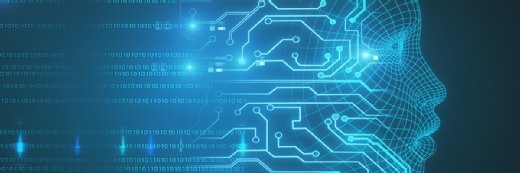
peshkov - stock.adobe.com
Are giant AI chips the future of AI hardware?
Giant AI chips like the Cerebras WSE are dazzlingly fast and could transform AI models, but how soon is the question for CIOs. Experts mull the merits of small vs. big AI chips.
New types of AI chips that adopt different ways of organizing memory, compute and networking could reshape the way leading enterprises design and deploy AI algorithms.
At least one vendor, Cerebras Systems, has begun testing a single chip about the size of an iPad that moves data around thousands of times faster than existing AI chips. This could open opportunities for developers to experiment with new kinds of AI algorithms.
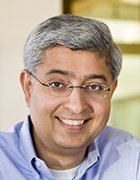
"This is a massive market opportunity and I see a complete rethink of computer architecture in progress," said Ashmeet Sidana, chief engineer at Engineering Capital, a VC firm.
The rethink is long overdue, Sidana noted. The industry has historically focused on scaling simple machine learning workloads on top of traditional computer architectures rather than thinking about the most appropriate way of building an AI-specific computer.
But the situation is changing rapidly as startups like Cerebras and Graphcore, along with established players like Intel, with its purchase of Habana Labs, race to build a new generation of AI chips.
In the short run, these kinds of advances will have the biggest impact on companies that are AI-heavy. In the long run, enterprises of all sizes will have to rethink their IT, data engineering and data science processes to stay on the cutting edge.
Addressing the bandwidth bottleneck in traditional AI hardware
Cerebras shocked the industry when it introduced the Wafer Scale Engine (WSE) chip: The size of a whole silicon wafer, the WSE chip is 56 times bigger and holds 78 times more cores than the biggest GPU. But its real advantage lies in how fast it can move data around -- over 10,000 times faster.
"The biggest innovation that I find in this solution is the integration of memory with such a high bandwidth," said Tom Hackenberg, senior principal analyst and associate director of processors at research firm Omdia.
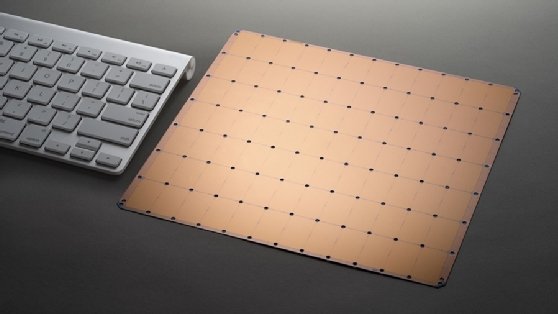
"Memory access and configuration is one of the technologies that many new startups are [tackling] because traditional large-scale memory addresses are not well optimized for neural networking algorithms," he said.
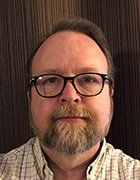
A big bottleneck in traditional hardware architecture for AI lies in the time it takes to go between different processing cores, memory and the processing cores on other chips. Consequently, AI algorithms need to be optimized to minimize the requirements for communication between cores. This applies whether the algorithms are run on multiple CPUs or multiple GPUs.
The cores on the WSE are more densely interconnected than on a traditional chip. This allows faster communication between cores and between the cores and the on-board RAM, called SRAM. Also, the there is more SRAM stored on the same substrate as the processing cores. The WSE has 32 gigabytes of SRAM compared to a few dozen megabytes for a traditional chip. The dense interconnect allows the data to move between memory and processor at 9 petabytes per second and between cores at 100 petabytes per second.
In a typical computer, the intermediate steps in a calculation are stored in DRAM attached to the motherboard, which is much slower than SRAM, but faster than external storage.
"DRAM is like the grocery story around the corner, whereas SRAM is like your fridge," said Andrew Feldman, CEO of Cerebras. If you want to get something fast, it better fit in the fridge, he said, riffing on the analogy. It does not matter how big the grocery store is, because you are going to miss some of the football game if you have to go to the grocery store to get a beer.
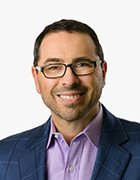
By comparison, a state-of-the-art GPU like the Tesla V100 has a GPU memory bandwidth of 900 gigabytes per second.
Other AI chip providers are also easing the bandwidth bottleneck. For example, Graphcore's Intelligence Processing Unit sports 300 megabytes of SRAM and 45 terabytes per second of memory bandwidth.
The effort to rethink how memory and compute are organized may ultimately solve some of the memory access barriers of traditional systems. It could also dramatically reduce power requirements, Hackenberg said. This would appeal to cloud-services superscalars that are already dabbling in AI accelerator coprocessors -- Alibaba, Alphabet, Amazon and Microsoft are among those that have designed their own ASIC solutions.
Ecosystem support is key for new AI chips
Cerebras, like all new chip vendors, must invest in making its big AI chips work with existing AI development frameworks like TensorFlow and PyTorch. But the jury is still out on how well the WSE and big AI chips like it will work with AI development workflows.
"It will take not only performance per watt, but also significant ecosystem support, services and pricing incentives to pry away market share from leaders such as AMD, Intel, Nvidia or Xilinx," Omdia's Hackenberg cautioned.
The largest vendors in this market provide not only economies of scale, but also many years of ecosystem support, Hackenberg said. To move from beta-testing to commercial viability, startups in this space will need to offer a lot of incentives for buyers to abandon their traditional suppliers -- including marked performance improvement, cost savings or a little of both.
"Long-term relationships and ecosystem support are often underestimated by startups," Hackenberg said.
Others are more hopeful about the potential of the giant AI chips and newer architectures to prove their value.
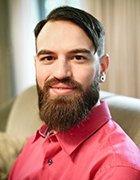
Agustin Huerta, vice president of technology at the IT consultancy Globant, said chip providers are making it easier for companies to use the bigger AI chips.
"In my personal experience, chip providers are also willing to be closer to enterprises than before and provide significant inputs, unlike traditional CPU providers," said Huerta, who leads Globant's AI and process automation studio.
Chip providers are working directly with end users to optimize their chips for different use cases and architectures, he said. The support relieves AI teams of having to do this this work. It also means that AI teams can write an algorithm once and recompile it for a variety of different target production environments.
In praise of problem-solving chiplets
That said, Huerta expects that bigger chips will mainly be used by enterprises where AI applications are the core of their business or by companies who provide development services on AI. Giant AI chips will allow them to invest more time in fine-tuning the models or identifying cases when additional training does not return the expected results. For many other companies, however, these chips will not be relevant -- at least not in the near future.
"Most enterprises, like banks or retailers, will scarcely use those solutions -- there is just too much computing power for them to really make good use of it," he said. "For more mainstream corporate usage, larger chips could be a waste of resources."
Indeed, Hackenberg cautioned that at this stage it is only a theory that bigger chips will gain traction. Many enterprises will see a better ROI by adopting smaller chips that cost less to buy and run. Indeed, the market trend is towards smaller chips. "Smaller tends to improve performance per watt and better yield to manufacture," he said.
Traditional processor suppliers are trying to develop less costly AI hardware, such as chiplets -- modular pieces that can be combined into larger heterogeneous processors to add bandwidth and run specialized algorithms.
Huerta believes these smaller, low-powered chips could enable the use of AI on devices that cannot reliably connect to a network such as robotics arms, delivery bots and remote equipment automation.
A giant AI chip has a different function. Larger chips promise to open new avenues of AI research, argued Cerebras' Feldman.
"When you have new hardware that will allow different things, you can write different models," he said. So far, data scientists have only explored a subset of algorithms tailored to the characteristics of GPUs. They are writing the same kind of algorithms to run faster.
But down the road, Feldman said he expects researchers to explore new models that could include larger networks, deeper networks or extraordinarily sparse networks.
Rethinking IT for AI: First things first
New hardware -- including AI chips the size of an iPad -- will not provide a major competitive advantage for most enterprises.
Rather, Engineering Capital's Sidana said CIOs should focus on the fundamentals, starting with building a machine learning pipeline capable of consistently pushing new models into production. For many CIOs the production number will be in the single digits, whereas AI powerhouses will have thousands of models in production.
In either case, CIOs tasked with overseeing AI initiatives will need to reevaluate the way they purchase hardware and services, said Globant's Huerta.
"One misstep many companies make is buying inadequate hardware for the current needs in the AI space," he said. Many IT departments rely on their own knowledge of traditional server needs and base their investment decisions on the same factors they used in the past, unaware of the particular infrastructure requirements for deploying AI.
He said CIOs must ensure their teams accurately anticipate their AI needs to avoid overspending on capabilities that will never be really used. The analysis can also help pinpoint where AI infrastructure is falling short, resulting, for example, in long delays when running mission critical applications.
The major challenge for companies, in Huerta's experience, is to determine which is the right hardware or cloud solution to use for their specific computing needs. In many cases, renting AI processing capabilities from cloud providers could have a larger ROI for companies than buying and running their own servers. The key, he said, here is to work with partners from software development, cloud and hardware perspectives to develop a comprehensive understanding of requirements and costs.
Successful AI also takes vigilance, Sidana said. AI teams must be able to retrain models when they lose accuracy. Traditional enterprises may spend months to retrain a model, whereas the most sophisticated companies can do it daily or even every minute.
"Most enterprises still have a few orders of magnitude to improve," Sidana said, before they get to high level AI production.