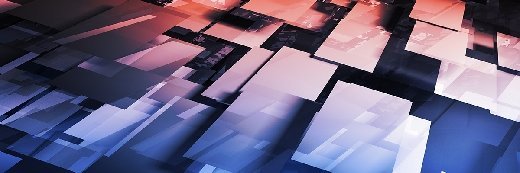
kentoh - Fotolia
3 GAN use cases that showcase their positive potential
GANs' ability to create realistic images and deepfakes have caused industry concern. But, if you dig beyond fear, GANs have practical applications that are overwhelmingly good.
Generative adversarial networks are making headlines with their unique ability to understand and recreate content with increasingly remarkable accuracy. Significant attention has been given to the GAN use cases that generate photorealistic images of faces. Programs showcase examples of completely computer-generated images that are both remarkable in their likeness to real people and concerning in how the technology could be applied.
Earlier iterations of GAN-generated images were relatively easy to identify as being computer-generated. However, the latest versions of highly trained GANs are starting to make realistic portraits of humans that can easily fool most casual observers. These GAN-generated images bring up serious concerns about privacy and identity. There's little to stop someone from creating fake social media accounts using GAN-generated images for malicious use and fraudulent activities. Further, for companies dependent on facial recognition software, these images could result in security and privacy challenges.
The genius behind GANs is their adversarial system, which is composed of two primary components: generative and discriminatory models. These models are pitted against each other in an eternal battle for perfection with one analyzing and collecting data to reference and the other generating comparable content to pit against the analysis of the other system. These neural networks enable them to not only learn and analyze images and other data, but also create them in their own unique way. Their ability to both recognize complex patterns within data and then generate content based off of those patterns is leading to advancements in several industries.
GANs in art and content creation
Though GANs open up questions of significant concern, many companies are finding ways to utilize GANs for the greater good. A GAN's ability to create a portrait can easily be used by police to create altered photos of missing persons or can make your smartphone better at recognizing your face in new circumstances.
GANs are finding a wide range of applications in creating realistic images that are new and novel. GANs are able to recognize the style of an art piece and then perfectly create new, original artwork that further mimics that style in a realistic manner. GANs can also generate and create other forms of content, from building facades that don't exist to completely generated apparel items, renditions of nature and outdoor scenes -- and even entirely fictitious, completely furnished rooms in a house. Chipmaker Nvidia, based in Santa Clara, Calif., is using GANs for a generation of high-definition and incredibly detailed virtual worlds for the future of gaming.
Since GANs create a compressed version of an ideal representation of an image, they can also be used for quick search of images and other unstructured data. Used in conjunction with unstructured data repositories, GANs retrieve and identify images that are visually similar. Rather than using some sort of file-based fingerprint, the GAN represents a compressed image representation that can be compared against other compressed image representations to give a best match.
With this idea of the compressed representation of an image in mind, you can even use GANs to generate new and novel images just from textual descriptions of an image. We can use forms of supervised learning to label the images that GANs create and then use our own human-generated textual descriptions to surface a GAN-generated image that best matches the description. This brings up the unique idea of text to image, like the concept of text to speech with machine-generated speech.
GANs in healthcare
Currently, GAN use cases in healthcare include identifying physical anomalies in lab results that could lead to a quicker diagnosis and treatment options for patients. Their ability to recognize errors in an image enables them to immediately analyze and make determinations on the health of a patient. Though they might not make the official diagnosis, they can certainly be used in an augmented intelligence approach to raise flags for medical professionals. GANs are also being used to look into medication alterations by aligning treatments with diseases to generate new medications for existing and previously incurable conditions.
Furthermore, researchers are starting to use GANs to facilitate drug discovery and novel drug creation. In much the same manner that a GAN can create a realistic image, it can create realistic drug compounds and molecules that could potentially provide new treatments for medical conditions. Researchers from Insilico Medicine, a biotechnology company based in Maryland, are using GANs to generate drug candidate compounds that might be worth further research. With GANs, researchers are finding that you can use the discriminator-generator model of GANs to rapidly try out multiple potential drug candidates and see if they will be suitable for further investigation.
GANs also hold significant promise in quality control, given their ability to quickly and accurately detect anomalies. The systems are trained to process complex data and distill it down to its smallest possible components. GANs can also make judgment calls regarding how to accurately fill gaps in data, which is being shown through GANs taking small images and making them significantly larger without compromising the image itself. This means that GANs can make educated guesses regarding what should be where and adapt accordingly.
GANs are a powerful evolution of the use of machine learning and neural networks. Since GANs are capable of analyzing and recognizing detailed data, these systems are a powerhouse for generating artificial content. Unfortunately, the current process to produce GAN-generated content requires significant human work, an excessive budget, time and technology. With the introduction of business applications, perhaps we can more easily generate this sort of realistic content to find wide and positive GAN use cases.