cognitive bias
What is cognitive bias?
Cognitive bias is a systematic thought process caused by the tendency of the human brain to simplify information processing through a filter of personal experience and preferences. The filtering process is a coping mechanism that enables the brain to prioritize and process large amounts of information quickly. While the mechanism is effective, its limitations can cause errors in thought.
Essentially, cognitive biases help humans find mental shortcuts to assist in the navigation of daily life, but may often cause irrational interpretations and judgments.
Cognitive biases often stem from problems related to memory, attention and other mental mistakes. They're often unconscious decision-making processes that make it easy for individuals to be affected without intentionally realizing it. The filtering process and coping mechanism used to process large amounts of information quickly is called heuristics.
Cognitive bias as a concept was first introduced by Amos Tversky and Daniel Kahneman in 1972. It may not be possible to completely eliminate the brain's predisposition to taking shortcuts but understanding that biases exist can be useful when making decisions.
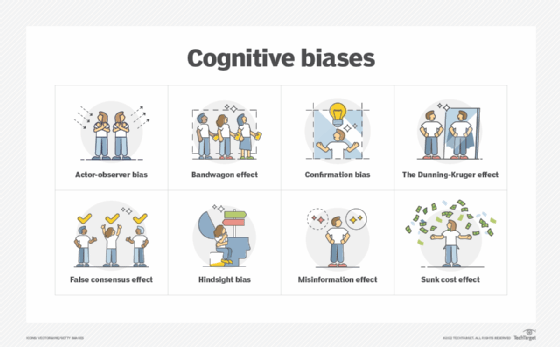
Types of cognitive bias
A continually evolving list of cognitive biases has been identified over the last six decades of research on human judgment and decision-making in cognitive psychology, social psychology and behavioral economics. They include the following:
- Actor-observer bias. The tendency for an individual to credit their own situation to external causes while ascribing other people's behaviors to internal causes.
- Anchoring bias. The tendency for the brain to rely too much on the first information it received when making decisions.
- Attentional bias. The tendency for an individual to pay attention to a single object or idea while deviating from others.
- Availability bias. The tendency for the brain to conclude that a known instance is more representative of the whole than is actually the case.
- Availability heuristic. The tendency to use information that comes to the mind quickly when making decisions based on the future.
- Bandwagon effect. The tendency for the brain to conclude that something must be desirable because other people desire it.
- Bias blind spot. The tendency for the brain to recognize another's bias but not its own.
- Clustering illusion. The tendency for the brain to want to see a pattern in what is actually a random sequence of numbers or events.
- Confirmation bias. The tendency for the brain to value new information that supports existing ideas.
- The Dunning-Kruger effect. The tendency for an individual with limited knowledge or competence in a given field to overestimate their own skills in that field.
- False consensus effect. The tendency for an individual to overestimate how much other people agree with them.
- Framing effect. The tendency for the brain to arrive at different conclusions when reviewing the same information, depending on how the information is presented.
- Functional fixedness. The tendency to see objects as only being used in one specific way.
- Group think. The tendency for the brain to place value on consensus.
- Halo effect. The tendency for a person's impression in one area to influence an opinion in another area.
- Hindsight bias. The tendency to interpret past events as more predictable than they actually were.
- Misinformation effect. The tendency for information that appears after an event to interfere with the memory of an original event.
- Negativity bias. The tendency for the brain to subconsciously place more significance on negative events than positive ones.
- Proximity bias. Proximity bias is the subconscious tendency to give preferential treatment to people that are physically close. A physical worker being considered for a raise before a remote worker because they are in the immediate vicinity of their superior is an example of proximity bias.
- Recency bias. The tendency for the brain to subconsciously place more value on the last information it received about a topic.
- Self-serving bias. The tendency for an individual to blame external forces when bad events happen but give themselves credit when good events happen.
- Sunk cost effect. Also called the sunk cost fallacy, this is the tendency for the brain to continue investing in something that clearly isn't working in order to avoid failure.
- Survivorship bias. The tendency for the brain to focus on positive outcomes in favor of negative ones. A related phenomenon is the ostrich effect, in which people metaphorically bury their heads in the sand to avoid bad news.
Signs and effects of bias
Because cognitive bias is often an unconscious process, it's easier for an individual to recognize a bias in someone other than themself. However, some ways to recognize bias include the following:
- if an individual attributes a success to themselves, while attributing other's successes to luck;
- if an individual assumes they have more knowledge than they actually have on a topic;
- if an individual insists on blaming outside factors instead of themself;
- if an individual is only paying attention to what confirms their opinions; and
- if an individual assumes everyone shares their own opinions.
Individuals should try their best to stay away from these signs, as they all affect -- at a base rate -- how that person interprets the world around them. Even if an individual is objective, logical and can accurately evaluate their surroundings, they still should be wary of adapting any new unconscious cognitive biases.
Impact of cognitive bias on software development data analytics
Cognitive biases can affect individuals by changing their world views or influencing their work. For example, in the technical field, cognitive biases can affect software data analytics and machine learning models.
Being aware of how human bias can obscure analytics is an important first step toward preventing it from happening. While data analytics tools can help business executives make data-driven decisions, it's still up to humans to select what data should be analyzed. This is why it's important for business managers to understand that cognitive biases that occur when selecting data can cause digital tools used in predictive analytics and prescriptive analytics to generate false results.
As an example, data analysts could use predictive modeling without first examining data for bias, which could lead to unexpected results. These biases could also influence developer actions. Some examples of cognitive bias that can inadvertently affect algorithms are stereotyping, the bandwagon effect, priming, selective perception and confirmation bias.
Cognitive bias can also lead to machine learning bias, which is a phenomenon that occurs when an algorithm produces systemically prejudiced results due to assumptions made in the machine learning process. Machine learning bias often originates from poor or incomplete data that results in inaccurate predictions. Bias may also be introduced by individuals who design and train the machine learning systems, as these algorithms can reflect the unintended cognitive biases of the developers. Some example types of biases that affect machine learning include prejudice bias, algorithm bias, sample bias, measurement bias and exclusion bias.
Tips for overcoming and preventing bias
To prevent cognitive bias and other types of bias caused by it, organizations should check the data being used to train machine learning models for lack of comprehensiveness.
Data scientists developing the algorithms should shape data samples in a way that minimizes algorithmic and other types of machine learning bias, and decision-makers should evaluate when it is appropriate, or inappropriate, to apply machine learning technology. Data revolving around people should be representative of different races, genders, backgrounds and cultures that could be adversely affected.
Awareness and good governance are two main ways that can help prevent machine learning bias. This means the following actions should be taken:
- Appropriate training data should be selected.
- Systems should be tested and validated to ensure the results don't reflect bias.
- Systems should be monitored as they perform their tasks.
- Additional tools and resources should be used, such as Google's What-If Tool or IBM's AI Fairness 360 open source toolkit.
Organizations can mitigate for bias using both automated and human action, but they may not be able to remove it all. But not all bias is inherently damaging. Algorithmic bias, for example, is the process an algorithm uses to determine how to sort and organize data. It's important to ensure that the type of bias isn't inherently harmful and makes sense for the task at hand.
Learn more about bias in artificial intelligence and the key trends that exist in this article.