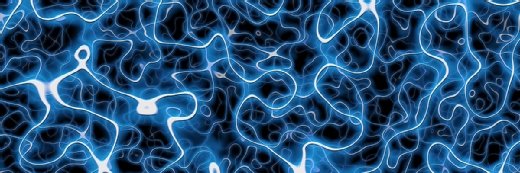
blobbotronic - stock.adobe.com
Here's how one lawyer advises removing bias from AI
Avoiding bias in AI applications is one of the central challenges in using the technology. Here's some advice on deploying AI technologies in a way that is fair.
Businesses, particularly in the financial sector, are applying AI techniques to rich datasets to develop powerful tools that engage and understand their customers, deliver better products and increase access to a broad range of services. It is difficult to remain competitive in financial services without applying AI tools.
But the pitfalls can be existential. Enterprises that don't make removing bias in AI applications a central component of their technology initiatives may suffer severe reputational harm and find themselves in the crosshairs of regulators. Building AI applications that avoid biased recommendations has never been more important.
What is bias in AI?
Technology is ethically neutral, but AI systems reflect the biases of the people who create them. These biases can be difficult for an organization to recognize ahead of implementation and the potential negative results could include loss of profit, reputational harm and exposure to myriad consumer and regulatory liability.
In financial services, bias in AI tools can cause an organization to make errors in asset valuations that cause missed investment opportunities or deny credit based on an applicant's race or gender, potentially violating anti-discrimination laws.
While it may not be possible to completely remove bias from AI applications, understanding how it arises and having a robust governance infrastructure with thoughtful policies, procedures and controls can mitigate its effects. Avoiding biases in these projects requires effective oversight and infrastructure, with technology deployment informed by balanced and diverse datasets and sophisticated governance programs. Teams need to know how to properly apply algorithms and consistently test outcomes for potential anomalies.
How can biased AI damage a business?
Even if stakeholders are aware of the potential for algorithmic blunders and are conscious of the importance of removing bias from AI tools, a well-intentioned team may overlook issues in the excitement or pressure to develop a new product. This can create specific liabilities for companies operating in heavily regulated sectors like financial services.
Automated decision systems that use traditional statistical analysis or machine learning algorithms are still governed by anti-discrimination laws. Courts may hold the creators of an AI program responsible for decisions that violate laws guaranteeing equal access to credit or other financial services. Even if developers consciously avoid using protected class attributes in their algorithms, they may be held responsible for AI programs that produce disparate impact, which is when policies adversely affect a particular protected class, even if that wasn't their intent. This raises the legal stakes, and judges typically have little patience for defenses that rely on the opaque processes behind an AI program.
The European Union's General Data Protection Regulation (GDPR) grants individuals the right to refuse having fully automated systems render consequential judgments. Even if the individual consents, the GDPR imposes transparency and accountability requirements on such processes. The Hong Kong Monetary Authority recently issued guidelines on the use of AI in banking that hold humans responsible for AI behavior. This is just the beginning.
It is difficult to imagine a legal regime where AI processes may cause harm to individuals without accountability. Developers, manufacturers, sellers, owners, and users of AI-based products and services will need to address and contractually define AI product liability.
Because legal doctrines of contributory liability in this industry are still developing, businesses must take a proactive approach to mitigate potential liabilities that may arise from AI tools. That approach should include transparent and explainable processes for developing their products, clear and conspicuous disclosures to the public using their products and a culture of open dialogue with the communities they seek to serve.
How to remove bias from AI deployments
AI projects have the best chance for success when the data engineers building the product work with the leadership team requesting it to develop consensus on what fairness frameworks will be embedded in the AI before launch.
The first step to removing bias from AI projects is to create a diverse internal group of stakeholders tasked with anticipating issues, conducting diligence and providing oversight and expert guidance. This group must maintain sufficient independence and authority to remain effective. In addition to understanding the risks involved, it is essential to understand the context in which the AI project will be deployed and how those risks will impact the organization.
Data inputs should be as comprehensive as possible and supplemented frequently. Think outside of the expected user group or the typical customer base, and maintain flexibility as the process unfolds. Spend time developing models for what "fair" looks like on each project -- with dozens of mathematical definitions of fair, choosing one or more necessarily excludes other models.
Ensure that the governance group is staffed by a cross-section of individuals with myriad backgrounds, identities, experiences and disciplines. A homogeneous committee simply reinforces the conscious and unconscious biases of the organization and groupthink has been at the root of many disasters. Many times these disasters are easily foreseeable by those outside of the group.
Before development, consider reaching out to a group of independent advisors to oversee the process. Although secrecy around these projects often demands a certain level of insularity, it will be difficult to avoid inherent biases in the AI program without the benefit of a range of perspectives. An independent advisory team, carefully curated and bound by obligations of confidentiality, can help establish a culture of interrogation, analysis and advice that endures as the project progresses.
Prior to launch, AI tools must be thoroughly tested, including auditing and validating process, results and outcomes of the solution, intended or unintended. Product development, risk, trust and legal teams should be primarily responsible for making sure all goes smoothly, but the governance group should oversee their efforts. The organization must also implement processes to continually monitor and validate AI products after they are launched.
The considerations and frameworks discussed here must also be integrated into the organization's broader governance structure, up to and including the board of directors. The board of directors may ultimately be held accountable for legal and social impacts of any new products and services, as well as the ethical use and handling of data. Adopting a transparent process, with all relevant stakeholders regularly educated on the progress of AI projects and their potential challenges, can prevent undesirable outcomes.
Properly designed and deployed AI programs can actually lessen the impact of discrimination. A study by researchers at the University of California, Berkeley shows that algorithmic lending models discriminated 40% less than face-to-face lenders for mortgage refinancing loans. While this is encouraging, the rate at which discrimination played a role in face-to-face loan transactions reinforces the presence of bias in all human actors.
Algorithmic lending that reduces the impact of bias is the result of an ethical and conscientious approach to AI development. It is not an indication that AI-based financial products and services are a panacea to discrimination in lending or other financial transactions.