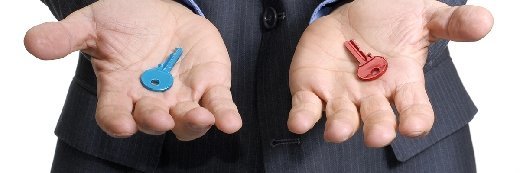
gosphotodesign - Fotolia
Data scientist vs. business analyst: What's the difference?
Data science and business analyst roles differ in that data scientists must deep dive into data and come up with unique business solutions -- but the distinctions don't end there.
What is the difference between data science and business analyst jobs? And what kind of training or education is required to become a data scientist?
There are a number of differences between data scientists and business analysts, the two most common business analytics roles, but at a high level, you can think about the distinction as similar to a medical researcher and a lab technician. One uses experimentation and the scientific method to search out new, potentially groundbreaking discoveries, while the other applies existing knowledge in an operational context.
Data scientist vs. business analyst comes down to the realms they inhabit. Data scientists delve into big data sets and use experimentation to discover new insights in data. Business analysts, on the other hand, typically use self-service analytics tools to review curated data sets, build reports and data visualizations, and report targeted findings -- things like revenue by quarter or sales needed to hit targets.
What does a data scientist do?
A data scientist takes analytics and data warehousing programs to the next level: What does the data really say about the company, and is the company able to decipher relevant data from irrelevant data?
A data scientist should be able to leverage the enterprise data warehouse to dive deeper into the data that comes out or to analyze new types of data stored in Hadoop clusters and other big data systems. A data scientist doesn't just report on data like a classic business analyst does, he also delivers business insights based on the data.
A data scientist job also requires a strong business sense and the ability to communicate data-driven conclusions to business stakeholders. Strong data scientists don't just address business problems, they'll also pinpoint the problems that have the most value to the organization. A data scientist plays a more strategic role within an organization.
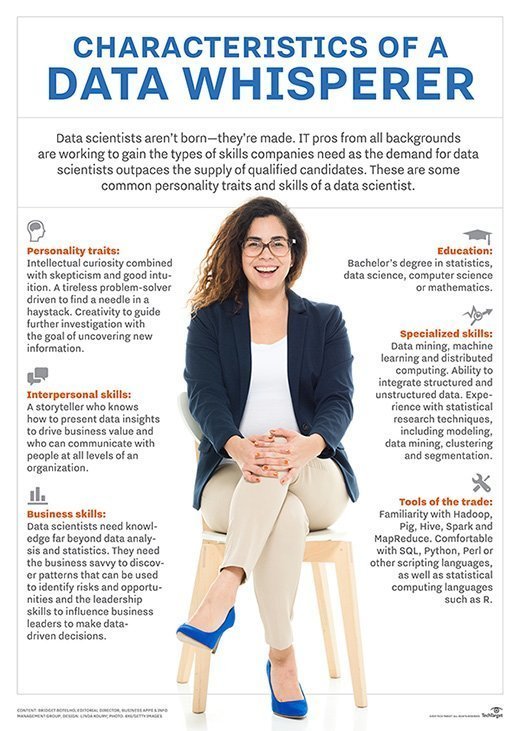
Data scientists look through all the available data with the goal of discovering a previously hidden insight that, in turn, can provide a competitive advantage or address a pressing business problem. Data scientists do not simply collect and report on data -- they also look at it from many angles, determine what it means and then recommend ways to apply the data. These insights could lead to a new product or even an entirely new business model.
Data scientists apply advanced machine learning models to automate processes that previously took too long or were inefficient. They use data processing and programming tools -- often open source, like Python, R and TensorFlow -- to develop new data science applications that take advantage of advances in artificial intelligence. These applications may perform a task such as transcribing calls to a customer service line using natural language processing or automatically generating text for email campaigns.
What does a business analyst do?
A business analyst -- a title often used interchangeably with data analyst -- focuses more on delivering operational insights to lines of business using smaller, more targeted data sets. For example, a business analyst tied to a sales team will work primarily with sales data to see how individual team members are performing, to identify members who might need extra coaching and to search for other areas where the team can improve on its performance.
Business analysts typically use self-service analytics and data visualization tools. Using these tools, business analysts can build reports and dashboards that team members can use to track their performance. Typically, the information contained in these reports is retrospective rather than predictive.
Data scientist vs. business analyst training, tools and trends
To become a business analyst, you need a familiarity with statistics and the basic fundamentals of data analysis, but there are many self-service analytics tools that do the mathematical heavy lifting for you. Of course, you have to know if it's statistically meaningful to join two separate data sets, and you have to understand the distinction between correlation and causation. But, on the whole, a deep background in mathematics is unnecessary.
To become a data scientist, on the other hand, you need a strong background in math. This is one of the primary differences in the question of data scientists vs. business analysts.
Many data scientists have doctorates in some field of math. Many have backgrounds in physics or other advanced sciences that lean heavily on statistical inference.
Business analysts can generally pick up the technical skills they need on the job. Whether an enterprise uses Tableau, Qlik or Power BI -- the three most common self-service analytics platforms -- or another tool, most use graphical user interfaces that are designed to be intuitive and easy to pick up.
Data science jobs require more specific technical training. In addition to advanced mathematical education, data scientists need deep technical skills. They must be proficient in several common coding languages -- including Python, SQL and Java -- which enable them to run complex machine learning models against big data stored in Hadoop or other distributed data management platforms. Most often, data scientists pick up these skills from a college-level computer science curriculum.
However, trends in data analytics are beginning to collapse the line between data science and data analysis. Increasingly, software companies are introducing platforms that can automate complex tasks using machine learning. At the same time, self-service software supports deeper analytical functionality, meaning data scientists are increasingly using tools that were once solely for business analysts.
Companies often report the highest analytics success when blending teams, so data scientists working alongside business analysts can produce operational benefits. This means that the data scientist vs. business analyst distinctions could become less important as time goes on -- a trend that may pay off for enterprises.