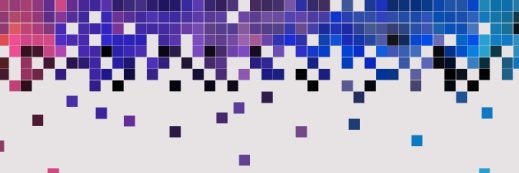
Getty Images
How to use a data governance maturity model
A data governance maturity model identifies where current operations are lacking and how to make improvements that better protect and use data.
Data can't generate valuable insights and improve decision-making without strong data governance. A data governance maturity model helps organizations evaluate current achievement levels and identify steps to improve.
Businesses rely on data to effectively run their business, and they are responsible for protecting data assets. Data governance is an ongoing business process responsible for data oversight, accountability and authority. It provides a control framework defining the rules that govern data across the organization, including people, process and technologies.
Failing to govern data can cause operational inefficiencies, cost increases and system downtime. Noncompliance can lead to civil penalties, monetary fines, brand damage, reputation harm, legal action, consumer mistrust and financial loss.
Organizations must understand the maturity level of their data governance program to realize the full value of their data. A maturity model is an effective tool for objectively evaluating and communicating the performance of a data governance program. It instills confidence in the proactive management of data assets and cultivates a culture of continuous improvement. It can help establish stakeholder trust that enterprise data is appropriately governed, accurately measured and ready for business use.
How a data governance maturity model works
A maturity model gauges the current level of data governance, where an organization wants that level to be and how to get there. It measures various capabilities along tiered levels of achievement, often in a matrix form.
Each row represents a tier of measured achievement. Matrix columns represent capabilities of data governance. The model evaluates and measures the capabilities against achievement tiers using criteria associated with the tier-capability intersection.
Simple markers indicate a benchmark of current state performance or roadmap milestones. A desired target state is enough to measure data governance capabilities. It may not be possible or necessary to optimize every capability.
For more detailed models, capabilities can have various dimensions of data governance individually measured to add value. Dimensions are a lens into a capability and serve as actionable viewpoints in the model. As capability and dimension maturity progress through the levels, achievement increases.
Organizations benefit from the intuitive visualization of a maturity model. It's a great communication and planning tool to foster stakeholder collaboration. It provides transparency and objectivity that enable models to showcase reality rather than unvalidated opinions.
Maturity model insights provide critical information to develop a targeted data governance strategy and subsequent roadmap. They validate the required investment to mature data governance program capabilities. Maturity models better equip organizations to manage effective data governance programs and use data as a strategic asset.
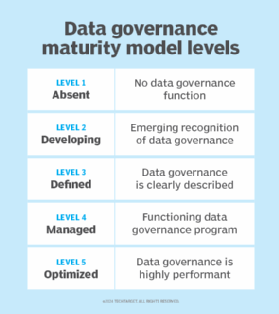
Data governance maturity model levels
Data governance maturity model levels represent tiers of achievement and help ascertain where an organization is currently and where it aspires to reach on a continuous improvement scale.
Maturity models typically have four to six levels of performance. Levels and scale vary depending on the focus of the model. Figure 1 presents example levels:
- Level 1: Absent. No data governance function; characterizes a level of achievement that is unaware and nonexistent.
- Level 2: Developing. Emerging recognition of data governance; characterizes a level of achievement that is fragmented, investigative, informal, inconsistent, unstructured, tactical, ad hoc, lightly documented, reactive and functionally siloed.
- Level 3: Defined. Clearly described data governance; characterizes a level of achievement that is documented, planned, structured, consistent, standardized, repeatable, formalized and cross-functionally aligned.
- Level 4: Managed. Functioning data governance program integrated into critical operations; characterizes a level of achievement that is mostly manual, recognizable, adopted, implemented, trained, institutionalized, communicated, budgeted, enforced and business-driven.
- Level 5: Optimized. Highly performant data governance; characterizes a level of achievement that is integrated into all capabilities of business processes and decision-making, trusted, monitored, measurable, proactive, refined to best practice, automated, frictionless, flexible, continuously improving, evolving with business, collaborative and viewed as a strategic business competency.
Putting it all together
Capabilities and dimensions can, like maturity levels, vary depending on the audience and purpose of a model. For example, an informative model might be appropriate for executives, while an actionable model might be better for stewardship. In either case, scoring rationale needs reinforcement from documented, evidence-based facts.
Depending on where an organization is in its data governance journey, a high-level, aggregated, informative maturity model can help bring awareness to the importance of data governance. Coarse-grained capabilities with a single measure can illustrate the correlation and causation between data governance's alignment to business drivers and its effect on realizing data value.
The example maturity model shown in Figure 2 illustrates how data governance's misalignment with the business can result in underachieving data value for the business.
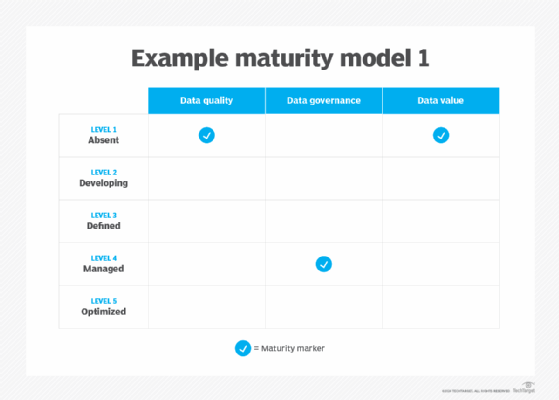
Increasing capability precision also increases the accuracy and value of the model. To gain a high level of actionable insight, an organization should measure capabilities at a finer grain. For additional detail, capability dimensions can identify discrete initiatives to improve specific areas of maturity.
The example maturity model shown in Figure 3 illustrates, at a micro level, that different capabilities of data governance and capability dimensions can have varying levels of maturity.
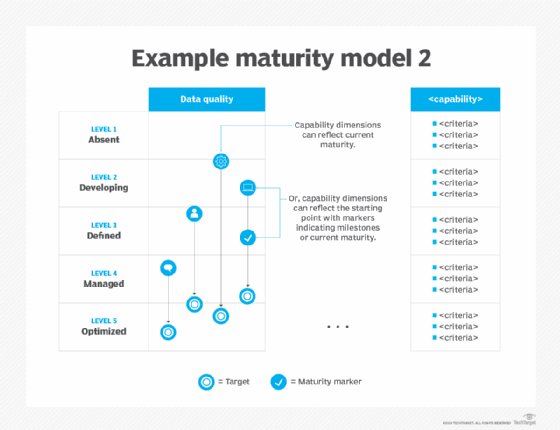
It is important to note that a data governance maturity model measures the maturity of governance, not the capability. For example, the people, process and technology governance surrounding data quality can be mature, while the data quality capability itself is immature if it can only govern 10% of data elements. To gain insight into a capability's maturity, use metrics to measure effectiveness. Data quality metrics might include the percentage of critical data that is of known quality, complete, accurate, consistent, timely and usable.
Jeff McCormick is an enterprise data architecture director and IT principal who has extensive experience in data-related IT roles. He is also an inventor, patent holder, freelance writer and industry presenter.