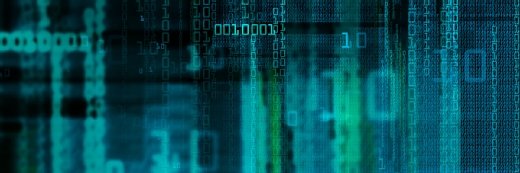
Getty Images
Top 6 data fabric tools providers
Organizations that want a data fabric architecture should consider how six top vendors use their suite of tools to support a range of data management, automation and AI capabilities.
To build a data fabric, an organization needs multiple tools and products. Data management vendors offer different combinations of capabilities from their toolboxes to support a data fabric architecture with data management, governance, AI and machine learning.
A data fabric is an architectural concept for creating a data virtualization layer across an organization. Fabrics can help reduce data silos, simplify access across users and apps, and facilitate the reuse of data in different ways.
Building a working data fabric involves a combination of tools, including data ingestion, virtualization, data governance and security tools, as well as cloud computing. These help with integration, ontology and semantics, data quality, and data transformation. A variety of data management tools support some or many of these capabilities.
The combination of tools forms the foundation of a data fabric. Technologies such as knowledge graphs are gaining popularity for managing and integrating data within the data fabric. Knowledge graphs use semantic relationships to enable meaningful data search and querying. Knowledge graphs provide users with valuable insights into the history and context of the data they are working with by tracing data lineage.
A data mesh is a competitive alternative to a data fabric that focuses on data products rather than infrastructure. It is also supported by popular data fabric tools.
Top features for data fabrics
Real-time analytics and recommendation engines enable organizations to extract insights precisely and effortlessly from their data. The capabilities also enhance human actions by providing recommendations and insights.
AI/machine learning capabilities help extract insights from vast amounts of structured and unstructured data sets.
Scalability helps businesses keep up with growing volumes and third-party data.
Data management is essential for organizing large volumes of data and making it available to users. Key processes include indexing, searching and querying.
Security and privacy are paramount for organizations handling sensitive information. Data fabric tools should offer strong security protocols to safeguard sensitive data from unauthorized access.
Challenges to consider
Implementing a working and cost-effective data fabric presents several challenges. Decision-makers must consider all potential problems that specific tools might have so they can ask the right questions of their technical staff and vendors during the decision-making process. Key challenges to consider include the following:
- Integration challenges. Incorporating data from diverse sources and platforms can be complex, particularly when dealing with varying formats or structures. Integrating a data fabric into an existing infrastructure can also pose difficulties.
- Complexity. Implementing a data fabric can be complex. It requires high expertise and the right resources to set up and manage, which is challenging for organizations with limited IT and data skill sets.
- Data security. Implementing a data fabric requires organizations to possess considerable proficiency in data security. Organizations must guarantee that data remains protected from unauthorized access and potential security threats.
- Existing tools. Organizations might encounter challenges integrating a data fabric with current data management tools and platforms, which could restrict the fabric's overall efficacy.
6 top data fabric tools
Following are six of the top data fabric tools and services to consider based on research from sources including the Everest Group, Forrester and Gartner. The tools in this unranked list have the essential capabilities that organizations might need to deploy data fabrics, but vendors do not specifically list them as data fabric offerings. The list is in alphabetical order.
Denodo
Denodo's core offering is the Denodo Platform, which features cloud and express variants tuned for various use cases. The company has a long history in data virtualization tools, and has developed an extensive partner and integration ecosystem since its founding in 1999. Key strengths include various deployment options, data transformation and data processing tools.
Denodo supports a variety of semantic and schema management capabilities for working with various data models, generating data catalogs, discovering data assets, classification and data quality management. In addition to data fabrics, it also has uses in data mesh architectures. It's a good fit for large-scale enterprise deployments and can support a variety of uses, including analytics, data science and AI workloads. Another plus is its FinOps capabilities that help guide financial decisions in cost optimization for data infrastructure provision. The launch of Denodo Platform 9 in June 2024 brought improved AI integration, better self-service, and support for natural language queries and AI-ready data.
Denodo offers four pricing tiers: professional, standard, enterprise and enterprise plus. Prices are not publicly available, but Denodo offers a free 30-day trial of the professional version.
IBM
IBM has a solid base of data integration, quality, master data management, governance and security tools. It gradually expanded this core foundation to support cloud and multi-cloud scenarios. Key strengths include tools for data discovery, data models and data classification. For example, the IBM Cloud Pak for Data consists of various supporting elements, such as DataStage Enterprise Plus Cartridge, Watson Knowledge Catalog and other data virtualization capabilities.
IBM made several data fabric-related acquisitions over the past couple years including Databand for data pipeline observability in 2022, Manta for data lineage and StepZen for GraphQL support in 2023. It finalized the acquisition of the StreamSets and webMethods platforms from Software AG in July 2024 to further build on current tools with more dynamic and composable integration capabilities.
Some key differentiators IBM has from competitors include support for tools to facilitate better management of data fabric infrastructure after deployment. For example, schema drift capabilities notify data users of changes. It can also automatically update metadata on the fly and reflect changes to all users.
IBM's portfolio of tools has grown through acquisitions and internal development. It's important to identify and prioritize the best fit for an organization's needs. Pricing varies based on the tool.
Informatica
Informatica's data fabric reference architecture places the semantic layer at the top. It facilitates connections to data governance, data catalog, data orchestration and various AI, machine learning and knowledge graph integrations. The semantic layer also organizes interactions with data prep and data marketplace tools. Its Claire AI service can continuously analyze new data to populate the semantic layer automatically. It can also use cost data optimization processes to manage the costs associated with a large data footprint efficiently.
Informatica excels at support for knowledge graphs, process automation, and democratizing AI and data processes. Beyond the pure data fabric side of things, it has built a solid foundation of tools for data transformations, discovery, classification and integration. Relevant data fabric tools and services include Intelligent Data Management Cloud, PowerCenter Cloud Modernization, Platform Trust, Modern Data Center Architecture, Cloud Connectivity, Data Catalog and Cloud Data Marketplace.
Informatica uses a pay-as-you-go licensing model that simplifies licensing considerations around a large collection of tools. Informatica is a good choice for organizations looking for a comprehensive product baked in a solid semantics layer to guide their data fabric journey.
Oracle
Oracle was one of the original upstarts to IBM's legacy database franchise. Oracle built a solid foundation of data management, integration and governance tools ideally suited for data fabric architectures. One key advantage is its globally distributed transaction architecture that helps integrate data into a common data fabric. It streamlines the process of distilling and processing information for operational and analytics purposes.
Oracle invested in generative AI to improve search and queries and automate data pipelines. Future work promises improved knowledge graphs, self-service and support for more data sources. Oracle achieved strong performance with its monolithic on-premises data stack, but also embraced cloud opportunities with a new line of data fabric tools supporting high performance and flexibility. It's a good fit for large organizations with a multinational footprint seeking to flesh out their data fabric strategy. Oracles offers usage-based pricing for each tool.
Qlik
Qlik, founded in 1993, was an early pioneer in interactive visualization technology. Data pipeline support encompasses various data fabric capabilities. Tools and services include Qlik Application Automation, Qlik Catalog, Qlik Cloud, Qlik Compose, Qlik Enterprise Manager and Qlik Replicate. In May 2023, Qlik acquired Talend to build out its tool ecosystem. The company has a strong presence in the financial services, healthcare, manufacturing and retail industries.
Qlik uses its roster of tools to differentiate itself from competitors. Qlik Compose helps automate data ingestion and ETL pipelines. Qlik AutoML helps automatically update schema adaptation using version AI techniques. Qlik has also developed advanced capabilities for democratizing data efforts through natural language prompts. It's a good fit for organizations with a large cloud footprint looking for a data fabric that combines analytics.
SAP
SAP methodically upgraded its ERP software to embrace data fabric opportunities. It built integration infrastructure to facilitate the use of data across a coherent data fabric. SAP Datasphere incorporates operational data through a strong metadata layer. It supports a rich set of metadata capabilities that can inform the semantic tier to help harmonize data across diverse users and applications. Metadata capabilities improve uses around data catalogs, search, linear and compliance.
SAP traditionally used a top-tier pricing model, which was not ideal for smaller organizations. The company transitioned to a pay-as-you-go licensing model aligned with usage. Organizations should examine integration issues closely when trying to extend their capabilities outside of the SAP ecosystem of tools and services. However, SAP continues to invest in integration tools to extend the reach and value of its core offerings. It's a good fit for companies with a strong SAP footprint, particularly those trying to incorporate operational data.
George Lawton is a journalist based in London. Over the last 30 years, he has written more than 3,000 stories about computers, communications, knowledge management, business, health and other areas that interest him.