What is AI art and how is it created?
Artificial intelligence art (AI art) is any form of art that has been created or enhanced with AI tools. Although commonly associated with visual art, such as images or videos, the term AI art also applies to music, writing and other creative forms.
Tools for creating AI art use machine learning algorithms trained with digitized artwork data and their associated descriptive data. This enables the algorithms to learn what art is and how to describe it. AI art tools also leverage other advanced technologies, such as neural networks and generative adversarial networks (GANs). Together these technologies make it possible to change or enhance existing images or generate new images based on the submitted text prompts.
AI art challenges the millennia-old paradigm of humans as the sole creators of art. Its introduction raises questions about the genesis of creativity and carries ethical and legal concerns. AI art also presents an opportunity to extend the boundaries of art and creativity in multiple ways.
AI art can enable almost anyone to create digital art in a fraction of the time it would take compared to other methods. In addition, AI art can generate visual or audio works that would be difficult to create otherwise. With text-to-image generative AI tools, such as Dall-E or Stable Diffusion, users can generate the images they want without a high degree of technical skill. They simply type (or speak) a descriptive text prompt, and the tool generates the desired image.
This article is part of
What is GenAI? Generative AI explained
How does AI art work?
AI art tools use a variety of models and techniques to generate images although most are based on the same underlying technologies. For example, AI art tools make extensive use of machine learning algorithms to uncover complex patterns in the collected data. An algorithm is fed the data it needs to train the AI model, which then makes it possible to generate accurate and realistic images. The data itself is an extensive collection of digital images and their descriptive information.
Once the model has been trained, a user can submit a text prompt to the AI platform. The prompt is incorporated into the generation process to ensure accuracy and relevancy. Modern AI art tools such as ChatGPT and Microsoft Copilot provide an interface for users to easily submit text prompts directly to the platform. However, the prompts must carefully describe the types of images to generate. The more precise the prompts, the better the images. To support this process, the AI tools typically use some form of natural language processing (NLP) to understand and interpret the prompts.
AI art platforms use different types of models, including the following:
- Generative adversarial network. GAN uses deep learning and multiple neural networks to generate images that resemble the training data, while taking into account the user's prompt.
- Convolutional neural network. With the CNN approach, the deep learning model identifies objects, which can then be used to generate new images.
- Neural style transfer. An NST is used in conjunction with a CNN as a deep learning technique that enables the style of one image to be transferred to another. For example, a user might use an NST to generate an image in the style of Van Gogh.
- Recurrent neural network. An RNN generates sequences of data, such as those used in music. The RNN uses a feedback loop to produce a sequence of outputs based on prior inputs. This enables the RNN to generate new outputs that resemble the inputs on which they were trained.
How difficult is it to make AI art?
Making AI art has become increasingly easier for all types of creators, regardless of their skill levels or experience with AI tools.
At the most advanced level, artists can train their own AI models based on the type of art they want to create. In this approach, the artist first needs to collect or have access to a data set that contains both the digitized art pieces and their descriptions. Once the data has been collected, the artist can train the AI model, using a model that is suited to generating art, such as GAN.
Rather than training AI models themselves, artists can use one of the multiple AI art tools now available. These tools are easy to use because the models have already been trained on a data set of existing art. Depending on the tool, it might be possible for artists to add their own sets of images to further refine the model.
After a model has been trained, the artist can then generate images by submitting text prompts to the AI platform. Some platforms let users further refine their images by submitting subsequent prompts within the same session as the original prompt. A platform might also provide artists with visual design tools to fine-tune their creations.
A number of AI image generator tools are available for creating art, including the following:
- Adobe Firefly.
- Artbreeder.
- Dall-E.
- Deep Dream Generator.
- DreamStudio.
- Midjourney.
- Playform.
- Stable Diffusion.
Many AI art tools offer new users free credits or free trials to try them out. Other services, such as ChatGPT and Copilot, offer a free tier that permits image generation within a limited scope.
How are artists using AI?
In the past, an artist's primary tools were brushes, paints, chisels, typewriters, musical instruments or other physical devices. AI art offers a new type of tool for creating art, one that could benefit a variety of use cases:
- Art therapy. Many people create art for personal enjoyment and relaxation. Therapists can also use AI art for occupational therapy and other types of therapies.
- Education. Educators and teachers can use AI art tools to help teach a new generation of artists. AI art can also be used to generate images for use in their classrooms to help explain complex concepts.
- Art creation. AI tools help artists create entirely new pieces of visual art, videos and music.
- Art enhancement. Artists can use AI tools to enhance, augment and improve their creations.
- Art inspiration. AI tools provide artists with starting points that can offer new perspectives, prompt innovation and inspire new ideas or styles.
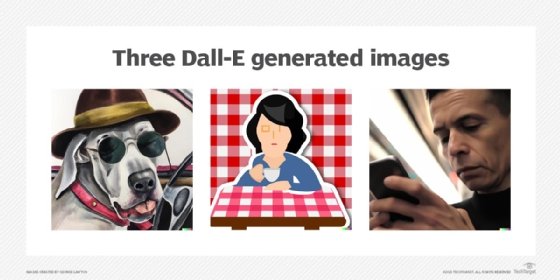
Why is AI art controversial?
AI art platforms offer artists multiple opportunities for creating art. However, AI art also comes with a number of concerns:
- Authorship. Artists have long enjoyed the practice of signing their names to their works of art. But how does authorship work with AI art? An emerging ethical concern questions who actually created the work: the AI platform, the artists behind the source artwork used to train the data, or the user who submitted the prompt to the platform?
- Bias. An AI model is only as diverse and representative as the data on which it was trained. Bias can show up in the generated images if the data itself is biased toward or against a particular group of people.
- Copyright. A major concern is the potential for intellectual property theft. For example, some large GAN-based AI platforms use copyrighted data when training their models, which has resulted in multiple lawsuits. Getty Images, for example, filed a lawsuit in January 2023 against Stable Diffusion for alleged infringement on copyrights held by Getty.
- Originality. While defining what constitutes art has long been the subject of debate, one common attribute is that art is, in some way, original. With AI art there is an ethical question about whether generated works are genuinely original or just derivative.
History of AI-generated art
One of the earliest attempts at AI art was the Aaron system, which was introduced in 1973. Developed by Harold Cohen, Aaron was an AI assistant that used a symbolic AI approach to create black-and-white art drawings. However, progress in AI-generated art didn't begin in earnest until 2014, when GANs were first introduced. GANs also provided a foundation for generative AI technologies.
In 2015, Google released DeepDream, which used a convolutional neural network as an experimental approach to AI art, further advancing the field. Three years later, in 2018, Ganbreeder was launched and later rebranded as Artbreeder. It incorporated GAN models that enabled humans to use AI to modify existing images and create new ones.
The same year, the Obvious artist collective used GAM models to create a painting named Edmond de Belamy. The GAN models were trained on a corpus of 15,000 portraits from the 14th century through the 19th. The artwork was publicly available on the WikiArt website. The collective eventually sold the painting at Christie's auction house for $432,500.
In January 2021, OpenAI launched Dall-E, the first major GAN-based text-to-image generation tool. Dall-E offered a publicly accessible and usable system that enabled anyone with internet access to use text prompts to create AI art, giving the world a glimpse into the potential of AI art.

In May 2022, Google announced its Imagen text-to-image technology as another option for AI art. This was followed in August 2022 by Stability AI, which launched Stable Diffusion services, another GAN-based, publicly accessible tool for creating AI art with text prompts.
The growth of AI art tools continued in 2023, with large software vendors joining the market. A notable example is the Adobe Firefly service, which was announced in March 2023. Adobe integrated the GAN-based approach into its popular image and video editing tools, including Photoshop and Premier.
Explore the pros and cons of AI-generated content, AI content generators and AI and machine learning trends. Learn why creative workers say livelihoods are threatened by generative AI and how AI will affect the future of content marketing.