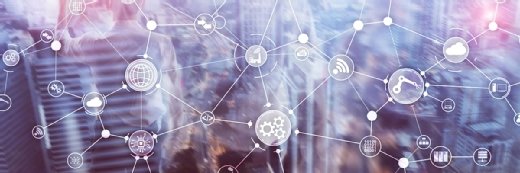
Funtap - stock.adobe.com
Monte Carlo adds more GenAI to data observability platform
The vendor's latest update features an LLM-powered tool that delivers recommendations for developing data quality monitors along with a DataOps dashboard and new integrations.
Monte Carlo on Thursday unveiled a series of new tools aimed at enabling customers to feed data and AI products with trusted data, including generative AI-powered data observability capabilities that help users develop and deploy data quality monitors.
In addition to GenAI Monitor Recommendations, Monte Carlo's latest set of new features includes a DataOps Dashboard to track data quality initiatives and integrations with commonly used extract, transform and load pipelines Azure Data Factory, Databricks Workflows and Informatica.
The new tools were revealed during Monte Carlo's Impact Data Observability Summit, a virtual user conference. Each of the new features is now generally available.
With enterprise interest in AI surging and high-quality data critical to training successful AI models and applications, data observability is gaining importance. As a result, Monte Carlo's emphasis on helping customers feed their data and AI products with data that can be trusted is significant, according to Matt Aslett, an analyst at ISG's Ventana Research.
"Maintaining quality and trust is a perennial data management challenge, which the rise of AI has brought into sharper focus in recent years," he said. "The significance of data observability is increasingly pertinent due to the rise of enterprise AI initiatives that combine enterprise data with AI and generative AI models to automate customer service and business decision-making.”
Based in San Francisco, Monte Carlo is a data observability specialist whose platform enables users to monitor data throughout its lifecycle, checking characteristics such as its freshness, schema and lineage to ensure its quality.
Recently, the vendor unveiled root cause analysis capabilities designed to help users uncover the underlying reasons for code changes that lead to poor data quality.
New capabilities
Analytics and AI tools demand high-quality data. Reports, dashboards, models and applications are only as good as the data that informs them. Without accurate data that can be trusted, analytics and AI tools will be inaccurate and untrustworthy.
Ensuring data quality, however, is impossible for even teams of data experts.
Before the advent of cloud-based data warehouses and lakes that can store massive amounts of data, organizations kept their data in on-premises databases overseen by IT teams. Decision makers had to submit tickets to IT departments requesting them to develop reports and dashboards, and IT teams could carefully check data for its quality before including it in an analytics product.
Now, however, decisions need to be made in real time. And due to the cloud, organizations can collect and store exponentially more data than they could when data was exclusively kept on premises.
As a result, the sheer volume of data -- nearly half of all organizations now manage at least 500 petabytes of data -- makes it futile for data teams to even attempt to observe data for quality manually.
Enter data observability vendors such as Monte Carlo, Acceldata, Metaplane and Soda Data -- among others -- that provide platforms that automate monitoring data.
Like many of its peers, Monte Carlo was founded late last decade. Since its start in 2019, the vendor has added to and improved its capabilities to better enable customers to track data as it moves through pipelines and informs analytics and AI tools. Over the past two years, that has included developing generative AI-powered features to simplify data observability, including a tool that enables users to generate code using natural language, and another uses generative AI to suggest fixes in code.
Now, the vendor is adding a new generative AI feature.
GenAI Monitor Recommendations uses a large language model (LLM) to examine an organization's data and deliver recommendations for developing and deploying data quality monitors. Development and deployment can then be executed by technical and non-technical users alike with previously existing generative AI tools such as Generate With AI.
GenAI Monitor Recommendations is powered by Data Explorer, Monte Carlo's data profiling engine. Integrated with an LLM, Data Explorer uses generative AI to discover relationships between columns and patterns in data that would be nearly impossible for humans to find.
Subsequently, GenAI Monitor Recommendations suggests data quality rules and monitors to build and deploy that help users engender trust in the data they use to train analytics and AI tools.
Trust, meanwhile, is a critical focus for all data management and analytics vendors as they seek to help customers develop analytics and AI tools, according to Aslett. If users don't trust the data underlying reports, dashboards, models and applications, they won't use the analytics and AI tools to inform decisions.
"As enterprises seek to automate aspects of their decision-making processes using AI, it is essential that they have confidence in the data upon which AI depends," Aslett said. "This has increased the focus on data observability software providers and the role they play in ensuring data meets quality and reliability requirements."
Meanwhile, the use of generative AI tools to automate and improve data observability is somewhat nascent, he continued. As a result, Monte Carlo's addition of GenAI Monitor Recommendations is not only significant for users but also could help the vendor differentiate itself from its peers.
"The use of GenAI in data observability is still emerging and has not yet been widely adopted by data observability software providers, so the launch of GenAI Monitor Recommendations gives Monte Carlo a potential competitive advantage over some of its rivals in lowering the barriers to widespread adoption," Aslett said.
Stewart Bond, an analyst at IDC, noted that GenAI Monitor Recommendations fits into the concept of AI for data, which is one of the main areas in which data-driven organizations are investing. AI for data simply means using technologies such as data observability that are embedded with AI to make them more accurate and efficient.
In addition, GenAI Monitor Recommendations aligns with the increasing emphasis on data quality demanded by surging enterprise interest in developing AI tools, Bond continued.
"Data quality has to be addressed to improve the accuracy and relevancy of AI outcomes," he said.
As a result, GenAI Monitor Recommendations is a timely addition for Monte Carlo that addresses user needs, according to Bond.
"Adding GenAI capabilities to Monte Carlo is an important feature for users," he said. "Manual processes are no longer reasonable, and relationships within data not recognizable without using technology to uncover them."
Regarding the impetus for developing GenAI Monitor Recommendations, Lior Gavish, co-founder and chief technology officer of Monte Carlo, noted that much of the vendor's product development aims to help users be more productive and extract more value from their data.
GenAI Monitor Recommendations aims to address both.
"Writing data quality rules takes time, and the larger or more complex the data or the environment becomes, that time really adds up," Gavish said. "With GenAI Monitor Recommendations, our customers receive actionable recommendations for new monitors based on relational patterns in their data, saving them time from having to write dozens if not hundreds of rules manually."
In addition, because generative AI is able to monitor data for semantic meaning, it enables the discovery of relationships between data points and datasets that might not otherwise be discovered, Gavish continued.
"That can catch issues that may have otherwise gone undetected [and do so] before they wreak havoc on the business," he said.
While GenAI Monitor Recommendations provides new generative AI-fueled data observability capabilities, Monte Carlo's DataOps Dashboard is designed to equip organizations with a means of easily viewing operational metrics and tracking the progress of data quality initiatives.
By providing users with a clear view of metrics that show data quality, users will better understand whether data can be trusted and used to inform analytics and AI projects to make decisions or if it needs to be improved before it can be operationalized.
Data observability metrics populating the DataOps Dashboard include response times for monitor alerts, incident resolution times, total number of incidents, their severity and who is responsible for rectifying incidents.
Just as the motivation for developing GenAI Monitor Recommendations is to save users time and derive more value from their data, efficiency and value provided the impetus for creating the DataOps Dashboard, according to Gavish.
"In the same way that [application performance monitoring] solutions help teams not just detect issues but also root cause and resolve them, Monte Carlo is dedicated to helping teams reduce the amount of time and resources spent on data quality," he said.
Meanwhile, given the added insight into data observability provided by the DataOps Dashboard, it is a beneficial new tool for Monte Carlo customers, according to Aslett.
"DataOps can enable data professionals to measure improvements related to the use of data and demonstrate the value of their role to the wider organization," he said. "Monte Carlo already provides users with key metrics related to the health of specific datasets. The DataOps Dashboard provides additional operational context to provide a more holistic view of data reliability."
Beyond the DataOps Dashboard and GenAI Monitor Recommendations, the integrations with Azure Data Factory, Databricks Workflows and Informatica aim to give users visibility into data lineage and data pipeline performance in a single location.
Next steps
As Monte Carlo plans future product development, one of its focal points will be continuing to add generative AI-powered data observability tools to detect, prioritize and resolve data issues, according to Gavish.
Other initiatives include expanding data observability's reach beyond a small audience of development teams to data engineers and analysts and extending data observability to enterprises' entire data estate by adding integrations with data platform vendors including AWS, Databricks, Google Cloud, Microsoft Azure and Snowflake.
Finally, and perhaps most critical in terms of assisting organizations as they develop AI and machine learning tools, is improving data observability for unstructured data.
Unstructured data such as text, images and audio files is estimated to account for over 80% of all data. Including that data in the pipelines that train AI models and applications is critical to making those models and applications as well-informed as possible so they deliver the most accurate results possible.
As a result, support for unstructured data is critical for data observability vendors.
Part of the complexity of the 2025 data landscape is the explosion of unstructured data," Gavish said. "We're working with our customers to build capabilities that allow them to ensure the unstructured data powering their LLMs and [retrieval-augmented generation] pipelines is reliable and accurate."
Eric Avidon is a senior news writer for TechTarget Editorial and a journalist with more than 25 years of experience. He covers analytics and data management.