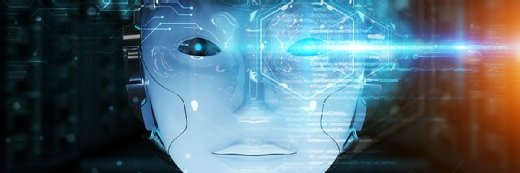
sdecoret - stock.adobe.com
Combating racial bias in AI
By employing a diverse team to work on AI models, using large, diverse training sets, and keeping a sharp eye out, enterprises can root out bias in their AI models.
Peoples' perceptions of the world around them are shaped by their preferences and biases. While some of these preferences are innocuous, many biases are harmful, resulting in the exclusion, marginalization or targeting of groups.
It should come as little surprise that these biases end up in the data that we use to inform our algorithmic decision-making systems. When left unchecked, biased data creates skewed or inaccurate machine learning models that perpetuate social prejudices and injustices. This can present a challenge to organizations, which increasingly rely on data for many operations.
How AI bias furthers racial bias
There are many ways in which data can reflect biases. Data collection suffers from different biases that can result in the underrepresentation or overrepresentation of certain groups, populations or categories. This is especially the case when multiple data sets are combined and used in aggregate.
Data might become tainted through the under-selection or over-selection of certain communities, groups or races. Give extra attention to historical data, especially in areas that have been riddled with prejudicial bias, to make sure new models created with this data don't incorporate those historical biases or injustices.
On numerous platforms, AI-based facial recognition systems have problems recognizing women and people of color as accurately as they can identify white men. Much of this problem is due to the lack of diverse training data the models were trained on. A study from Joy Buolamwini, a researcher at the MIT Media Lab, showed that nearly 35% of black women were misidentified by prominent facial recognition systems. Suppose police, governments or organizations use this kind of technology. In that case, systems could incorrectly identify certain groups of individuals nearly one-third of the time, resulting in the incorrect arrest of individuals or denying them access to systems, buildings or other important information.
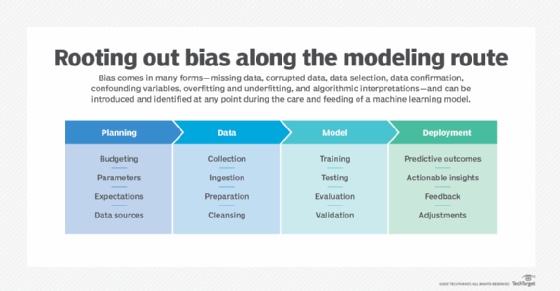
In 2015, Google's image recognition model in Google Photos incorrectly classified black people as gorillas. When called out by those in the industry and media, the company promised to fix the problem. However, instead of retraining its models, the company blocked its image recognition algorithms from identifying gorillas altogether. Most likely due to a lack of sufficient training data for people of color, Google's image recognition model could not learn how to identify people of color.
Injustices perpetrated by AI
Hiring algorithms especially fall victim to both gender and racial biases. For companies that receive large volumes of applications regularly, it's nearly impossible for a human to go through and review each resume manually. Some companies use automation filters to take a first pass and weed out resumes and candidates that do not meet the job requirements. However, these resume scanners are typically trained on past company hires and successes. So, if a company historically hired more men than women, a scanner could automatically favor male candidates over female candidates.
Biased data can also further racial injustices, as evident in Correctional Offender Management Profiling for Alternative Sanctions software, or COMPAS for short. COMPAS is used by courts in the United States to assess the likelihood of a defendant becoming a recidivist and helps make decisions on court sentences. With the help of AI, the system helps courts determine how likely someone is to commit a crime in the future.
Yet, experts have found racial biases in the system, including evidence that the system tends to predict higher recidivism rates for people of color, even when their circumstances were similar to circumstances of white defendants.
Going beyond training data
In order to address racial bias and injustice, broader representation is needed in the entire AI process, from design, model iteration, deployment and governance of AI systems. This includes hiring more women, people of color and people from various backgrounds, to have broader representation on teams. Experts argue that more diverse AI teams produce more diverse training data sets that are more representative of society at large.
Companies and organizations need to recognize there's a range of different biases that can end up in their data and therefore end up in their machine learning models. By understanding and acknowledging this, creators can better detect and potentially reduce bias in their models.
When bias becomes embedded in machine learning models, it impacts our daily lives. Bias can show itself in the form of exclusion, such as certain groups or types of individuals denied loans, passed over for jobs or denied access to resources. As AI continues to become an increasing part of our everyday lives, the risks from bias only grow larger.
Companies, researchers and developers have a responsibility to minimize bias in AI systems and ensure data sets and AI teams are representative and that the interpretations of data sets are correctly understood.