary formats, which can be hard to decode," he said.
Some companies sell after-market sensors that customers can fit onto their older machines if they understand what they want to measure, Miller continued.
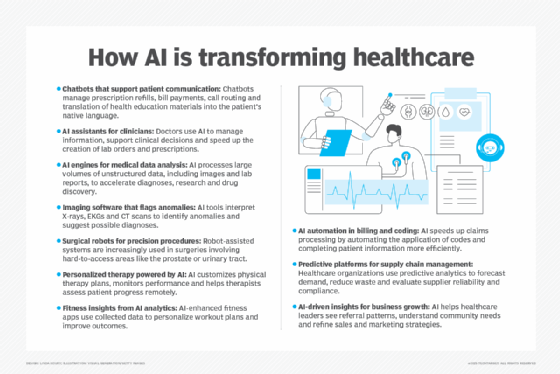
Even with a steady stream of data coming in, factory users need an understanding of what is important in that data and what the signals mean, he said.
For example, users can't shut down a machine because it gets a bit warm -- machines often warm slightly, Miller said. They need to determine how warm is too warm.
Here, it's important to involve the factory floor workers -- the people who have been working with these machines for years and know what the important signals are, Miller said.
"This isn't just an exercise in data science, which can be done in isolation from the reality of the factory floor," he said.
"Usually, when it's approached as just a data science exercise, you get a tool that is pretty good at triggering alarms and alerts too often, and the engineers on the shop floor quickly learn to ignore it and to go back to doing what they did in the past," he continued. "That's a missed opportunity."
Before using Fero Labs, Gerdau, which already had sensors on its machines, faced problems with the clarity of the machine data it was collecting and sending to numerous other software programs it uses.
"The data was just really bad or missing half the time," Kreuzer said.
Optimizing work
The Fero platform identified some of the bad or missing data coming in, alerting Gerdau quality departments to data they had to drill down into and clean.
After cleaning up its data, Gerdau focused on getting accurate predictions about the mechanical properties of the steel it made based on the data coming in. The company then focused on creating live-prediction dashboards that could show operators real-time results as they work the machines.
Gerdau then focused on using Fero Labs to optimize its chemical formulas to use the least amount of raw materials possible to make steel with the mechanical properties it needed. The company experimented with those predictions, creating models that could detail how they could move leftover materials to different products, to further cut down on waste.
"So, there's been a lot of development as we go," Kreuzer said.
Gerdau is now trying to make its predictions more accurate. Gerdau has set up the Fero platform to send a monthly report detailing the predictions the platform has made and if or how results differed from those predictions.
Gerdau is working on optimizing its predictions and results based on those reports and creating new reports, which include one that details how much money the company could save if the predictions worked correctly.
"We keep having new ideas of what we could do with these predictions," Kreuzer said.
It's easy to set up new models with Fero, she said.
"If we have an idea and we want to see if they can predict it, we can just set those models up," she said. "There's always room to build new cases as we go along."
Gerdau has reduced production costs, so far, by about 9% since using Fero Labs, according to Kreuzer.