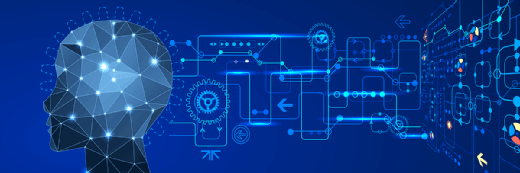
Better together: Predictive analytics and AI boost each other
Enterprises have long seen the value of predictive analytics, but now that AI is starting to influence forecasting tools, the benefits may start to go even deeper.
Through machine learning models, companies in retail, insurance, energy, meteorology, marketing, healthcare and other industries are seeing the benefits of predictive analytics tools. With these tools, companies can predict customer behavior, foresee equipment failure, improve forecasting, identify and select the best product fit for customers, and improve data matching, among other things.
Enterprises of all sizes are now finding that the combination of predictive analytics and AI can help them stay ahead of their competitors.
Forecasting gets a boost with AI
Retail brands are constantly looking to stay relevant by associating themselves with the latest trends. Before each season, designers are hard at work coming up with new styles and designs they think will be hits. However, these predictions can be faulty based on a number of factors, such as changes in customer buying patterns, changing tastes in particular colors or styles, and other factors that are difficult to predict.
AI-based approaches to demand projection can reduce forecasting errors by up to 50%, according to Business of Fashion. This improvement can mean big savings for a retail brand's bottom line and positive ROI for organizations that are inventory-sensitive.
Another industry that has seen tremendous improvements is meteorology and weather forecasting. Traditionally, weather forecasting has been prone to error. However, that is changing, as the accuracy of 5-day forecasts and hurricane tracking forecasts has improved dramatically in recent years.
According to the Weather Channel, hurricane track forecasts are now more accurate five days in advance than two-day forecasts were in 1992. These extra few days can give people in a hurricane's path extra time to prepare and evacuate, potentially saving lives.
Utility companies are also using predictive analytics to help spot trends in energy usage. Smart meters monitor activity and notify customers of consumption spikes at certain times of the day, helping them cut back on power usage. Utility companies are also helping customers predict when they might get a high bill based of a variety of data points and can send out alerts to warn customers if they are running up a large bill that month.
Reducing downtime and disturbance
For industries that heavily rely on equipment, such as manufacturing, agriculture, energy or mining, unexpected downtime can be costly. Companies are increasingly using predictive analytics and AI systems to help detect and prevent failures.
AI-enabled predictive maintenance systems can self-monitor and report equipment issues in real time. IoT sensors attached to critical equipment can gather real-time data, spotting issues or potential problems as they arise and notifying teams so they can respond to them right away. The systems can also formulate predictions of upcoming issues, reducing costly unplanned downtime.
Power plants need to be monitored constantly to make sure they are functioning properly and safely and to make sure they are providing energy to the many customers that rely on them. Predictive analytics is being used to help run early warning systems that can identify anomalies and notify managers of issues weeks to months earlier than traditional warning systems. This can lead to improved maintenance planning and more efficient prioritization of maintenance activities.
Additionally, AI can help predict when a component or piece of equipment might fail, reducing unexpected equipment failure and unplanned downtime, while also lowering maintenance costs.
In industries such as mining, which rely heavily on location data, making sure you're operating in the correct area is paramount. Goldcorp, one of the largest gold mining companies in the world, partnered with IBM Watson to improve its targeting of new deposits of gold.
By analyzing previously collected data, IBM Watson was able to improve geologists' accuracy of finding new gold deposits. Through the use of predictive analytics, the company was able to gather new information from existing data, better determine specific areas to explore next, and reach high-value exploration targets faster.
Increased situational awareness
Predictive analytics and AI are also great at anticipating situational events by collecting data from the environment and making decisions based on that data. This is different from object recognition, for example, because the system is helping to predict future events rather than reacting to current data.
Brands need to stay on top of their online presence, as well as what's being said about them on social media. Tracking social media to get real-time feedback from customers is important, especially for retail brands and restaurants. Bad reviews and negative comments can be detrimental, particularly for smaller brands.
With this awareness and by tracking comments on social media in near real-time, companies can gather immediate feedback and respond to situations quickly. Situational awareness can also help with competition tracking, market awareness, market trend predictions and anticipated geopolitical problems.
With companies of all sizes in every industry trying to stay ahead of their competitors and predict market trends, this forward-looking approach of predictive analytics is proving valuable. Predictive analytics is such a core part of AI application development that it is one of the core seven patterns of AI identified by AI market research and analysis firm Cognilytica.
The use of machine learning to help give humans more data to make better decisions is compelling, and it's one of the more beneficial uses of machine learning technology.