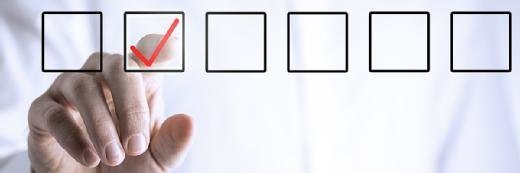
Gajus - Fotolia
6 best practices on data governance for big data environments
Efforts to govern big data must corral a mix of structured and unstructured data. That's a challenge for most organizations. These six action items will help.
In many organizations, data governance used to be relatively straightforward. The business data being governed was mainly generated internally in transaction processing systems and ensconced behind the firewall. Data analysis and reporting applications enabled by the governance program were the province of a select group of IT and BI professionals, who typically used slow-changing processes to analyze data and planned projects well in advance.
As a result, data governance efforts were often treated as a behind-the-scenes IT process.
"Governance was considered synonymous with a bureaucracy tax within traditional data environments to manage risk and drive multiyear data and analytics initiatives," said Yasmeen Ahmad, vice president of global business analytics at data platform vendor Teradata.
The rise of low-cost storage and compute resources and access to more types of data changed all that, inspiring data scientists and business users throughout the enterprise to find new ways to analyze data for operational insights and a competitive edge. Data analytics became decentralized and more self-service, allowing businesses to move faster. "But with greater freedom to access and leverage data comes great responsibility," Ahmad said.
Big data's impact on data governance
The advent of big data analytics has increased that responsibility. Data governance for big data requires keeping pace with a much faster rate of change. With incremental application updates on a continuous basis and the addition of new data sources and analytics methods, data governance has gone from a one-time bureaucratic tax to an integral -- and highly dynamic -- component of big data projects.
Big data governance must track data access and usage across multiple platforms, monitor analytics applications for ethical issues and mitigate the risks of improper use of data. In a big data environment, it's also important that data governance programs validate new data sources and ensure both data quality and data integrity. In addition, enterprises need to watch out for how data from different sources could be combined to create new combinations that violate privacy regulations.
Based on those needs, here are six best practices for managing and improving data governance for big data environments.
1. Validate new data sources
Big data isn't just about large amounts of data; it's also about different types of data and where the data is coming from. Cloud services, social media and mobile apps provide new sources of data to organizations for use in enterprise applications. Companies are also finding ways to democratize the use of this data in order to expand their analytics applications and make them more productive.
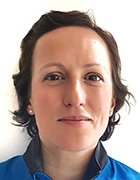
But the images, videos, tweets and tracking data that give companies a better understanding of their customers and other aspects of business operations also create a variety of governance challenges, said Ana Maloberti, a big data architect at IT consultancy Globant.
For example, new data privacy laws like GDPR and the California Consumer Privacy Act add urgency to getting the governance of big data right. The challenges presented by new sources of data were there in the past, Maloberti added, "but nowadays all companies are scrutinized like never before, so a breach or policy violation could mean heavy fines and the loss of customer trust."
New sources of data also introduce challenges on data quality and reliability, Maloberti said. The needed validations to keep a big data environment trustworthy require up-to-date technologies and monitoring tools. It's also important to confer with the legal department on what policies and regulations need to be considered when adding new sources to a big data platform.
2. Understand the quality of data
Data governance for big data must pay special attention to data quality, agreed Emily Washington, executive vice president of product management at Infogix, a vendor of data governance and management software.
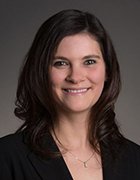
Big data environments contain a mix of structured, unstructured and semistructured data from a multitude of internal and third-party systems. As this mix of data flows across the data supply chain, it's exposed to new systems, processes, procedures, changes and uses -- all of which can jeopardize data quality.
"Data governance, when integrated with data quality, allows users to trust and utilize their big data sets," Washington said.
She recommended asking the following three questions to assess data quality in big data environments:
- Can you trust the source?
- Is it accurate?
- Does it have multiple meanings?
3. Quantify data integrity
The use of diverse applications, databases and systems in big data analytics projects can also make it difficult to identify and resolve ongoing data integrity issues, Washington said.
Data integrity refers to the overall validity and trustworthiness of data, including such attributes as accuracy, completeness and consistency. As part of governing big data, enterprises should find ways to measure and score the integrity of the various data sources in their environments so that users trust the data and feel they can confidently use it to make business decisions, Washington advised.
Data integrity in big data environments
Infogix's Washington elaborated on best practices for tracking and measuring data integrity, providing the following example:
"A marketing team leverages the output of a predictive model to assess the likelihood a newly implemented marketing campaign will be effective for a certain customer demographic over the next three months. The customer data feeding the predictive model comes from a big data repository, which may store thousands of customer attributes.
"The data science team, however, cares about only 200 of the thousands of attributes. By governing those 200 attributes, the data scientists can be certain the required data is accessible, and that values are complete and accurate for that specific model. By scoring and tracking ongoing quality trends, the team can quickly identify and address any bad data that may feed the models to ensure they are providing the marketing team with high-quality analytic outputs. They can also identify when data quality may deteriorate over time to evaluate the root cause and address issues upstream."
4. Shine a light on dark data
Big data can also make it harder for people to develop a holistic view of their data ecosystems, said Lewis Wynne-Jones, head of data acquisition and partnerships at ThinkData Works, a data science tools provider.
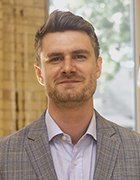
"The challenges for organizations that are incorporating a mix of structured and unstructured data is that their digital blind spot gets bigger as they incorporate more, and different, data into their day-to-day operations," Wynne-Jones said.
For example, an organization might start to pull unstructured news data into its data warehouse or data lake. Even if the organization is running natural language processing over the raw data to pull out the relevant data points, the raw data itself might not be governed in any substantive way.
"Increasingly, governance needs to apply not only to the data that organizations are actively using, but also the dark data that resides in the hard-to-reach corners of their data warehouse," Wynne-Jones said. This will require finding ways to monitor all the data that's flowing into and out of their environment.
5. Stress-test governance for big data
Wynne-Jones said data variety also needs to be considered as part of data governance for big data. Large data volumes and different types of data both add stress to processes that might work fine in a controlled environment.
"Training your governance process on these kinds of data will help you figure out where there are gaps, giving you a sense of where to focus your efforts moving forward," he said.
In his experience, most enterprises have the basic elements of a data governance framework in place. Identifying what's working and why is as important as figuring out what might be missing.
"The first role of someone tasked with implementing data governance should be researching what's out there, not trying to build something new," Wynne-Jones said. As with anything else, iteration is critically important to success, he added.
6. Watch out for toxic combinations of data
It's important to consider how data might be combined in ways that violate GDPR and other privacy mandates.
"While many organizations will mask the identities of customers, consumers or patients for analytic projects, combinations of other data elements may lead to unexpected toxic combinations," said Kristina Bergman, founder and CEO of data privacy tools developer Integris Software.
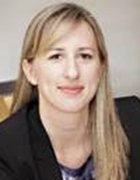
Toxic combinations of data unintentionally blend data elements in a way that can lead to unauthorized identification of individuals. An example would be a data set that provides the date of birth, zip code and gender of individuals. Based on this information, 87% of the U.S. population can be identified, according to Bergman. The rate may be lower for de-identified data, but organizations must exercise due diligence to ensure they protect the privacy of people whose data is used in big data analytics.
Bergman recommended a careful analysis of the data sets in big data systems to understand what inferences could be made about people's identities. This analysis may lead to restricting the use of certain data elements or further anonymization of the data.