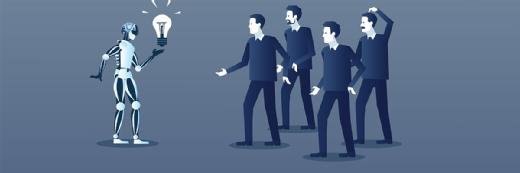
Common sense in AI remains elusive
While AI and machine learning have made major improvements and advancements to computers, common sense in AI has proven to be a significant challenge.
Artificial intelligence has come a long way since the term was first coined in 1956. Today, we have computers that can carry out complex tasks, vehicles that can operate autonomously and computers that can recognize objects and people in images. AI and machine learning have helped create and improve these advances.
Machine learning gives computers a way to learn from data and examples. Existing forms of machine learning -- supervised, unsupervised and reinforcement -- provide a range of methods for a computer to take information and create generalizations that it can apply to new data it has not seen before. But there are many situations that you can't train a system to understand.
Without cracking the code on how humans reason and apply common sense, however, we will never be able to achieve the goals of Artificial General Intelligence (AGI). Without being able to learn common sense, a computer is less able to adapt to new situations or changes.
Why is common sense reasoning so hard?
One of the reasons why enabling computers with common sense is so difficult is that machines require data and need to find repetitive patterns in that data to learn. Without these patterns, the machine has no way of reasoning with the data. It is possible to teach an AI how to learn about a specific situation or area, such as playing chess or a video game, but it isn't possible to teach that same system how to learn to play different games with the current set of data it was trained on.
Humans have generalized intelligence, but machines are not able to handle this level of understanding with significant ability. Much progress has been made specifically on this area of machine learning, with research clearly showing the ability to process and understand unstructured data such as images and text.
However, accomplishing machine learning is not sufficient to achieve the goals of artificial intelligence. Machines that can learn on their own and generalize from previous experiences without specifically being trained on that data would open the possibility of a whole selection of new applications for AI.
There has been some recent work in applying limited forms of this common-sense reasoning in existing AI systems. Companies using machine reasoning can understand conversations better than simply using natural language systems that have been pre-configured by humans with "intents." A machine is not capable of understanding any conversation that it has not been configured to handle with machine learning-based approaches. But tentative applications of common sense and machine reasoning solutions add more power to these systems.
In one application, the Cleveland Clinic tested an AI-backed system with more advanced common-sense reasoning capabilities. This enabled users to ask more open-ended questions in surveys. The system is able to use common-sense NLP to create a query interface of biomedical information spanning decades of information on cardiothoracic surgeries.
Cyc: The longest-running common sense AI project
One of the longest running AI projects is focused squarely on the problem of common sense and machine reasoning. Coming out of the second wave of AI, which was focused on symbolic reasoning, the Cyc project began in July 1984 by Douglas Lenat. The goal of the Cyc project is to encode human common sense in a way that can be applied in an adaptive manner.
The Cyc project uses a comprehensive ontology and knowledge base of basic concepts and rules of how the world works. Cyc gathers data from a number of sources and codifies it into a custom language, CycL, which it then stores in a knowledge base. In addition to being able to store all of this data, the system is able to create inferences from it. The general common-sense rules and knowledge base in Cyc continue to grow. The Cyc knowledge base of general common-sense rules and assertions was at about 1 million entries in 1994 and grew to about 24.5 million entries by 2017. While this growth is impressive, we still have a way to go to get the knowledge base to be equivalent to what adult humans are capable of understanding.
At MIT Media Lab you will find a similar project being worked on called the Open Mind Common Sense (OMCS) Project, which was started in September 1999. The idea behind the project is the same: to establish a large knowledge base of facts, most of which we humans take for granted. Most of the work behind OMCS has been done via a website created by MIT to take information and contributions from many thousands of individuals across the web and convert to computer knowledge. Since its founding, OMCS has accumulated more than one million English facts from over 15,000 contributors.
The world of machine learning continues to make great strides. Being able to have semi-autonomous cars on the road, have a conversation with a device and having hyperpersonalized news, content and product recommendations is no small feat. However, there is still a long way to go before computers are able to have generalized intelligence and we might be hitting limits with what AI is able to accomplish.
Methods for accomplishing common sense are difficult and in many ways still beyond our grasp. Because of this, we're already hitting limits of what's possible with machine learning. This absence of common-sense AI is perhaps the most significant barrier between the narrowly-focused AI applications we currently have and the more general AI applications that we ultimately want.