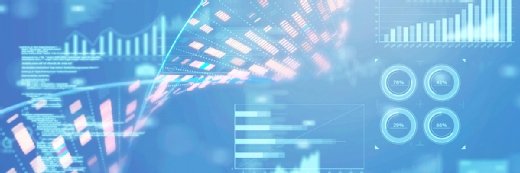
Yuichiro Chino/Moment via Getty
9 metadata management standards examples that guide success
Organizations looking to implement metadata management can choose from existing standards that support archiving, sciences, finance and other kinds of digital resources.
Data-driven decision-making fails without metadata consistency. Selecting the right metadata management standard ensures the consistency your organization needs to compare and analyze data from various sources.
Metadata is information about a data set, such as the creator of a file, the date it was created or modified, the file size, the file name and descriptive keywords. Offering standardized descriptors helps users locate and understand data resources, and it supports effective data governance.
Metadata management standards provide protocols built upon tested foundations of information science and data management practices to ensure consistency. Various standards can offer general applications across different industries or focus on a specific niche.
Benefits of using metadata standards
A structured approach promotes consistency, interoperability, and quality in metadata creation and management. Standards facilitating effective data sharing and retrieval across different systems and platforms improve four aspects of the management process:
- Consistency. Standards provide uniformity in metadata descriptions, reducing ambiguities.
- Interoperability. Standards enable seamless data exchange between different systems and organizations.
- Efficiency. Standards simplify the data management processes and reduce duplication of effort.
- Discoverability. Standards consistently tag metadata, enhancing search capabilities and data retrieval.
Potential downsides of metadata standards
Metadata standards provide significant advantages in organizing and managing data, but it's important to consider disadvantages. Planning for potential downsides can help your organization prepare its metadata to be most effective.
Investment. Implementing standards can be resource-intensive because adopting a metadata standard requires a deep understanding of the standard and the existing data infrastructure. Your organization might need to invest in training personnel, updating software systems or redesigning workflows to align with the new standard. The initial setup can be time-consuming and may face some resistance from team members to process change.
Rigidity. Standards are broadly applicable, but might not cover every unique or new requirement your organization has. For example, if you are adopting GenAI broadly, you might need to record new metadata such as the GenAI model name and version, the prompt used with the model, a confidence score or a flag showing that a human has reviewed the content for ethics. If a metadata standard does not support it, your organization might feel forced to omit necessary metadata or create custom extensions. Both actions can compromise compliance and interoperability, negating some benefits of standardization.
Maintenance. As technologies and practices evolve, out-of-date metadata can introduce errors or break an automated workflow. Even well-established metadata standards change over time to address new developments, industry codes and regulatory requirements. You must stay abreast of all changes to ensure compliance and interoperability. A metadata process requires regular reviews and updates to schemas, training materials and data management techniques.
AI automation and metadata
You can mitigate potential issues with metadata management by implementing automation throughout the metadata lifecycle, which includes making updates when the associated data changes and retiring outdated information.
Automated metadata extraction tools can generate metadata tags directly from data assets, reducing manual effort and human error. Automation reduces the need for manual metadata creation and ensures that metadata is comprehensive and consistent.
Automated processes can run continuously to analyze and interpret data and to update metadata as needed. LLMs can check large volumes of records against updated standards and organizational policies. They can identify metadata elements that no longer comply, signaling the need for revision or even retirement of outdated metadata. LLMs can analyze the usage patterns of metadata to determine which elements are rarely or never accessed to identify data for retirement.
In short, the language capabilities of large language models (LLMs) promise to dramatically change metadata management in the coming years, even though metadata standards may evolve more slowly. The following sections explain some of these standards in more detail.
Metadata standards examples
No metadata standard is universal; different industries and use cases all have their own.
For example, the Dublin Core Metadata Element Set is an example of a widely adopted metadata standard that specifies 15 core elements for describing a resource.
Metadata element | Metadata content |
Title | The name given to the resource. |
Creator | The entity primarily responsible for making the content. |
Subject | The topic or keywords pertaining to the content. |
Description | A textual description of the content. |
Publisher | The entity responsible for making the resource available. |
Date | A point or period associated with the lifecycle of the resource. |
Applying Dublin Core to a Microsoft Excel file, an example table should include all the categories listed in the previous table.
Metadata element | Metadata content |
Title | Partner List for New Product Launch |
Creator | Jane Smith |
Subject | Marketing Partners, Product Launch 2024 |
Description | A spreadsheet containing contact details and profiles of potential marketing partners for the upcoming product launch. |
Publisher | Company Name |
Date | 2024-09-01 |
Adhering to the Dublin Core standard ensures that anyone accessing the metadata can easily understand and locate the file, regardless of the system in use. It's particularly useful for libraries, educational institutions and archives for cataloging resources.
Dublin Core is one of 9 commonly used standards, listed in the following table.
Standard | Industries | Brief description |
Dublin Core | Libraries, education, archives | A simple and widely used set of 15 metadata elements for describing digital resources. |
HL7 Clinical Document Architecture | Healthcare organizations, electronic health record systems | Specifies the structure and semantics of clinical documents for exchange. It enables the sharing of electronic documents across different healthcare settings. |
MDDL | Financial services | An XML-derived specification to facilitate the interchange of information about financial instruments worldwide. It maps market data into a common language and structure, simplifying the exchange and processing of complex data sets. |
VRA Core | Museums, art institutions, historians | A standard for describing visual resources and works of art such as work and image records. Metadata includes creator, work type, material, measurements and location. |
Data Documentation Initiative | Social science, statistics | An XML-based standard for documenting and managing data in the social, behavioral and economic sciences. Popular uses include research institutions, data archives and statistical agencies. |
Metadata Object Description Schema | Libraries, digital repositories | A bibliographic metadata standard that is richer than Dublin Core, but simpler than the Machine Readable Cataloging that advanced institutions, such as the Library of Congress, use. |
Darwin Core | Biodiversity, environmental science | A standard for sharing information about biological diversity by providing a glossary of terms for taxonomic information, geospatial data, occurrence records and ecological relationships. |
Ecological Metadata Language | Ecology, environmental research | An XML-based standard for documenting ecological data sets to facilitate data sharing and reuse. It includes information about creators, methods, data tables and geographic coverage. |
Open Language Archives Community | Linguistics, language archives | A standard for describing language resources to support discovery and interoperability among language archives, including language descriptions, lexicons and language-related tools. |
How to choose the right metadata standard
Successful metadata management requires the right balance between standardization and flexibility, system-wide consistency and the ability to adapt to your organization's unique requirements. Evaluate organizational and implementation needs with standards' features to identify which standard is best for your situation.
Assess organizational needs
Understanding your organization's unique requirements is the most critical step in choosing a metadata standard. If your system has any aspects specific to your business processes, you must ensure standards capture them accurately.
- Data types. Identify the data types you manage -- textual, numerical or multimedia. Knowing your data formats and content types helps you select a standard that adequately describes and handles the data.
- Industry requirements. Consider industry-specific standards that address your data's unique aspects. Some industries have established metadata standards tailored to their specific needs, such as the common warehouse metamodel for data warehousing. Specific standards might offer more relevant features and be necessary for compliance with industry codes of conduct or best practices.
- Interoperability goals. Adopting a widely used standard can make collaboration and exchanging data externally easier.
Evaluate standard features
The next step is to evaluate the features of available metadata standards to ensure they are maintainable long term.
- Flexibility. Choose a standard that can adapt to your data's complexity. A flexible standard affords customization and extension, which are crucial as data evolves.
- Community support. Look for standards with active communities and ongoing maintenance. Strong community support means better resources, updates and troubleshooting assistance, which improve the standard's longevity and reliability.
- Compliance and regulations. Ensure the standard meets any legal or regulatory requirements relevant to your industry. Compliance is essential to avoid legal issues and to maintain trust with stakeholders and clients.
Implementation considerations
The practical aspects of working with the metadata standard are just as important as its features. Important considerations include how effectively you can adopt the standard and the effort it takes to maintain or evolve it as the business grows. Evaluate the current technical resources and skills of your workforce:
- Technical resources. Assess your organization's technical capacity to implement and maintain the standard, including hardware, software and expertise.
- Training needs. Plan for staff training to ensure the standard is applied correctly. Adequate IT training ensures that your team can effectively use the standard, and training for businesses ensures they can benefit from its advantages.
Metadata management is not static. As data ecosystems become more complex and interconnected, traditional standards might fragment and require a more flexible and adaptive approach. Evolution could lead to unexpected and useful innovations in how your organization structures and uses metadata across various industries and applications.
Donald Farmer is a data strategist with over 30 years of experience, including as a product team leader at Microsoft and Qlik. He advises global clients on data, analytics, AI and innovation strategy, with expertise spanning from tech giants to startups. He lives in an experimental woodland home near Seattle.