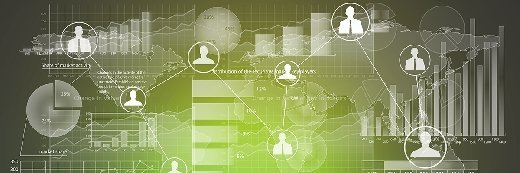
adam121 - Fotolia
Clashes between AI and data privacy affect model training
Enterprises' lax data rules reveal weaknesses around AI and model training -- particularly machine learning's reliance on unrestrained big data collection.
Data is at the heart of AI, fueling machine learning models to help companies obtain more accurate predictions, gain better insights and increase sales. Recently, the way companies are acquiring and using the data that powers those models is being evaluated.
For many years, companies have been complacent toward how their third parties obtain critical data. But recent headline-making data breaches and consumer rights advocates have called these third-party data collection practices into question. Users, businesses and governments are now increasingly concerned about the usage and widespread sharing of data, and these concerns are starting to have an impact. The relationship between AI and data privacy is set to affect how machine learning models are being trained, shared and deployed.
Data breaches lead to AI concerns
Until recently, many consumers paid little attention to the collection of their data, handing over their sensitive data freely and loosely to get free online products and services. This data -- collected over many years -- is the data now fueling machine learning models, but it can also be used by third parties with innocent or malicious intents to expand the possibility for identity theft, impersonation or other improper usages.
Lax enterprise data collection policies taken advantage of by third parties were showcased in 2018's Cambridge Analytica-Facebook scandal. This data science company used AI to harvest data taken from social media profiles to identify key individuals and advertise politically in an attempt to influence their actions in a few different recent elections. Cambridge Analytica obtained information on millions of users without consent and then quietly overtook their social media experience to manipulate their feeds, primarily due to Facebook's lack of personal data sharing regulations.
In the same way AI can manipulate user experiences, AI has already proven it is an increasingly valuable tool in the hands of hackers. While hackers have to build profiles to carry out targeted hacking campaigns, AI grants them the ability to profile victims in a scalable manner in order to set them up for complex hacking attempts -- even stealing over $240,000 from a corporate bank account by using deepfake technology to mimic the voice of CEOs authorizing a wire transfer. These systems can be further used to impersonate users on other systems, causing a range of problems across multiple platforms.
Regulation in the era of data sharing
The Cambridge Analytica scandal exposed a major issue surrounding the use and transparency of customer data, exposing how large companies are handling -- or, rather, mishandling -- user data.
With many other enterprises experiencing severe, brand-damaging cybersecurity attacks and data theft, customers are concerned their critical personal information is being misused. In response, the California Consumer Privacy Act, signed into law in June 2018 and taking effect on Jan. 1, 2020, provides guidelines on the use and sharing of personal information and data, the right to be forgotten by online companies, notices of data collection, rights to access and correct personal data, and the right to access and move your own data. This law surrounding AI and data privacy has an impact on transparency, given the requirements that personal data may not be allowed to be used to train AI models.
Other governing bodies, such as the EU, have gone one step further and given users the right to delete their data profiles and opt out of data collection. Under new rules from the General Data Protection Regulation, customers have the right to remove their data from a company's database. However, when training an AI model, data is pooled together and used anonymously or semi-anonymously. While this law definitely tightens the reins on how companies can use data, machine learning modeling may be an exception to the strict rules.
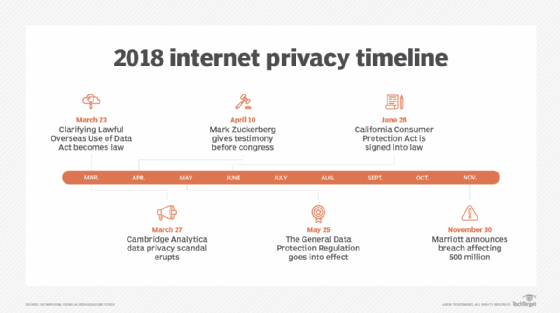
Big challenge: Data sharing in a data compliance environment
The problem posed by the newfound pushback against data sharing and stronger data regulation laws is navigating how organizations can train and create machine learning models in this new environment. On the one hand, organizations have a desire to gain a better understanding of their consumers and use insights from their data. On the other, possibly stronger hand, the compliance and security portions of their organizations are preventing the access and use of the necessary data sets that would train models.
This tension between increasing the use and spread of data for higher-powered machine learning models and the desire to lock down access to that same data is causing challenges for many organizations. Regardless of whether the disclosures are intentional or not, organizations slowly have to come up with new approaches to data collections that satisfy the desires of data scientists and information security officers.
While data collection is fueling much of the AI growth of the past decade, the use and misuse of data is increasingly also grabbing headlines as a cause for concern. In addition to addressing challenges of AI ethics and responsibility, enterprises will need to find a balance between the use of data for models that can provide benefits to their customers and the need for tighter control of data to prevent security breaches, maintain privacy and comply with emerging regulations.