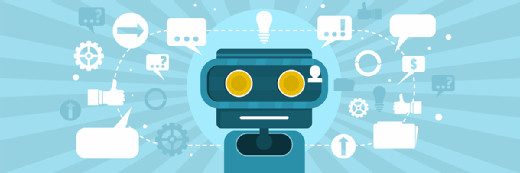
NLP vs. NLU and the growing ability of machines to understand
NLP and NLU technologies are becoming more prominent in the enterprise. The former simply parses words, but the latter aims at a deeper level of understanding.
Humans want to speak to machines the same way they speak to each other -- in natural language, not the language of machines. The field of natural language processing in computing emerged to provide a technology approach by which machines can interpret natural language data. In other words, NLP lets people and machines talk to each other naturally in human language and syntax. NLP-enabled systems are intended to understand what the human said, process the data, act if needed and respond back in language the human will understand.
However, NLP, which has been in development for decades, is still limited in terms of what the computer can actually understand. Adding machine learning and other AI technologies to NLP leads to natural language understanding (NLU), which can enhance a machine's ability to understand what humans say. As it stands, NLU is considered to be a subset of NLP, focusing primarily on getting machines to understand the meaning behind text information.
However, in the future, as AI systems move from machine learning to machine reasoning, NLU will itself be much broader as it starts to encapsulate developing areas of common sense and machine reasoning, areas where AI currently struggles. In this context, when we talk about NLP vs. NLU, we're referring both to the literal interpretation of what humans mean by what they write or say and also the more general understanding of their intent and understanding.
When people hear about natural language applications, many first think of chatbots. AI and machine learning have helped advance areas of NLP and natural language generation (NLG) to unlock the power of unstructured information and content in ways not possible before. In particular, NLP has seen its widest application powering voice assistants we interact with, such as Amazon Alexa, Google Assistant or Apple's Siri. However, the use of NLP in the enterprise is much broader than the conversational assistant, and the applications for NLU and NLG go well beyond chatbots.
NLU enhances sentiment analysis
Unlike structured data, which conforms to a well-formatted schema and is stored in an orderly fashion, such as a database, unstructured data can come in any arbitrary format and be stored in any location where computers interact with that information. For many organizations, the majority of their data is unstructured content, such as email, online reviews, videos and other content, that doesn't fit neatly into databases and spreadsheets. Many firms estimate that at least 80% of their content is in unstructured forms, and some firms, especially social media and content-driven organizations, have over 90% of their total content in unstructured forms.
In the past, this data either needed to be processed manually or was simply ignored because it was too labor-intensive and time-consuming to go through. Cognitive technologies taking advantage of NLP are now enabling analysis and understanding of unstructured text data in ways not possible before with traditional big data approaches to information. AI-enabled NLU gives systems the ability to make sense of this information that would otherwise require humans to process and understand.
Stephen Lernout of software company Nalantis explains
natural language understanding
For customer service departments, sentiment analysis is a valuable tool used to monitor opinions, emotions and interactions. Sentiment analysis is the process of identifying and categorizing opinions expressed in text, especially in order to determine whether the writer's attitude is positive, negative or neutral. Sentiment analysis enables companies to analyze customer feedback to discover trending topics, identify top complaints and track critical trends over time.
This deep functionality is one of the main differences between NLP vs. NLU. By using NLU technology, organizations can analyze a wide range of unstructured content sources, such as reviews, survey responses and social media posts, as well as monitor and review conversations to find patterns in these customer interactions. AI technologies enable companies to track feedback far faster than they could with humans monitoring the systems and extract information in multiple languages without large amounts of work and training.
NLP still drives value inside the organization
In addition to monitoring content that originates outside the walls of the enterprise, organizations are seeing value in understanding internal data as well, and here, more traditional NLP still has value. Organizations are using NLP technology to enhance the value from internal document and data sharing. The use of NLP technology gives individuals and departments the ability to have tailored text, generated by the system using NLG approaches.
For example, executives and senior management might want summary information in the form of a daily report, but the billing department may be interested in deeper information on a more focused area. Companies are also using NLP technology to improve internal support operations, providing help with internal routing of tickets or support communication. Using NLP, every inbound message and request can be reviewed and routed to the correct parties quickly with fewer errors.
NLP powers e-commerce
E-commerce applications, as well as search engines, such as Google and Microsoft Bing, are using NLP to understand their users. These companies have also seen benefits of NLP helping with descriptions and search features. Major internet companies are training their systems to understand the context of a word in a sentence or employ users' previous searches to help them optimize future searches and provide more relevant results to that individual.
For example, Amazon has been using AI-enabled NLP systems for years to suggest product recommendations based on what users have already said they liked. Google has used NLP to automatically create captions after recognizing the content in images, making it easy for computers to summarize an image with just a few words.
There's no doubt that AI and machine learning technologies are changing the ways that companies deal with and approach their vast amounts of unstructured data. Companies are applying their advanced technology in this area to bring more visibility, understanding and analytical power over what has often been called the dark matter of the enterprise. The market for unstructured text analysis is increasingly attracting offerings from major platform providers, as well as startups. With unstructured content only growing for most organizations, it's important to have ways to continue to capture, analyze and make sense of this valuable data, and understanding the differences between NLP vs. NLU is a crucial first step.