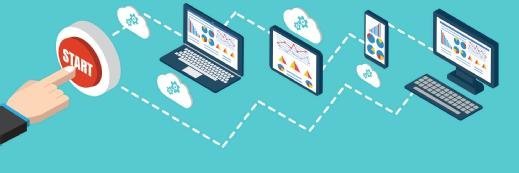
Seraphim Vector - Fotolia
How to streamline your data cleansing process
Data cleansing is an important part of maintaining data quality, and the process is easier if you keep ahead of it by upholding governance and quality standards.
Data drives so much of our lives these days, but how trustworthy is it? A 2017 KPMG Global CEO Survey found that over three quarters of CEOs were concerned about the quality of the data they base their decisions on, while a January 2020 survey of 646 data scientists by Trifacta, a data wrangling vendor, found much the same result -- 74% doubted their data was accurate. These data errors cost money, sometimes a lot of money.
In my own experience, I worked with a mobile phone manufacturer that once ordered a vast number of boxes for a new phone. There was an error in the contract specifying the dimension of the box in the wrong unit of measurement, and the mistake was only realized when the shipment arrived with boxes 10 times larger than intended. This error alone cost the company 25 million euros. A data improvement project I was involved in found 80% of the customer master file was duplicated. People do not deliberately set out to make these errors, but they creep in.
Given this state of affairs, what can organizations do to actually sort out and maintain the data cleansing process? There are several issues to tackle, some technical and some organizational.
Data governance in your data cleansing process
A one-time cleanup may help in the short term, but it will not work for long unless the underlying structural issues are addressed.
It is important to establish someone to be responsible for data quality within a company or business unit. Data quality cannot simply be delegated to the IT department, because in most cases they will not have the authority to change the business processes that are causing the issues in the first place.
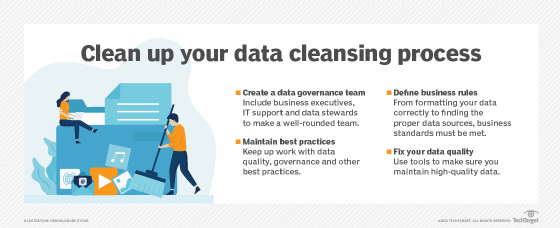
Organizations that have taken this problem seriously have set up data governance initiatives, led by a senior business executive. These initiatives usually consist of a steering group of senior people with authority, a small full-time team to support the work and part-time data stewards within the various business lines who are responsible for data quality within their particular areas.
The role of the data governance group is to raise the quality and reliability of key data in the organization, addressing issues of data duplication, ownership, quality, accessibility and timeliness. Data quality goals can be set by this group, such as "at least x percent of customer records must have a validated postal code" and similar benchmarks.
Assessing your data quality
The next step is to produce a baseline assessment of data quality, and technology can help here. There are dozens of good data quality tools out there. Many have a data profiling capability, where existing databases or files are scanned and summary statistics are produced to give an initial picture of the state of the data.
Profiling will look at the structure of the data and do basic statistical analysis to identify incomplete data fields, apparent duplicates, null data and anomalies. Profiling may identify which values fit a certain profile like a postal code, social security number or a bank routing number and can highlight any data items in a file that do not match the profile format.
Many data quality platforms have algorithms to comb through data sets and spot likely problems. For example, one of these tools might realize that "Andy Hayler" and "Andrew Hayler" with the same address are likely the same person. Some data quality tools use artificial intelligence capabilities to improve their duplicate spotting and matching skills. These tools can also validate postal codes by looking up regularly refreshed postal databases.
Data quality platforms often have algorithms that spot common typing errors. The platform can look at multiple fields associated with a record to assign a probability of it being a match to another record. Sometimes manual intervention is needed, but you can set thresholds so a match probability greater than a certain percent is dealt with by the platform without referring it to a human.
These tools can help you narrow down where you need to focus during the data cleansing process.
Defining business rules
Once you have a handle on your data, you can go further and define business rules to which that data should conform. For example, product codes and customer numbers will usually follow a particular format.
Beyond formatting, you will need to identify your organization's data sources and where duplicate data is held. Common master data like customer, asset, location and product will be scattered across many systems, but you need to determine which source is the most trustworthy.
Try to be ahead of the process
Once you have gone through these steps and have started the data cleansing process, it is important to put strategies in place that keep the quality high in the long term. Data quality is ideally applied at source. For example, a computer system used by sales staff could automatically detect a likely existing account to avoid the creation of an accidental duplicate.
You want to set up reporting systems that monitor the ongoing quality of the data. See whether there is a sudden deterioration in data quality in a certain area, figure out why that is and fix the underlying problem as well as the problematic data. It is vital to understand that data quality is a journey, not a destination.