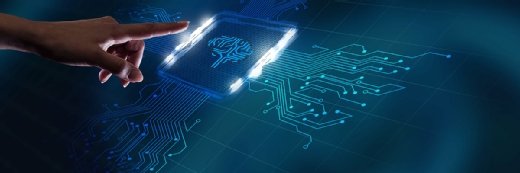
putilov_denis - stock.adobe.com
Insights on generative AI for automation vs. augmentation
At the MIT Sloan CIO Symposium, industry leaders shared experiences with generative AI's benefits and challenges, highlighting the technology's ability to assist human workers.
From boosting productivity in call centers to aiding junior developers, generative AI is reshaping today's workplaces, but not without raising critical ethical and practical concerns.
At the MIT Sloan CIO Symposium this week, speakers raised many potential benefits that incorporating generative AI could offer businesses. But they also emphasized that human oversight and critical thinking are crucial to effectively using the technology.
"The core concept of human judgment and context and knowledge doesn't go away," said Sanjay Srivastava, chief digital officer at professional services firm Genpact.
Despite the excitement currently surrounding generative AI, experts emphasize that its deployment is fraught with challenges. While the technology can enhance productivity and support knowledge workers, it also raises complex ethical questions and practical challenges for organizations.
"It's a unique moment for CIOs in general, and we need to use our voice around governance and risk management and quality and ethics, in addition to just being technologists," said Akira Bell, senior vice president and CIO at research and data analytics consultancy Mathematica.
Generative AI as a collaborator, rather than a replacement
Despite recent advancements and the resulting hype, today's generative AI models typically aren't fully automating jobs. The technology still has notable limitations, and many organizations don't currently have the data, compute resources or AI talent to deploy it effectively.
But that doesn't mean there's no role for generative AI in enterprise settings. In the panel "The Human-AI Collaboration: Integrating Human Judgment with Advanced Technologies," participants emphasized the distinction between fully automating tasks and augmenting human work with AI.
Here, full automation would mean using AI to completely take over certain tasks or even entire jobs, eliminating the need for human intervention. In contrast, augmentation involves using AI to enhance and support human capabilities -- think using an LLM to summarize sales history and generate an outline for a person writing a report on that data rather than composing the entire document start to finish.
For many professions, particularly knowledge workers whose responsibilities involve advanced skills and complex decision-making, generative AI is better suited as a collaborative tool. Positioning generative AI as an assistant rather than a replacement can also mitigate risks stemming from the underlying architecture of LLMs.
Unlike traditional deterministic systems, where actions have predictable outcomes, generative AI models produce probabilistic outputs, meaning that their responses have a degree of uncertainty. While often beneficial for creative tasks, this unpredictability means that decisions based on these models often can't be fully automated. A human in the loop is necessary to make judgment calls, particularly for high-stakes decisions.
"I think the issue is, what are the use cases and circumstances and scenarios where you defer to the automation versus [where] you have decision rights?" said Michael Schrage, a fellow with MIT Sloan's Initiative on the Digital Economy. "Who has the right to make certain kinds of decisions? Who is obligated to make certain kinds of decisions?"
Schrage also noted that the line between augmentation and automation might be blurry in some settings. "It's becoming harder to tell the difference between automation and augmentation," he said. If a knowledge worker uses an LLM to brainstorm, for example, to what extent is that augmenting the worker's preexisting thought processes versus automating or removing them?
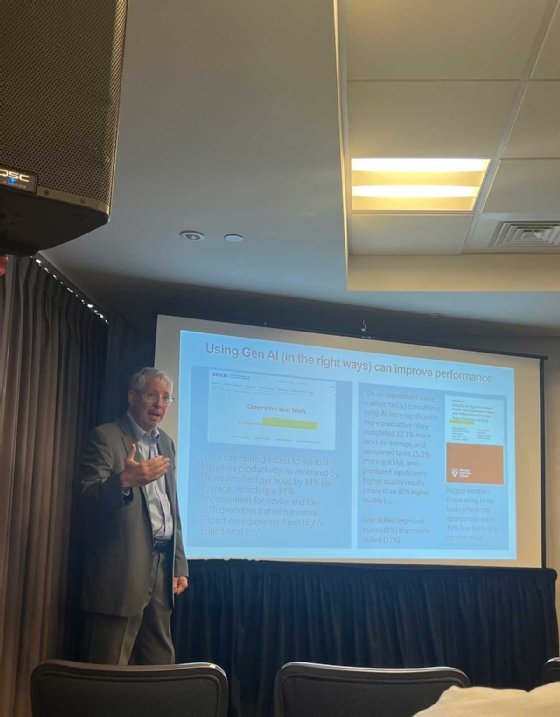
Consequently, it's important to avoid mythologizing generative AI and instead understand it as just one component of broader IT and digital transformation strategy. "Gen AI is another tool to aid in those transformations," said George Westerman, a senior lecturer at MIT Sloan. "It's a good tool; it's moving really quickly. But we want to think about the transformation part, not the technology."
And given the current levels of what Bell terms "AI fever," it's also worth keeping in mind that generative AI isn't the only form of AI, nor is it the appropriate choice for every task. She mentioned several examples of non-generative AI that have already shown promise in enterprises -- including robotic process automation and forms of natural language processing besides LLMs -- and encouraged companies to choose "the right tool for the right problem."
Westerman echoed this sentiment. "We've got a lot of hammers looking for nails," he said in his presentation "How Generative AI Will Transform Knowledge Work." He said it's important to prioritize solutions to real business problems over simply seeking ways to apply trendy technologies like generative AI.
"There are certain kinds of problems where generative AI is the wrong answer, so you want to be careful about using it in the right places," he said in an interview. "If you need to be repeatable, explainable, [and] 100% accurate, consider whether there might be a better alternative."
Generative AI's diverse industry applications
Westerman described three ways he sees generative AI aiding knowledge workers: reducing cognitive load, boosting existing capabilities, and serving as a coach or brainstorming partner.
But despite these broad benefits, generative AI won't be equally relevant to all job roles and workflows. Some sectors and tasks make more sense as early adopters than others. "Different industries are approaching it very differently," Bell said.
As the technology matures, this has led to a shift towards what Srivastava termed vertical AI: smaller models optimized for specific applications and industry sectors rather than generic LLMs like the public versions of ChatGPT and Claude.
Multiple speakers mentioned customer service and call centers as areas particularly ripe for implementing generative AI. In his presentation, Westerman referenced Klarna's OpenAI-powered customer support assistant, which the company says does an amount of work equivalent to 700 full-time agents and had over 2 million conversations in its first month.
But there's also a potential role for generative AI in customer service as a collaborative tool rather than a replacement for human work. In a study published last November, implementing a generative AI-based conversational assistant increased customer support agents' productivity by an average of 14%, with an even greater improvement of 34% among novice and low-skilled workers.
Bell described a similar experience with AI elevating the productivity of less experienced employees in coding -- another field that several presenters highlighted as an early area of generative AI adoption. Incorporating AI in software development, she said, has helped junior developers contribute more meaningful work earlier in their careers. This helps them provide value to the organization more quickly while also improving job satisfaction.
"That's one of the use cases where we are both accelerating the workflow of development products but also helping with talent development and retention as a byproduct," she said.
For more experienced professionals with a strong command of a particular discipline, generative AI tools can help them effectively communicate their knowledge. At Mathematica, for example, Bell said that generative AI helps specialized knowledge workers such as economists, social scientists and data scientists translate their findings for nonexpert audiences, such as policymakers, teachers and researchers in other fields.
Addressing AI's practical, ethical and risk management challenges
Generative AI also entails significant practical, ethical and risk management concerns, including data governance, privacy, security, bias mitigation and reputational risk. Organizations won't fully reap the technology's benefits if they don't adequately address these issues.
"[Generative AI] is incredibly easy to pilot -- scarily easy to pilot -- but it's incredibly hard to get into production," Srivastava said. He highlighted the challenge of wrangling enterprise data, which tends to be messy and difficult to mine.
"When we run pilots, it's super easy to take a contained use case with a few known outcomes, run it across a very clean set of data and be able to show amazing results," Srivastava said. "When you take the same thing and put it in the real-world environment … many of us run into some pretty steep problems."
On the privacy side, sending internal data in conversations with a public generative AI chatbot exposes that data to third parties. For example, OpenAI by default uses conversations with ChatGPT as data for model retraining and updates. End users, especially nontechnical ones, might not be aware of this and thus inadvertently expose sensitive company information, creating security and compliance concerns.
"The good thing about unstructured data so far is that it was very secure in that it was obscure," Srivastava said. "All of a sudden, with all these [AI] copilots, the email I wrote to you three weeks ago, the PowerPoint I shared with my colleague, the Word document I wrote last night -- all of that can become discoverable. And as it does, the data in that can go to places I don't want it to go."
Generative AI also raises new cybersecurity considerations, one of the most significant being more effective phishing attempts. Due to the availability of LLMs that can produce high-quality, human-sounding text on demand, phishing attacks are becoming increasingly targeted and harder to detect.
"If you think about your typical really high-powered hacker as being a cat burglar to get into your systems, and you think about the phishing attempts as being zombies, just throwing thousands of them against the gates hoping one will get through," Westerman said. "Now [with LLMs], those zombies are going to be as smart as cat burglars."
These risk management concerns are especially significant given generative AI's unusually widespread availability to end users. Tech leaders have always encountered challenges around new technologies, such as security vulnerabilities, shadow IT and output quality management.
But, as Bell and Westerman both pointed out, the broad availability and consumer recognition of generative AI means that CIOs are having to deal with these issues much more quickly and at a larger scale than they did with previous technology waves, such as cloud migration.
"Whereas other forms of emerging technology have come to the market and largely just been at the hands of us tech folks, generative AI is not that at all," Bell said. "It's released to the masses already."
These issues go beyond managing privacy and security risk to encompass more complex ethical questions. Generative AI, such as all AI systems, is susceptible to algorithmic bias. If a model's training data reflects existing inequities related to factors such as race or gender, the AI's outputs can perpetuate and even amplify these biases. For example, a generative AI model trained on a data set that underrepresents certain demographics is likely to produce outputs that don't adequately reflect those groups.
"We cannot take our eye off the ball with the issues that may be present and inherent in the data that we look at -- how it may reflect biases in different systems, how certain populations may not be present in certain situations and may be overrepresented in others," Bell said.
Lev Craig covers AI and machine learning as the site editor for TechTarget Editorial's Enterprise AI site. Craig graduated from Harvard University with a bachelor's degree in English and has previously written about enterprise IT, software development and cybersecurity.
Olivia Wisbey contributed reporting to this story.