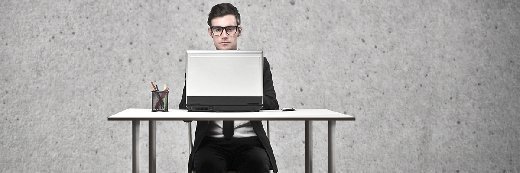
olly - Fotolia
5 mistakes that disrupt data science best practices
Through asking questions and understanding the real-world issues other parts of the company face, data scientists polish their enterprise contribution.
Data has tremendous value right now -- and businesses are relying on data to fuel everything from market spending and staffing decisions to product development, which means that data scientists are becoming more prominent in the workplace.
As AI development rises, data scientists are becoming more and more desired -- and citizen data scientists are being trained throughout companies. From our position as AI enablers to models for employee trainings, it's important that we be self-aware enough to ensure that data scientists are consistently adding value and utilizing data science best practices. Here's a look at five common mistakes data scientists make, along with how to prevent them from hurting business outcomes.
1. Focusing on computers, not colleagues
There's a common misconception among data science students that in the real world their jobs will consist of writing technical code and someone else will present their findings to business stakeholders. Nothing could be further from the truth. The job of a data scientist is to uncover information that will help a business grow.
First, data scientists must be able to communicate how the information they discover affects the larger business, and second, they must know where to look for this information. The second part is critical: a data scientist who spends the whole day at their desk may never realize that the sales team is having a problem with customer drop-off, or that the marketing team is struggling with one part of the conversion funnel.
No business is perfect, and there are plenty of problems a data scientist can help solve. Don't only look at data, step away from your desk to hear about the business's daily work so you can understand how to add the most value.
2. Ignoring the larger business context
Besides making sure you're communicating with colleagues regularly, it's important to make sure you're taking time to understand large-scale context of the business you're working in. If you're working on solutions for a retail company, take the time to drive to their physical location and observe how it operates – what the sales associates are doing, how shoppers engage with the space, how managers work, etc.
Getting the full contextual understanding is crucial to offering business insights and a key component of data science best practices. If you're not aware of how the business operates, it's impossible to help it operate better. Data scientists must understand what the data represents. Without that, you'll run into situations where everything should work perfectly according to your models -- but where there are still real-world problems that you would only know about from seeing the business in action.
When you have a sense of the larger business context, you can identify processes that aren't working, look at the data, hypothesize what's wrong, test and confirm your hypothesis, and make changes that improve operations.
3. Focusing on theory and ignoring practice
Data science, like many fields, is much thornier in practice than it is in theory. And the thing is, you can't learn how to handle the practical side of data science until you actually do it in implicational settings.
In an enterprise, data scientists must weather all kinds of forces, including:
- Coordinating with other departments and other teams. This might mean jumping from one project to another as internal priorities change or finding alternative solutions when your primary solution can't be implemented as recommended.
- Code integration challenges. Sometimes, your code can't be easily integrated with existing code, which means you must figure out a workaround.
- Budget limitations. In the real world, every project has budget limitations. Figuring out how to work within them to arrive at good-enough (rather than perfect) solutions is a key part of being effective in a data scientist role.
While it's also important to keep up with the latest articles and blogs and cutting-edge technology, there are certain parts of the job you can only learn by doing. An effective data scientist knows how to balance both parts of their professional development.
4. Not asking questions
To be a better data scientist, simply ask why. This question helps eliminate the communication barriers between data scientists and employees in other parts of the business.
Imagine a marketing leader at a retail company asks for a data model showing how much customers spend based on the channel they use to get to the website. You could create that model, or you could ask why. Is it to understand which customers are most valuable so they know where to funnel additional marketing dollars? Is it to help the sales team prioritize leads? Do they have a way to measure new versus repeat customers? Have they factored in product returns?
In order to build a truly useful model, you must understand the problem your colleague is trying to solve with it -- and when you do, you may be able to solve it more easily than you initially thought, which benefits everyone.
5. Assuming your data is clean
In many cases, 80 percent of a data scientist's work is cleaning up data -- the last 20 percent is running machine learning or deep learning models to come up with an insight.
The first step to take when receiving a data set is deciphering how much you can rely on it, and step two is determining what you'll have to do to make it usable.
Data is never perfect -- if it was, data scientists wouldn't have jobs. We have to make imperfect data usable, which requires us to understand the larger business context (which pieces of information don't you need? Which ones are mission critical?).
It's easy to fall into the contemporary mindset that data is the source of all meaning and value within a business (especially if you're a data scientist). But if we want to continue to bring value to the companies we work for, to utilize data science best practices, we have to acknowledge that our work is most valuable when it's part of the larger business ecosystem -- and that it's up to us to engage with that ecosystem to ensure the quality of our work.