What is a generative model?
A generative model uses artificial intelligence (AI) and statistical and probabilistic methods to create representations or abstractions of observed phenomena or target variables. These representations can then be used to generate new data similar to the observed data.
Generative modeling is used in unsupervised machine learning (ML) to describe phenomena in data, enabling computers to understand the real world. This AI understanding can be used to predict all manner of probabilities about a subject from modeled data.
Generative models are a class of statistical models that generate new data instances.
How generative models work
Generative models are generally run on neural networks. To create a generative model, a large data set is typically required. The model is trained by feeding it various examples from the data set and adjusting its parameters to better match the distribution of data.
Once the model is trained, it can be used to generate new data by sampling from the learned distribution. The generated data can be similar to the original data set but with some variations or noise. For example, a data set containing images of horses could be used to build a model that can generate a new image of a horse that has never existed but still looks almost realistic. This is possible because the model has learned the general rules that govern the appearance of a horse.
This article is part of
What is GenAI? Generative AI explained
Generative models can also be used in unsupervised learning to discover underlying patterns and structure in unlabeled data as well as many other applications, such as image generation, speech generation and data augmentation.
Types of generative models
The following are prominent types of generative models:
Generative adversarial network (GAN)
This model is based on ML and deep neural networks. In it, two unstable neural networks -- a generator and a discriminator -- compete against each other to provide more accurate predictions and realistic data.
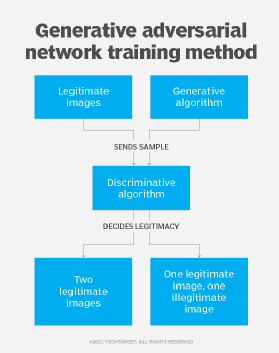
A GAN is an unsupervised learning technique that makes it possible to automatically find and learn different patterns in input data. One of its main uses is image-to-image translation, which can change daylight photos into nighttime photos. GANs are also used to create incredibly lifelike renderings of a variety of objects, people and scenes that are challenging for even a human brain to identify as fake.
Variational autoencoders (VAEs)
Similar to GANs, VAEs are generative models based on neural network autoencoders, which are composed of two separate neural networks -- encoders and decoders. They're the most efficient and practical method for developing generative models.
A Bayesian inference-based probabilistic graphical model, VAE seeks to understand the underlying probability distribution of the training data so that it can quickly sample new data from that distribution. In VAEs, the encoders aim to represent data more effectively, whereas the decoders regenerate the original data set more efficiently. Popular applications of VAEs include anomaly detection for predictive maintenance, signal processing and security analytics applications.
Autoregressive models
Autoregressive models predict future values based on historical values and can easily handle a variety of time-series patterns. These models predict the future values of a sequence based on a linear combination of the sequence's past values.
Autoregressive models are widely used in forecasting and time series analysis, such as stock prices and index values. Other use cases include modeling and forecasting weather patterns, forecasting demand for products using past sales data and studying health outcomes and crime rates.
Bayesian networks
Bayesian networks are graphical models that depict probabilistic relationships between variables. They excel in situations where understanding cause and effect is vital. For instance, in medical diagnostics, a Bayesian network can effectively assess the probability of a disease based on observed symptoms.
Diffusion models
Diffusion models create data by progressively introducing noise and then learning to reverse this process.
They're instrumental in understanding how phenomena evolve and are particularly useful for analyzing situations such as the spread of rumors in social networks or the transmission of infectious diseases within a population.
Restricted Boltzmann machines
RBMs are two-layered neural networks capable of learning the probability distribution of input data. They're used in recommendation systems, such as suggesting movies on streaming services based on user preferences.
Pixel recurrent neural networks
PixelRNNs are a type of generative model designed for image generation tasks. They're based on the concept of recurrent neural networks and are specifically trained to model images pixel by pixel, to generate new images that resemble the ones in the training data.
Markov chains
Markov chains are generative models that forecast future states based solely on the current state while ignoring any prior states. They're commonly used in text generation, where the next word in a sentence is predicted based only on the word currently in use.
Normalizing flows
These generative models transform a simple, easily sampled probability distribution, such as a Gaussian distribution, into a more complex distribution capable of modeling real-world data.
The primary purpose of normalizing flows is to apply a series of invertible transformations to a simple distribution so that after these transformations, the resulting distribution closely matches the target data distribution.
Generative models use cases
Generative models have a wide array of applications across various fields. Some notable use cases of generative models include the following:
- Image generation. GANs are widely used to create photorealistic images, which can be applied in industries including fashion, interior design and video game development. For instance, they can generate realistic human faces or design different elements for products.
- Art creation. Artists and musicians are increasingly using generative models to create new and innovative art pieces. Tools such as Midjourney have revolutionized the art world, enabling artists to generate stunning visuals based on text prompts or specific art styles.
- Drug discovery. Generative AI models are accelerating drug discovery by generating new molecular structures, predicting properties and optimizing them for traits such as efficacy and safety. This approach helps researchers explore a wider chemical space, reducing time and costs while improving the accuracy of identifying promising drug candidates.
- Content creation. Website owners are increasingly using generative models to streamline their content creation process by using tools such as HubSpot AI Content Writer and Copy.ai. For example, by providing prompts or topics, these AI-powered tools can generate blog posts, social media content, email marketing content and landing page copy. Content creation done by generative models speeds up the content creation process and helps maintain quality and consistency. However, it's important to remember that when creating content, human oversight is always needed to ensure accuracy, relevancy and a unique brand voice.
- Video games. Generative models accelerate video game development by automating various tasks, such as creating realistic textures, three-dimensional models and animations. The models generate diverse game worlds and levels, as well as intelligent non-player characters, or NPCs, with dynamic behaviors. By automating repetitive processes and generating creative content, generative models enable developers to focus on core gameplay, resulting in more immersive and innovative gaming experiences.
- Image-to-image translation. Generative models provide image-to-image translation by learning to map different image representations together through deep learning techniques. For instance, models such as GANs can transform a grayscale image into a colored version or convert rough sketches into realistic images.
- Text-to-image translation. Generative models can convert textual descriptions into corresponding images, providing applications in advertising and content creation. The text-to-image technology visualizes concepts that are described in words.
- Video generation. Generative models, such as GANs, create synthetic video content by learning patterns from existing video data. They analyze frame relationships, motion and visual elements to generate new sequences that mimic the original style. This capability supports applications in entertainment, advertising and virtual reality, where dynamic, engaging content is crucial.
- Audio generation. Generative models have significantly advanced the fields of speech synthesis and music composition. For instance, models including WaveNet and Tacotron use deep learning techniques to generate highly natural and expressive synthetic speech, which provide applications in virtual assistants, audiobooks and voice-overs. In the music realm, models such as Musenet and Jukedeck employ machine-learning algorithms to compose original music by analyzing extensive data sets of existing songs. This technology aids musicians in their creative endeavors and offers background scores for various multimedia projects.
Generative modeling vs. discriminative modeling
Machine learning models are typically classified into discriminative and generative models. Both serve different purposes in ML, each with a unique approach to understanding data.
Generative modeling contrasts with discriminative modeling, which identifies existing data and can be used to classify data. Generative modeling produces something, whereas discriminative modeling captures the conditional probability, recognizes tags and sorts data. A generative model can be enhanced by a discriminative model and vice versa. This is done by having the generative model try to fool the discriminative model into believing the generated images are real. Through successions of training, both become more sophisticated at their tasks.
The following is a brief rundown of major differences between the two models:
- Generative models are used in unsupervised ML problems, whereas discriminative models are used for supervised learning.
- When given an input, discriminative models estimate the likelihood of a particular class label. In contrast, generative models produce fresh data samples that are similar to the training data. Simply put, discriminative models concentrate on label prediction, whereas generative models concentrate on modeling the distribution of data points in a data set.
- Generative models are typically more flexible than discriminative models in expressing dependencies in complex learning tasks, but they can be more computationally expensive and could require more data to prevent overfitting. On the other hand, discriminative models are simpler and easier to train, and they typically outperform generative models when there's a distinct boundary between classes.
- Compared to discriminative models, generative models might be less accurate even though they use less data to train, since they're more biased due to the higher assumptions they make. The low accuracy levels also stem from the fact that generative models need to learn about the distribution of data, whereas discriminative models only need to learn about the relationship between inputs and outputs.
Benefits of generative models
Generative models offer the following advantages, which make them valuable in various applications:
- Data augmentation. Generative models can augment data sets by creating synthetic data, which is valuable when real-world labeled data is scarce. This improves the training of other ML models.
- Data distribution. Generative models provide insights into the underlying distribution of the data. By modeling how data is generated, they can help researchers and practitioners understand the relationships and dependencies within the data, leading to better decision-making and analysis.
- Anomaly detection. Generative models detect anomalies by learning the distribution of normal data during the training process. They generate new data points based on this distribution and flag any significant deviations as anomalies. This approach effectively identifies unusual events without needing labeled anomaly examples, making it useful for fraud detection and equipment monitoring applications.
- Flexibility. Generative models can be applied to various learning scenarios, such as unsupervised, semi-supervised and supervised learning, making them adaptable to a wide range of tasks.
- Cost optimization. Generative models reduce manual production and research costs across industries by automating content creation. For example, in manufacturing, generative models optimize designs, simulate production processes and predict maintenance needs, which cuts down on time, resources and operational costs.
- Handling of missing data. Generative models are effective in handling incomplete data sets by inferring missing values based on the learned distribution, enhancing analyses and predictions.
Challenges of generative models
Generative models provide several advantages, but they also have the following drawbacks:
- Computational requirements. Generative AI systems often require a large amount of data and computational power, which some organizations might find prohibitively expensive and time-consuming.
- Quality of generated outputs. Generated outputs from generative models might not always be accurate or free of errors. This could be caused by several things, including a shortage of data, inadequate training or an overly complicated model.
- Lack of interpretability. It might be challenging to comprehend how predictions are being made by generative AI models, as these models can be opaque and complicated. Ensuring the model is making impartial and fair decisions can be challenging at times.
- Overfitting. Overfitting can occur in generative models, resulting in poor generalization performance and incorrectly generated samples. It happens when a model is unable to generalize and instead fits too closely to the training data set. This can happen for a variety of reasons, including the training data set being too small and lacking enough data samples to adequately represent all potential input data values.
- Security. Generative AI systems can be used to disseminate false information or propaganda by generating realistic and convincing fake videos, images and text.
- Black box nature. Generative models, especially those based on deep learning, often operate as black boxes, making it difficult to understand their decision-making processes. This lack of interpretability can hinder trust and adoption in critical applications, such as healthcare or finance, where understanding the rationale behind generated outputs is crucial.
- Mode collapse. Mode collapse occurs when a generative model, such as a GAN, fails to capture the full diversity of the training data. Instead, it becomes stuck generating a limited set of similar outputs, often referred to as modes. This can lead to a lack of variety and creativity in the generated content.
Deep generative modeling
A subset of generative modeling, deep generative modeling uses deep neural networks to learn the underlying distribution of data. These models can develop novel samples that have never been seen before by producing new samples that are similar to the input data but not the same. Deep generative models come in many forms, including VAEs, GANs and autoregressive models. These models have proven promising in a wide range of applications, including text-to-image synthesis, music generation and drug discovery.
However, deep generative modeling remains an active area of research with many challenges. These include difficulties evaluating the quality of generated samples and preventing mode collapse, which can occur when the generator starts producing similar or identical samples, leading to a collapse in the modes of data distribution.
Large-scale deep generative models are increasingly popular. For example, BigGAN and VQ-VAE are used to generate images and can have hundreds of millions of parameters. Jukebox is another large generative model for musical audio that has billions of parameters. OpenAI's third-generation Generative Pre-trained Transformer (GPT-3) and its predecessors, which are autoregressive neural language models, also contain billions of parameters. But GPT-4o outshines all the previous versions of GPT in terms of dependability, originality and the capacity to comprehend complex instructions. It can process up to 64,000 tokens, enabling it to handle more complex prompts.
GPT-5 is expected to be released in 2025. Its training data is anticipated to be both extensive and diverse, combining around 70 trillion tokens across 281 terabytes of data.
Generative modeling history and timeline
Generative models have been a mainstay of AI since the 1950s. Early models at the time, including Hidden Markov models and Gaussian mixture models, provided simple data. However, the field has experienced a significant rise in popularity in recent years, thanks to the development of powerful generative models such as GANs and VAEs.
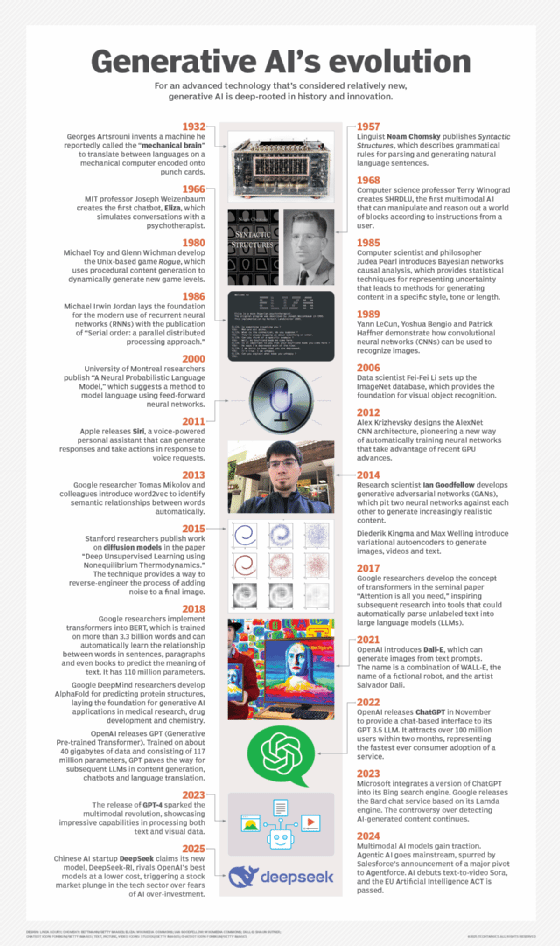
Ian Goodfellow first proposed GANs in 2014, as well as the two-part generator and discriminator architecture. The generator creates new data, while the discriminator tries to distinguish between the generated data and real data. The generator learns to improve its output by attempting to fool the discriminator.
In 2017, the transformer -- a deep learning architecture that underpins large language models including GPT-3, Google LaMDA and DeepMind Gopher -- was introduced. The transformer can generate text, computer code and even protein structures.
In 2021, OpenAI introduced a technique called Contrastive Language-Image Pre-training (CLIP) that's used heavily by text-to-image generators. Using image-caption pairs gathered from the internet, CLIP is particularly successful at discovering shared embeddings between images and text.
Since CLIP's release, multiple vision-language algorithms have emerged, including MetaAI's MetaCLIP, PubmedCLIP for medical and visual question and answering, and BioCLIP for classifying items by their biological taxonomy.
Recent AI generative services are aiding generative AI's quick and unparalleled rise to fame. Examples include OpenAI's Dall-E and ChatGPT.
The release of GPT-4 and GPT-4 Vision in 2023 ignited the multimodal revolution, demonstrating remarkable capabilities in processing text and visual data and elevating multimodal AI to new heights by enabling even more sophisticated and realistic interactions. This rapid progress, along with the 2024 release of the latest version, GPT-4o, has solidified multimodal AI and large multimodal models as some of the most prominent trends in generative AI for 2024.
These models have been applied in various fields, such as computer vision, natural language processing and music generation. Generative modeling has also seen advancements in quantum machine learning and reinforcement learning. In general, the rise of generative modeling has opened up many new possibilities for AI and has the potential to transform a wide range of industries, from entertainment to healthcare.
GANs and VAEs are two popular generative AI approaches. Analyze the benefits and drawbacks of each method and discover how GANs and VAEs stack up against each other.