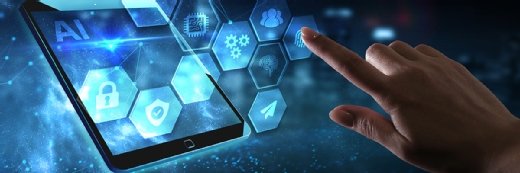
putilov_denis - stock.adobe.com
How different industries benefit from edge AI
From manufacturing to energy and healthcare, edge AI is promising to various industries. It brings data processing and analysis closer to data sources.
AI is known for eating up computing power, requiring massive data centers. But its impressive capabilities can exist on smaller and more portable devices when appropriate.
Edge AI is the practice of placing AI systems on decentralized hardware devices that operate closer to sources of data. This approach can reduce latency, improve privacy, cut down on data transfers from one device to another and improve the performance of local AI applications. However, it may also require more computing power than other common cloud computing workloads do.
Edge AI offers performance benefits in keeping computation close to a source of data, ensures security when privacy protection is a priority and provides logistical advantages where data is collected in difficult-to-reach locations. Use cases for edge AI that business leaders should know appear across various vertical markets. The main examples to explore are manufacturing and production, healthcare, energy, transportation and retail.
Edge AI vs. edge computing
Edge AI is about putting smart computing brainpower directly onto devices, such as smartphones, smart fridges and other IoT appliances. Devices make decisions without having to wait for information sent over the internet. Edge computing is about moving compute work close to where data is collected. In addition to AI-enabled decision-making, edge computing also covers tasks like data storage and simple processing.
"Edge AI is beginning to revolutionize the way that many industries collect and utilize data that is produced by their products and their operations," said Anand Rao, global AI lead at professional services firm PwC.
Edge computing runs many powerful systems, such as those for in-patient monitoring and predictive maintenance. Edge AI enhances the performance of these kinds of tasks. For example, it can use computer vision to detect important events, such as the slip and fall of a patient, from a local device and then send an alert to medical staff. Other examples of additional capabilities for edge devices include natural language processing and predictive analytics.
"Edge AI and edge computing are changing how we handle data and extract value out of data, but they do so in different ways," said Bharath Thota, partner in the advanced analytics practice of global strategy and management consulting firm Kearney.
Benefits of edge AI for businesses
Benefits for businesses include reduced costs associated with network load in instances of high data volumes, privacy protection for sensitive applications, and improved inference performance and accuracy. Edge AI also improves efficiency and reduces costs by pushing complex AI computations to local devices. This reduces reliance on large, centralized computer systems and servers for AI inferencing workloads. In cases where applications need to perform real-time analytics on data, the proximity to the data source reduces latency.
Edge AI can also enhance data security. It localizes the data collection process, performs predictions at the source of the data and restricts external network traffic to only the AI model's outputs, thereby protecting potentially sensitive data inputs. Finally, it's a reliable solution for devices and operations in remote locations with inconsistent network connections.
"Edge AI enables a variety of new use cases by reducing latency or even the need for an internet connection," said Lucas Lau, senior director at global consulting firm Protiviti. Apps run more quickly in these scenarios than they could without the edge computing setup.
Industry-specific use cases for edge AI
Industry use cases include the following:
- Manufacturing and production. Edge AI improves predictive maintenance on a fleet or set of production equipment. AI models predict when a piece of equipment is most likely to experience a failure. Locally running algorithms analyze factors like vibration, heat and acoustic data from machines. They do so at high resolutions and in real time. Insights gleaned from this analysis are the output.
Large-scale data collection efforts at manufacturing plants and field operations are improved by data cleansing and anomaly detection. Performing these tasks locally can reduce data overhead and improve response times. - Healthcare. Edge AI helps monitor patients in real time, improving care and safety. Wearable devices on the patient collect health data. When AI-based analysis of this information occurs locally, it reduces bandwidth consumption and enhances data privacy as compared to transmitting the data to be processed centrally.
- Energy. Edge AI can keep a real-time watch on equipment. This can help predict when energy equipment, like power plants and wind farms, might need fixing, just like in the manufacturing use case. In addition, it can improve tools that study energy use data to identify busy times, predict usage patterns and improve how plants distribute energy.
- Transportation. Edge AI processes data from sensors attached to vehicles in real time, enabling a self-driving vehicle to understand the surroundings, make decisions and navigate quickly. It can also provide warnings when a car is leaving its lane and enhance driver assistance systems.
- Retail. Edge AI improves security event detection by processing video streams that support loss prevention.