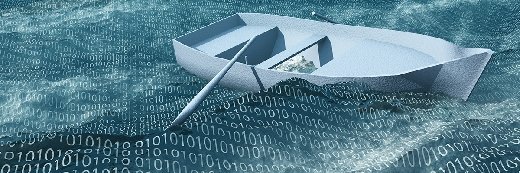
rolffimages - Fotolia
Key factors for successful data lake implementation
There are many important parts to a data lake implementation, from technology to governance. Read on for the top factors to evaluate in your implementation strategy.
In addition to the business drivers behind the growth of data lakes, the cloud's ability to offer vast amounts of storage and processing power at ever-decreasing price points are making data lake platforms increasingly attractive to organizations of all sizes.
Data lake implementation continues to capture the attention of the IT community. A recent analysis report from Research and Markets forecasts that the data lake market will grow by a 26% compound annual growth rate (CAGR), reaching $20.1 billion by 2024.
If your organization is considering a data lake implementation, here are some things you should consider.
What is a data lake?
An easy way to define and better understand data lakes is to compare them to data warehouses. Although data warehouses and data lakes are both used to store large amounts of data, there are significant differences.
Organizations can use data lake information in many ways, and the data sources do not need a predefined purpose to qualify for ingestion into a data lake. Analysts explore, experiment and evaluate data lake information to identify its benefits and use cases. Meanwhile, data warehouses ingest and store data for a predetermined purpose.
Data warehouse specialists often perform a high level of analysis to evaluate and identify input sources. But the strategy for a data lake implementation is to ingest and analyze data from virtually any system that generates information.
Data warehouses use predefined schemas to ingest data. In a data lake, analysts apply schemas after the ingestion process is complete.
Data lakes store data in its raw form. As a result, data ingestion is a fairly uncomplicated process. In a data warehouse, data is heavily processed during ingestion to ensure it adheres to the schema and its predefined purpose.
Data lakes specialize in ingesting structured, semistructured and unstructured data. They also provide mechanisms to easily ingest streaming data in addition to batch loads. Although data warehouses can accept many different forms of data, they usually ingest structured data using batch loads.
How to get started
The first step in data lake implementation is to learn more about data lake architectures, platforms, products and workflows through vendor websites and other resources.
Like any product evaluation, your organization will need to perform a thorough analysis of the competing offerings. Here is a starter list of evaluation criteria to help your analysis:
Technology. Although Apache Hadoop and its suite of supporting products have been the perennial favorites for many organizations, there are a growing number of alternatives. Many vendors that use Hadoop for their data lake offerings provide their own customizations and edge products to simplify, streamline and facilitate administration and analysis.
There are a wide range of platforms available, including Amazon Data Lake Solutions, Microsoft Azure Data Lake, Google Data Lakes, Snowflake for Data Lakes and Oracle Data Lake.
Security and access control. Data lakes hold a treasure trove of information about your business. Like all enterprise data stores, you will need to protect data lakes against unauthorized access.
Data ingestion. Does the platform easily and quickly ingest structured, semistructured and unstructured data? Is it capable of efficiently ingesting data streams, micro batch and mega batch data loads?
Metadata management. Big data specialists use metadata to search, identify and better understand the data sets that are in the data lake. How does the platform capture and store metadata?
Data processing, performance and scalability. What tools and processes does the platform offer users to interact with the data? How does it enable data exploration? What background processes does it execute during the course of daily operations? How fast are those processes and will they scale to meet your workload requirements?
Management and monitoring. Does the platform provide a strong UI for system administration and monitoring? What workload management capabilities does it offer?
Data governance. Does the platform offer mechanisms to ensure the data is consistent and reliable? Does it provide the ability to create sandbox environments that allow users to experiment with data without affecting the contents of the data lake?
Data analysis and accessibility. What mechanisms does the platform provide to analyze the data? Does it allow you to easily incorporate machine learning? What data analytics features does it offer to consumers? Can you easily integrate third-party analysis tools?
Costing strategies. How will the vendor charge you?
Data lake implementation
After platform selection, the next step is to build the organizational infrastructure, processes and procedures to load, govern, administer and analyze data in the data lake.
These are the key steps in a data lake implantation strategy:
- Identify the expertise you need to effectively support the platform and analyze the data. Like many complex technologies, data lakes have a steep learning curve. Hire experienced personnel and train internal staff. Your organization will need to define new organizational roles and reporting structures with data lake implementation.
- To execute a well-thought-out data lake implementation strategy and design, your organization will need to develop a traditional project plan with goals, milestones and assigned action items. You will need to identify the criteria your organization will use to evaluate the success of the data lake project. Design the system to foster self-service data analysis. You should also develop data classification standards for data storage and archival.
- Virtually any data the organization generates is a potential source for data lake ingestion. The challenge becomes one of prioritization. A good approach is to evaluate the source that generates the data and identify its importance to the organization at a high level.
- You should determine if the information is currently being analyzed and the level of analysis that is occurring. Highly analyzed data, although still a potential source for ingestion, may have a lower importance than data from a system that is not being evaluated.
- Develop, implement and enforce data governance strategies to ensure the data is secure, complete, consistent and accurate.
- Establish standards for data exploration, experimentation and analysis. Data scientists should follow a standardized but flexible process to evaluate the data and identify the use cases that will generate the most value to the business. Potential targets for the data are other BI platforms and new and existing business applications.