What is retrieval-augmented generation (RAG) in AI?
Retrieval-augmented generation (RAG) is an AI framework that retrieves data from external sources of knowledge to improve the quality of responses. This natural language processing (NLP) technique is commonly used to make large language models (LLMs) more accurate and up to date.
LLMs are AI models that power chatbots like OpenAI's ChatGPT and Google Gemini. LLMs can understand, summarize, generate and predict new content. However, they can still be inconsistent and fail at some knowledge-intensive tasks -- especially tasks that are outside their initial training data or those that require up-to-date information and transparency about how they make their decisions. When this happens, the LLM can return false information, also known as an AI hallucination.
When an LLM's trained data isn't enough, the quality of its responses can be improved by retrieving information from external sources. Retrieving information from an online source, for example, enables the LLM to access current information that it wasn't initially trained on. This process has become important for foundation AI models, chatbots and Q&A systems, as they need to respond to user queries with specific, up-to-date and accurate information.
What does RAG do and why is it important?
LLMs are a key component of modern AI systems, as they help enable AI to understand and generate human language. However, LLMs have several constraints and knowledge gaps. They're commonly trained offline, making the model unaware of any data that's created after it was trained. RAG retrieves data from outside the LLM, which then augments the LLM's response by adding relevant retrieved data to the generative response.
This article is part of
What is GenAI? Generative AI explained
This process helps reduce any apparent knowledge gaps and AI hallucinations. This is important in fields that require as much current and accurate information as possible, such as healthcare and customer support.
How to use RAG with LLMs
RAG combines a text generator model with an information retrieval component. This retrieval component searches for external knowledge, which is data gathered from anywhere outside the LLM's original training data. Information can be retrieved from several places, such as online sources, application programming interfaces, databases and document repositories.
Using the example of a chatbot, once a user inputs a prompt, RAG summarizes that prompt using vector embeddings -- which are commonly managed in vector databases -- keywords or semantic data. The converted data is sent to a search platform to retrieve the requested data, which is then sorted based on relevance.
The LLM then synthesizes the retrieved data with the augmented prompt and its internal training data to create a generated response that can be passed to the chatbot. Depending on the chatbot, sourced links can also be provided to the user.
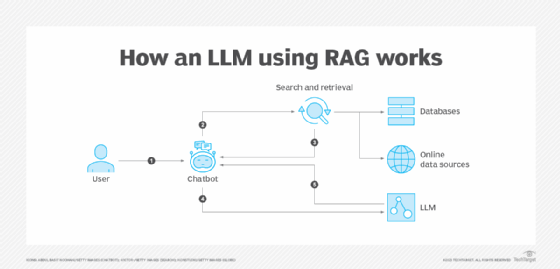
What are the benefits of RAG?
RAG offers the following benefits:
- Provides current information. RAG pulls information from relevant, reliable and up-to-date sources.
- Increases user trust. Depending on the AI implementation, users can access the model's sources, which promotes transparency and trust in the content and lets users verify its accuracy.
- Reduces AI hallucinations. Because LLMs are grounded to external data, the model has less chance to make up or return incorrect information.
- Reduces computational and financial costs. Organizations don't have to spend time and resources to continuously train the model on new data.
- Synthesizes information. RAG synthesizes data by combining relevant information from retrieval and generative models to produce a response.
- Easier to train. Because RAG uses retrieved knowledge sources, the need to train the LLM on a massive amount of data is reduced.
- Can be used for multiple tasks. Aside from chatbots, RAG can be fine-tuned for various specific use cases, such as text summarization and dialogue systems.
What are the limitations of RAG?
While RAG has numerous benefits, it also comes with its own challenges and limitations, including the following:
- Accuracy and data quality. Because RAG pulls from external sources, the accuracy of its response is only as good as the data it pulls from. RAG itself can't determine the accuracy of the data it gathers. This means that the accuracy of the retrieved data depends on the quality and reliability of its data sources.
- Computational cost. RAG requires a model and retrieval component that can integrate retrieved data efficiently, which is a resource-intensive process.
- Explainability. Some systems might not be designed to let users know where data was sourced from, which can affect user trust.
- Latency. Adding a retrieval step to an LLM can add to its latency. This is especially true if the retrieval mechanism must search through larger knowledge bases.
Retrieval augmented generation vs. semantic search
Semantic search is a data searching technique that focuses on understanding the intent and contextual meanings behind search queries. It does this by applying NLP and machine learning algorithms to various factors, such as the terms used in a query, previous searches and geographic location. This is a more effective method than keyword-based searches, which attempt to match exact words or phrases in a query. Semantic search is widely used in web search engines, content management systems, chatbots and e-commerce platforms.
While RAG attempts to improve the quality of responses from an LLM by using externally sourced data, semantic search instead focuses on improving search accuracy by understanding the search query and the intent behind it.
Both can serve complementary purposes. Semantic search can improve the quality of RAG-based queries, as it focuses on gaining a deeper understanding of searches. This leads to RAG systems producing more accurate and meaningful outputs.
On its own, RAG is ideal for applications that need the most up-to-date information, while semantic search is ideal for applications where understanding user intent to improve search accuracy is paramount.
History of RAG
In the early 1970s, researchers started experimenting with and creating question-answering systems that could access text on specific topics. The process, which is known as text mining, analyzes large amounts of unstructured text and is aided by software that can identify data attributes such as concepts, patterns, topics and keywords. In the 1990s, Ask Jeeves -- a precursor to Google, now called Ask.com -- popularized question-answering systems.
Google's paper titled "Attention Is All You Need," published in 2017, introduced transformer architecture, marking a turning point in the ability to create and train both scalable and efficient LLMs. The following year, OpenAI's GPT was released, with GPT standing for Generative Pre-trained Transformer.
It wasn't until 2020 that RAG as a framework was introduced. A team with Facebook, now Meta, working at a London AI lab developed a way to condense more knowledge into the parameters of an LLM. They integrated a retrieval system with an LLM to enable the model to create more dynamic and grounded responses.
RAG continues to grow and evolve in its use. It's implemented in many major AI chatbots, including ChatGPT, for example.
Learn more about generative AI models, such as VAEs, GANs, diffusion, transformers and NeRFs.