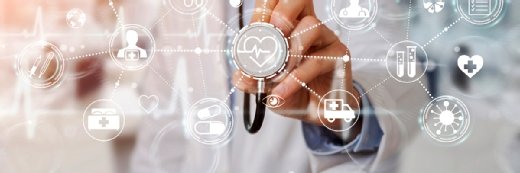
sdecoret - stock.adobe.com
AI in medical diagnostics and decision-making
AI technologies enable doctors to improve diagnostic accuracy, personalize treatment plans and streamline clinical workflows. However, they also pose challenges worth considering.
Rapid advances in AI and machine learning are reshaping healthcare. Among the most affected areas are medical diagnostics and clinical decision-making -- integral aspects of medicine now getting a boost from AI capabilities.
Historically, diagnostics has been a mainly human endeavor, dependent on physical examination techniques like pulse checks and palpation. In the last century, scientific advances have introduced important diagnostic tools such as laboratory testing and medical imaging. Although these new elements enable more precise diagnoses, diagnostics still heavily relies on doctors' interpretations to identify conditions and prescribe the best treatments.
AI is a rapidly growing element in medical diagnostics. A recent study in the journal European Radiology compared GPT-4's performance with human radiologists in differential diagnoses. GPT-4 achieved 94% accuracy, outperforming the top human radiologists, whose accuracy ranged from 73% to 89%.
AI can significantly improve patient outcomes and treatment efficacy by offering new levels of speed and accuracy. Healthcare facilities are beginning to integrate AI into medical decision-making, including imaging analysis, patient outcome prediction and AI-driven drug discovery.
But these uses, while beneficial, also come with significant risks. As AI becomes more common in medicine, healthcare providers will need to address challenges like maintaining regulatory compliance, training staff, protecting patient data and avoiding algorithmic bias -- not to mention the difficulties of integrating AI into healthcare IT environments.
AI's adaptive capabilities in medical diagnostics
AI's ability to mimic aspects of human cognition and analyze high volumes of data makes it well-suited for augmenting medical diagnostics.
Machine learning models are trained on massive volumes of data from varying sources. In healthcare, that data might include the following:
- Electronic health records containing patients' medical histories.
- Medical imaging and communications data sets, such as MIMIC-CXR and CheXpert, which provide annotated chest X-rays.
- Other specialized data sets, such as LUNA16 for lung nodule analysis.
- Public clinical databases, such as MIMIC-III that contains anonymized health data collected from over 40,000 critical care patients at Beth Israel Deaconess Medical Center between 2001 and 2012.
- Federated learning systems, which enable different healthcare institutions to collaborate on AI model training while maintaining patient data privacy. For example, hospitals can jointly train AI models to detect rare cancers without sharing sensitive patient data. Each institution trains the model locally on its data, sharing only model updates to create a global model that utilizes the combined knowledge of all participants.
By integrating these data sets, AI-powered healthcare tools can assist in medical decision-making in the following ways:
- Enhanced diagnostic capabilities. Machine learning and natural language processing models can analyze massive data sets to identify anomalies and patterns that human clinicians might fail to recognize.
- Personalized treatment plans. AI tools trained on anonymized patient records can identify treatment patterns, which can then be synthesized with individual patients' medical records and factors like age, symptoms and preexisting conditions to recommend treatments.
- Support for human judgment. AI can validate a doctor's initial assessment or serve as an emergency room triage tool to help clinicians determine appropriate levels of care.
How AI is used in medical diagnostics
Many AI use cases in healthcare focus on optimizing medical diagnostics and decision-making. Major applications include analyzing medical images, predicting patient outcomes, powering virtual health assistants, performing remote diagnosis, and advancing drug discovery and personalized medicine.
Analyze medical images
AI enhances medical image analysis by automating detection, improving diagnostic accuracy and aiding in treatment planning. For instance, incorporating AI into radiology facilitates early disease detection by identifying tiny details in scans that might go unnoticed by human eyes.
Predict patient outcomes
AI can use historical and real-time data to predict patient health condition outcomes, often with greater speed and accuracy than traditional methods. It can forecast health events, patient risks and treatment responses, enabling clinicians to make timelier medical interventions.
Power virtual health assistants
AI-powered virtual health assistants can provide real-time, personalized support, handling routine tasks and improving patient engagement. Advantages include the following:
- 24/7 availability. Patients can ask questions related to symptoms, medication usage and general health advice when physicians are unavailable.
- Language comprehension. Medical virtual assistants with natural language processing capabilities can understand patient communications and guide them to appropriate care.
- Personalization. Virtual assistants can provide tailored health recommendations based on patient information.
Perform remote diagnosis
AI can support remote diagnosis by analyzing data collected from virtual assistants, wearable devices and medical imaging to provide initial assessments or escalate cases to human specialists. For example, AI-powered systems can interact with patients and assess their symptoms in real time. Wearable devices like smartwatches can collect health metrics, such as heart rate, blood pressure and blood oxygen levels. Continually monitoring patients' vital health signs can help automatically alert healthcare providers to concerning patterns.
AI-driven drug discovery
AI's benefits in drug discovery, personalized medicine and precision medicine include the following:
- Accelerated development. AI models can process massive volumes of medical data, including genomic information and scientific literature, faster than human researchers can.
- Improved drug efficiency. AI tools can help develop targeted medicine customized to individual genetics -- particularly useful for fighting complex diseases such as cancer.
- Cost reduction. By reducing laboratory testing expenses and identifying failing compounds early, AI greatly reduces drug development costs.
Other AI uses in healthcare
AI's role in the medical field extends beyond diagnostics and decision-making. Healthcare providers increasingly also use it to streamline administrative tasks like the following:
- Automating data entry from scanned documents and laboratory results into electronic health records, reducing human error and saving time.
- Validating data in medical records to ensure accuracy and completeness.
- Scheduling patients based on criteria such as urgency, doctor availability and location, reducing wait times and optimizing resource use.
- Facilitating bill processing and improving fraud detection.
- Improving human resources management and streamlining talent acquisition.
Challenges of using AI in the medical field
Although AI in healthcare offers numerous benefits, it also introduces challenges and ethical considerations.
Regulatory compliance
Medical AI systems must adhere to strict healthcare regulations, such as HIPAA, GDPR and FDA requirements, while maintaining operational efficiency. This requires establishing comprehensive frameworks with security access controls and management protocols to govern access to sensitive patient data.
Data privacy
Medical AI-powered tools depend on aggregating patient data to train models, raising patient privacy concerns. Patients might fear their sensitive medical data is being used without their explicit consent, making transparency and secure data-handling practices essential.
Integration with legacy IT systems
Many healthcare institutions worldwide still rely on outdated IT systems that cannot integrate with modern AI technology. This creates major barriers to AI adoption in healthcare, even as more medical device manufacturers integrate AI into their products. Upgrading or replacing these systems can be time-consuming, expensive and complicated.
Societal acceptance of AI
Trust in AI is not a given for patients or providers. Some patients might distrust AI diagnoses, preferring to be treated by human doctors. And those working in healthcare might fear being replaced by AI, leading to skepticism that could negatively influence patients' perceptions of AI-generated outcomes.
Reduced human skills
Overreliance on AI in medical diagnostics might create a knowledge gap among healthcare professionals. If clinicians rely too heavily on AI, they could lose opportunities to hone their skills and critical thinking, resulting in a workforce that is less proficient in diagnostics and overly dependent on technology.
Nihad A. Hassan is an independent cybersecurity consultant, expert in digital forensics and cyber open source intelligence, blogger, and book author. Hassan has been actively researching various areas of information security for more than 15 years and has developed numerous cybersecurity education courses and technical guides.