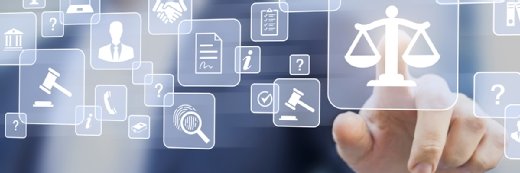
Getty Images/iStockphoto
How data governance and data quality work together
High-quality, reliable data is essential to the data governance process. Here are strategies to ensure data quality standards are ingrained in governance processes.
Data quality is an important pillar in the data governance framework and plays a vital role in an organization's ability to meet established governance standards.
While both exist as individual models, effective implementation of data quality and data governance structures has the potential to produce a symbiotic system that ultimately upholds an organization's strategic goals and informs decision-making.
Data governance and data quality: The key differences
It is first important to understand data governance and data quality as distinct concepts. Data governance refers to the oversight of an organization's information. It is a process that delineates owners who have rights to view and utilize information. It standardizes how this information is collected, stored and ultimately analyzed or disseminated for a specific use.
Data governance is somewhat of an umbrella term that encompasses several components. These components are typically formalized in a data management plan or data standard.
Michel Girard, Senior Fellow at the Centre for International Governance Innovation, stated in his 2020 research paper Helping Organizations Master Data Governance: An ideal governance standard should 1. include objectives the organization is pursuing, 2. identify the scope of data covered by the data governance standard, 3. designate a position that is accountable for the application of the data governance standard, 4. bring clarity on data ownership rights, 5. articulate how the data collection should be handled, [and] 6. describe how relevant data sets and data streams should be accessed and shared.
Girard also highlighted other areas -- such as compliance, privacy and security -- as pieces of the governance puzzle, demonstrating the extensive nature of a data governance system. In its simplest form, data governance can be surmised as strategic and intentional management of information.
To establish data quality, there should be standardized practices to monitor data integrity and bring forth inconsistencies or inaccuracies in the data being processed. A comprehensive data quality system outlines clear pathways for troubleshooting areas of concern, implementing improvements and establishing continuous monitoring.
The end user of the information also affects what is considered quality data. If the information being furnished is not useable, regardless of the validity and completeness, the data could be considered poor quality because it does not meet the end user's needs.
The principal difference between data governance and data quality is that data governance provides oversight and management of an organization's information, whereas data quality is focused on the integrity and value of the information itself. These two forces possess their own intricacies, but organizations have an opportunity to capitalize on their multifaceted nature to build complementary data quality and data governance structures that help meet organizational goals.
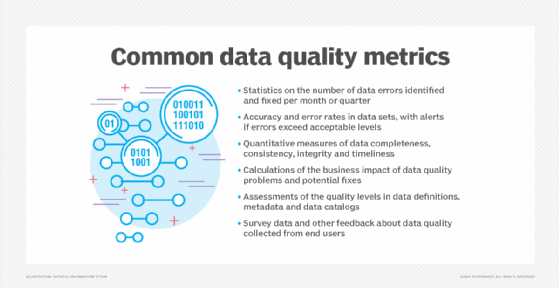
Where they intersect
Compliance is one of the areas in which data governance and data quality intersect. Take the healthcare or education sectors as examples: Both have regulations that delineate rights to information and how that data can be accessed and shared.
In healthcare, HIPAA protects patient information; in education, the Family Educational Rights and Privacy Act protects student information. A comprehensive data governance plan should reference governing regulations that detail requirements for managing an organization's unique data storage, privacy and security requirements.
To complement these regulatory needs, data quality systems should be designed to monitor information that an organization is required to safeguard or report back to a regulatory entity.
This same information should be monitored for completeness, timeliness, accuracy and validity to ensure compliance with the regulations. The data quality dimensions should support the governance standard.
Incorporating data quality in data governance standards
The data governance standard should incorporate data quality processes and quality dimensions to ensure data that informs decision-making is meaningful. Girard suggested that "ideally, individual organizations should be able to treat data governance the way they treat quality management, through the adoption and implementation of a normative document covering all relevant aspects." This "normative document" from a data governance perspective is known as the data governance standard, or data management plan, and should parallel the data quality plan.
Organizations can bridge these two plans to ensure that data quality initiatives and improvements support the objectives highlighted in the data governance standard. Ultimately these two areas should also align with the organization's strategic plan.
Additionally, the individual or individuals who are accountable for and have ownership over the implementation of an organization's data governance standard should be strategically woven into several aspects of the data quality system.
For example, management personnel should be routinely briefed on data integrity issues within an organization. They can identify staff resources to assist a quality or data quality team in troubleshooting integrity issues. These managers typically supervise individuals who enter data into a system and therefore can have direct impact on the quality of information at the point of data collection. Managers can help streamline workflows and standardize how information enters a system to ultimately reduce the frequency of errors within a system and improve the accuracy and validity data.
Those governing an organization's information should also participate in data quality improvement initiatives, as they are subject matter experts on an organization's governance standard. This is especially valuable when these improvement initiatives are related to compliance.
Managers in the governance system will also inform what is considered quality data as they typically receive various data analyses and summarizations. Managers should help develop how the quality dimensions come to fruition within their organizations and define what data accuracy, completeness and timeliness mean for their organization.
As the data governance framework is refined and standardized over time, organizations should seek to embed quality standards into their data oversight structure to ensure the information stored and shared within their systems is valid and can be used to meet organizational needs.