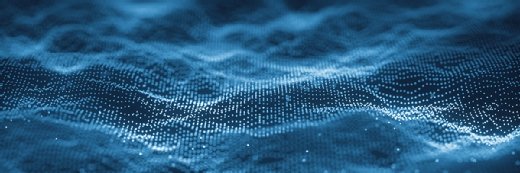
Nabugu - stock.adobe.com
Where does AI fit into a risk assessment strategy?
AI is a helpful way to take a different look at risk assessment and disaster recovery. AI sifts through massive amounts of risk data without the typical constraints of DR teams.
Risks come in all shapes, sizes and forms. These can include environmental, technical, geographical and financial, to name a few. Sometimes risk analysis can be so complex that humans have trouble conceptualizing and modeling potential issues. This misunderstanding can become costly.
That is where AI comes in. Organizations can use AI to find potential scenarios and issues with disaster recovery planning and execution that DR teams may not have considered. It can help users understand constraints that are not always visible, even under component testing.
Using all this intelligence is not easy. Organizations must sift through massive amounts of data to get anything useful out of it. When conducting risk assessments, DR teams must consider the severity and likelihood of events that have not even happened yet. The large amounts of data they must work with is one example of where AI can help. Before jumping in headfirst, however, there are several things that businesses must consider.
AI is not a magic tool to fix everything. However, with quality data sets and a firm understanding of where AI works best, disaster recovery teams have a powerful risk assessment tool on their hands.
Where AI fits in risk assessment
Insurance is one field where AI conceptualization and optimization is already being used. Some insurance companies use simulations, with virtual disasters ready to strike at the click of a mouse.
AI gives the insurance underwriters an idea of potential costs incurred when a disaster strikes, along with the likelihood of disasters occurring. Massive data sets and AI enable the insurance companies to map out disaster scenarios with detailed potential risk profiles for customers.
The actual use of the AI and data can be split into several different types. First is analytics, where the organization uses the data it acquires to find and report patterns.
An alternative use of AI is when the AI can be autonomous, working independently or in conjunction with human oversight and input. This is what a lot of people think of when they think of AI. Again, it's all about data and quality. Enabling these products to learn how humans react in a specific DR implementation lets the AI create optimized strategies to streamline DR.
Streamlined but not perfected
Some organizations use AI to decide how to approach DR when there are dozens of applications they have to restore. When humans prioritize applications, they may limit themselves to following a typical DR playbook to restoration. By contrast, AI is not subject to these same constraints and may be able to restore applications faster.
AI is not a silver bullet, and organizations must fully research any company that offers "magic" AI tools to ensure that they understand the capabilities and limitations of the product. Just because it mentions AI, it does not mean it will do things better than they are done presently.
Ensure a good quality of data, combined with people who have proper understanding of working with and examining data with a range of industry-standard AI tools. This will help DR teams find unconsidered risks and outcomes and prepare the organization accordingly.