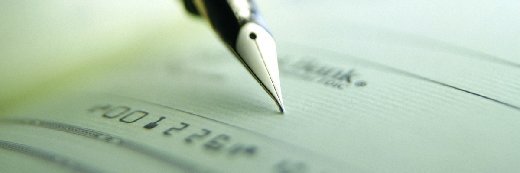
Art Allianz - Fotolia
Inventory optimization machine learning tool sharpens pricing
Retailers are chasing data-driven process automation in hopes of boosting sales and streamlining margins. But how do you decide which processes to automate or vendor to implement?
In a sea of vendors who promise to optimize e-commerce and boost sales, wading through the advertisements to find the right match for the right process can be incredibly difficult.
German-based international clothing retailer Orsay recently set out on a journey toward inventory optimization through machine learning tools. They chose to automate their timing and pricing of markdowns in order to maximize profit based on factors specific to consumers and their country.
In this Q&A, Katrin Starke, head of business development at Orsay, details the company's journey to using inventory optimization machine learning tools from project conception, decision-making, to implementation of a tool called Luminate Clearance Price (LCP), sold by Arizona-based technology vendor JDA Software. Orsay has been running the software for nearly a year.
Editor's note: The following has been edited for clarity and brevity.
How did you decide which process to automate?
Katrin Starke: We were looking to improve total margin gains for the company and realized optimized stock management could help us do this. We face a lot of challenges and insufficiencies along the product lifecycle due to manual interactions and [managing] volumes of data simultaneously.
We realized the gap could be closed through a scientific approach. A machine is better at managing complex business problems because it can keep a helicopter view and concentrate on what matters while gathering, sorting and managing real-time data.
Markdown optimization with the help of LCP was selected because of its forecast accuracy. The further we move along the [purchasing] cycle, the higher the forecast accuracy. For markdowns, it is almost 95% accurate, and that's why we wanted to start with markdown optimization.
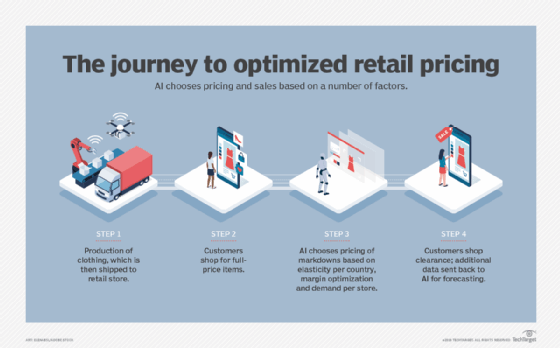
How are you using LCP as an inventory optimization machine learning tool?
Starke: The implementation of LCP supports Orsay in defining the right price at the right time to effectively manage inventory issues in a margin-optimized way. With the help of LCP and the applied AI algorithms from Blue Yonder [a JDA company], we can forecast demand over time and deliver data-driven, continuously optimized prices at the finest levels of granularity.
This data-driven approach helps us understand how we are managing our business and supports how we make decisions. For example, we can use LCP to understand which country is more price sensitive than other ones and can allocate inventory properly.
How has using LCP impacted your online and brick-and-mortar stores?
Starke: LCP allowed us to better define the price elasticity per country. This helps us send and clear the merchandise in the country where a demand exists. As a result, some stores receive fewer markdowns than others due to the demand forecast calculated by LCP. It helps free up space for fresh merchandise. The online store in each country is following the same strategy as brick-and-mortar, so there is no negative impact for online.
What are some benefits you've seen from implementation?
Starke: The value we've been able to derive out of our data. We understand our business better and concentrate on real issues, not only on markdowns. It also helped us realize that the targets we put in place years ago are not valid anymore. In the past, we mainly concentrated on stock optimization -- somehow getting rid of the merchandise. Now, we're concentrated on optimizing our margin.
What have been some pain points or learning curves in implementing an inventory optimization machine learning platform?
Starke: Besides having to learn how to use the available data and understand it, we faced challenges with employee buy-in. Not everyone trusts a machine and feels comfortable letting go of control over the pricing decision. We had to invest a lot of time to explain to people the benefits of automating the enterprise.
Another pain point at the beginning was the data availability. We had to digitize our business -- we originally did not have all of data points available in our data warehouse. We had to create these from scratch. It was a pain point at the beginning, but in the end, we were able to shift from business perspective to a data perspective.
Are you working with AI other than LCP systems?
Starke: Not today, but we would like to have end-to-end AI coverage in the future. We would eventually like the whole product lifecycle to be data-driven, from allocation to markdown. The base of each activity is demand forecast. This forecast can be done precisely only with technology support.