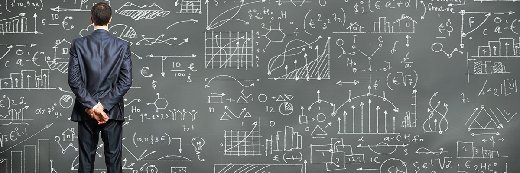
Sergej Khackimullin - Fotolia
What FAIR data management means for your enterprise
The FAIR principles were made to promote the sharing of data in the research field, but their guidance can help organizations in other industries improve their own data practices.
In research, healthcare and biotechnology, there is a growing need to improve support for the reuse of data. But data sets are often incomplete, lack proper documentation or lack accessibility, making that necessary reuse almost impossible. To solve this problem, a group of data professionals designed the FAIR data principles.
FAIR measures the findability, accessibility, interoperability and reuse of data. While these principles have been mainly directed at the scientific and pharmaceutical industries, the intent behind FAIR data management may be worth considering in broader enterprise settings.
FAIR and its intent
FAIR was developed with the intent to provide guidelines for organizations to improve their ability to reuse digital assets. This expands the role and purpose of data and can affect projects across organizations. FAIR was created to harmonize the ways data is captured and managed to allow more collaboration and is another step on the data management industry timeline.
Ruedi Blattmann, managing partner at Life Sciences Consulting Partners, has seen changes to the industry throughout his tenure, and FAIR is another large movement in the ever-changing data management industry.
"The vocabulary is not something written in marble," Blattmann said.
The terms and language in the data management industry have not been set. It is a fluid industry and as technology advances and capabilities increase, so does the vocabulary. Here's a breakdown of each principle:
Findable. Metadata and data should be easy to find for computers and humans alike. To achieve this, each data point should be assigned a unique and clear identifier, should be searchable and relatively easy to find, and metadata should include the identifier of the data it describes.
Accessible. Having put in the requirements to make the data findable, the user should now be able to access data and metadata by their identifier using a communications protocol. This should be open and allow for an authentication procedure if necessary. And metadata should be accessible even when the data is no longer available.
Interoperable. The data needs to be able to be integrated with other data. Also, data must be formatted such that it can be stored, analyzed and processed by multiple applications, not just the one that created it. Metadata and data should use an accessible and broadly applicable language for representation and be described with references to others so it is possible to understand the relations between data.
Reusable. Metadata and data should be well-described enough to permit replication with accurate and relevant attributes and be released with a usage license.
Behind these four pillars of FAIR data management is the goal of making data more broadly available for researchers to take larges sets of historic data and apply them to new questions and studies.
FAIR lessons for the enterprise
Enterprises have a lot to learn from FAIR data principles. The scientific community turned to this approach to increase the availability of data for research and encourage cooperation for the benefit of all. The hope is to gain more insights from existing data by allowing similar studies access to previously gathered and saved data.
Though this isn't necessarily an application that is viable in a cross-business environment where competition reigns supreme and insights are not generally shared, the ability to open silos and create a wider use of data within an organization is valuable. Along with this, the other aspects of FAIR can be constructively implemented in the enterprise.
Findability and accessibility require the elimination of silos within an organization. This opens data access by permitting all departments to collaboratively work on all data taken in by the organization. Working on interoperability and reusability can improve data's lifespan by keeping data gathered useful and adaptable.
Difficulties with adopting FAIR
FAIR data principles are relatively straightforward, but application can be a difficult process. Commitment to FAIR data management requires widespread buy-in from the organization, but even this can't ease the burden completely.
"The key problem is people need to understand data, the dynamics, the content of data and how can it be efficiently reused in the process of the lifecycle of a product," Blattmann said.
Data literacy is crucial to understanding how best to implement FAIR, according to Blattmann. Without an understanding of data, nothing can be done properly.
Legacy data especially can be an obstacle for organizations looking to alter their data practices. FAIR data principles emphasize open access to data and a holistic approach to management. This has not historically been the approach to data within organizations. Legacy data can be unstructured, not consistently tagged or lack common terminology, making it difficult to find and access. Transitioning legacy data to the FAIR principles requires restructuring and oftentimes tearing down old systems while ensuring organizational harmony.
Associate professor at Virginia Commonwealth University Peter Aiken noted that engineers were inventing this technology as it became necessary. But few people built systems with a holistic view of enterprise data in mind, which created silos that remain difficult for many organizations to tear down.
"Nobody since then has been willing to take the time to eliminate what we call the data debt that occurs around all of this," Aiken said.
This data debt occurs when data is improperly handled because of either poor data management practices or lack of understanding. Without committing to going back and solving these issues from years past, organizations won't get what they need out of their data.