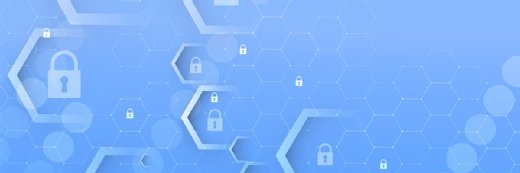
Vitalii Gulenok/istock via Getty
Balancing generative AI cybersecurity risks and rewards
At the MIT Sloan CIO Symposium, enterprise leaders grappled with AI's benefits and risks, emphasizing the need for cross-team collaboration, security controls and responsible AI.
CAMBRIDGE, MASS. -- As AI tools and systems have proliferated across enterprises, organizations are increasingly questioning the value of these tools compared with the security risks they might pose.
At the 2024 MIT Sloan CIO Symposium this week, industry leaders discussed the challenge of balancing AI's benefits with its security risks.
Since the introduction of ChatGPT in 2022, generative AI has become a particular concern. These tools have many use cases in business settings, from virtual help desk assistance to code generation.
"[AI] has moved from theoretical to practical, and I think that has raised [its] visibility," said Jeffrey Wheatman, cyber-risk evangelist at Black Kite, in an interview.
McKinsey & Company partner Jan Shelly Brown helps companies in the financial sector and other highly regulated industries evaluate the risk profiles of new technologies. Increasingly, this involves AI integration, which can introduce both business value and unforeseen risks.
"The cybersecurity agenda, because technology is woven into every corner of the business, becomes super, super important," Brown said in an interview.
The balancing act
Introducing AI into the enterprise brings cybersecurity benefits as well as drawbacks.
On the security front, AI tools can quickly analyze and detect potential risks, Wheatman said. Incorporating AI can bolster existing security practices, such as incident detection, automated penetration testing and rapid attack simulation.
"AI is starting to get really good at running through millions of iterations and determining which ones are actually real risks and which ones are not," Wheatman said.
While generative AI has seen increased use across enterprises, its security applications are still in the early stages.

"We believe that it's far too early yet for GenAI to be a core pillar of cyber preparedness," said Fahim Siddiqui, executive vice president and CIO at The Home Depot, in the panel "AI Barbarians at the Gate: The New Battleground of Cybersecurity and Threat Intelligence."
But despite these reservations about generative AI in particular, Siddiqui noted, many cybersecurity tools currently in use already incorporate some type of machine learning.
Andrew Stanley, chief information security officer and global digital operations vice president at Mars Inc., described the high-level benefits that generative AI can bring to enterprises in his presentation "The Path Goldilocks Should Have Taken: Balancing GenAI and Cybersecurity." One of these advantages is bridging gaps in technical knowledge.
"The really powerful thing that generative AI brings into security is the ability to allow ... nontechnical people to engage in technical analysis," Stanley said in his presentation.
Due to the technology's various benefits, businesses are increasingly using AI -- including generative AI -- in their workflows, often in the form of third-party or open source tools. Brown said she's seen extensive adoption of third-party tools within organizations. But organizations often don't know exactly how those tools use AI or manage data. Instead, they must rely on external vendor assessments and trust.
"That brings a whole different risk profile into the organization," Brown said.
The alternatives -- custom LLMs and other generative AI tools -- are currently less widely adopted among enterprises. Brown noted that while organizations are interested in custom generative AI, the process of identifying valuable use cases, garnering the right skill sets and investing in the necessary infrastructure is much more complex than using an off-the-shelf tool.
Regardless of whether an organization chooses a custom or third-party option, AI tools introduce new risk profiles and potential attack vectors, such as data poisoning, prompt injection and insider threats.
"The data starts to show you that in many cases, the threats may not exist outside the organization -- they can exist within," Brown said. "Your own employees can be a threat vector."
This risk includes shadow AI, where employees use unsanctioned AI tools, making it difficult for security teams to pinpoint threats and develop mitigation strategies. Explicit security breaches can also occur when malicious employees exploit poor governance and privacy controls to access AI tools.
The widespread availability of AI tools also means that external bad actors can use AI in unanticipated and harmful ways. "Defenders need to be perfect or close to perfect," Wheatman said. "The attackers only really need to find one way in -- one attack vector."
Threats from bad actors are even more concerning when cybersecurity teams aren't well versed in AI -- one of the many AI-related risks that organizations are starting to address. "A very low percentage of cybersecurity professionals really have the right AI background," Wheatman said.
Moving toward cyber resilience
When using AI in business settings, completely eliminating risk is impossible, Brown said.
As AI becomes integral to business operations, the key is instead to deploy it in a way that balances benefits with acceptable risk levels. Developing a plan for AI cyber resilience in the enterprise requires comprehensive risk evaluation, cross-team collaboration, internal policy frameworks and responsible AI training.
Risk level evaluation
First, Brown said, organizations must determine their risk appetite: the level of risk they're comfortable introducing into their workflows. Organizations should evaluate the value that a new AI tool or system could offer the business, then compare that value with the potential risks. With proper controls in place, organizations can then decide if they feel comfortable with the risk-value tradeoff.
Wheatman suggested a similar approach, suggesting that organizations consider factors such as revenue impact, effects on customers, reputational risk and regulatory concerns. In particular, prioritizing tangible risks over more theoretical threats can help companies efficiently assess their situation and move forward.
Cross-team collaboration
Nearly everyone in the enterprise has a role in secure AI use. "Organizationally, this is not a problem to be assessed or addressed by one team," Wheatman said.
Although data scientists, application developers, IT, security and legal are all exposed to potential risks from AI, "right now, everybody's having very separate conversations," he said.
Brown raised a similar point, explaining that teams across a wide range of functions -- from cybersecurity to risk management to finance and HR -- need to participate in risk evaluation.
For some organizations, this level of cross-collaboration might be new, but it's gaining traction. Data science and security teams in particular are starting to work closer together, which historically has not been the norm, Wheatman said. Bringing together these different aspects of AI workflows can shore up organizational defenses and ensure that everyone is aware of what AI tools and systems are brought into the organization.
Internal policy framework
After they initially connect, teams need to find a way to get on the same page. "If the organization doesn't have [a] framework to snap into, these conversations become very hard," Brown said.
"[In] a lot of organizations, most people don't even have a policy," Wheatman said. This can make it very difficult to answer questions such as what the AI tool is used for, what data it touches, and who uses it and why.
While the details of an AI security framework will be unique to each organization, comprehensive policies usually include access authorization levels, regulatory standards for AI use, mitigation procedures for security breaches and employee training plans.
Responsible AI training
With all the use cases and hype surrounding AI -- and especially generative AI -- in enterprises, there is genuine concern about developing overdependence and misplaced trust in AI systems, Brown said. Even with the right collaboration and policies, users need to be trained in responsible AI use.
"Generative AI specifically can so aggressively undermine what we all agree is right ... and it does so through natural means of trust," Stanley said during his presentation. He encouraged business leaders to reframe internal conversations around trust by telling users that "it's OK to be skeptical" about AI.
Generative AI has been responsible for uncanny deepfakes, biased algorithms and hallucinations, among other misleading outputs. Companies need strict plans in place to educate their employees and other users on how to use AI responsibly: with a healthy dose of skepticism and a strong understanding of the ethical issues raised by AI tools.
For instance, the data that LLMs are trained on is often implicitly biased, Brown said. In practice, models can propagate those biases, resulting in harmful outcomes for marginalized communities and adding a new dimension to an AI tool's risk profile. "That is not something a cyber control can mitigate," she said.
Therefore, organizations need to train their employees and technology users to always check a tool's output and be skeptical in any AI use, rather than relying solely on an AI system. Investing in the changes needed to safely incorporate AI technology into an organization can be even more expensive than investing in the actual AI product, Brown said.
This can include a wide range of necessary changes, such as responsible AI training, framework implementation and cross-team collaboration. But when businesses put in the necessary time, effort and budget to protect against AI cybersecurity risk, they'll be better positioned to reap the technology's rewards.
Olivia Wisbey is associate site editor for TechTarget Enterprise AI. She graduated with Bachelor of Arts degrees in English literature and political science from Colgate University, where she served as a peer writing consultant at the university's Writing and Speaking Center.