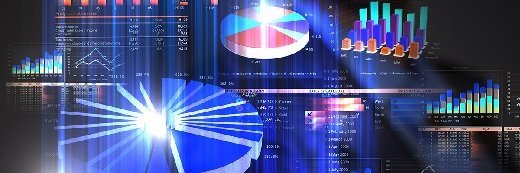
Sergey Nivens - Fotolia
Enterprise augmented data management benefits and growth
Gartner predicts plenty of growth in the booming augmented data management market, which helps data professionals focus on insights over administrative tasks.
Managing the unbridled growth of an organization's data stores is a significant challenge for IT shops.
Ever-increasing administrative workloads are compelling many IT departments to find ways to reduce the amount of time they spend storing, analyzing and presenting information to users. Organizations are constantly searching for products to reduce the amount of time their IT support professionals spend maintaining current systems to allow them to focus on higher ROI activities.
Vendors of all sizes are capitalizing on an interest to automate data management activities by releasing new products or enhancing existing offerings. A growing number of competitors offer time-reducing automation and AI and machine learning features that help organizations improve their data integration, metadata management, database administration, analytics and data governance capabilities.
Augmented data management growth
One of the fastest-growing trends is augmented tools that assist human workers to collect, prepare, store and analyze data and share business insights. A recent Gartner study predicted that manual data management tasks will be reduced by 45% due to augmentation through 2022.
Let's dive a bit deeper into augmented data management by looking at a summarized version of the information lifecycle and how augmentation plays a role in automating it.
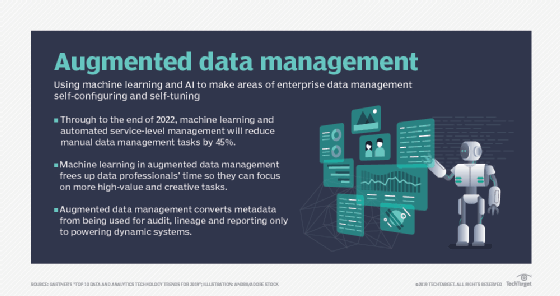
Data documentation and governance
Data governance provides a blueprint of controls to ensure effective management of data at the enterprise level. The goals of data governance are to break down data silos to promote information sharing across the enterprise and to ensure an organization's data assets are accurate, maintained consistently across systems and not misused.
A key component of data governance is metadata management. In order to turn data into information you can trust, you first need to collect it and record information about its meaning, business use, source, lineage, context and relationships to other elements. That information is known as metadata -- or data about data. Data specialists explore metadata repositories to identify and better understand the data assets that are available for use.
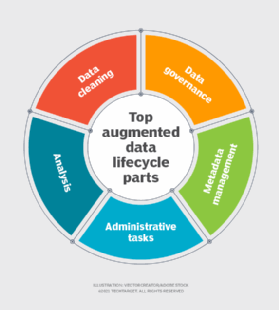
It is an easy assumption to make -- as well as a gross understatement -- that overall data governance and the effective management and documentation of an organization's data assets are time-consuming and expensive.
The information management market arena has exploded with dozens of products, ranging from applications focused on a specific set of administrative activities to general-purpose platforms that attempt to encompass the entire scope of the data governance process.
The one aspect that vendors have in common is their strategy of utilizing advancements in automation, AI and machine learning to differentiate their products from competing offerings. Here are a few sample vendor offerings with unique sets of augmented data management features.
- Informatica. Informatica is an industry leader that provides a wide range of data management offerings. The vendor recently announced augmented data management innovations integrated in its Intelligent Data Management Cloud products.
- Infogix. Although Infogix offers a broad range of data governance products, it now offers augmented metadata management strategies.
- IBM. The industry heavyweight continues to implement the latest advances in augmented data management across its suite of data governance products. IBM uses automation, AI and machine learning in its IBM Watson Knowledge Catalog to facilitate enterprise data governance.
Data infrastructure management
In a highly competitive market arena, database management system vendors understand their products must offer features that simplify and automate administrative activities. Vendors are aware that ease of administration and time-reducing automations are key evaluation points for organizations comparing product offerings.
Vendors will continue to integrate automations and product-generated recommendations that reduce the amount of time database administrators spend on repetitive, mundane and low ROI administrative activities.
Artificial intelligence for IT operations (AIOps) is a collection of big data, AI, machine learning and automation technologies that assist data infrastructure support personnel to quickly resolve issues and more effectively support large-scale enterprise computing environments.
Some examples of automation for data infrastructure management include:
- Oracle. An excellent example of using automations to reduce administrative task time and improve system quality is Oracle's Autonomous Database.
- Microsoft. Administering data platforms includes managing the cloud and on-premises versions of their OSes. Microsoft's Operations Management Suite provides a console that automates a wide range of administrative activities for both cloud and on-premises OSes.
- BMC Software. In addition to product manufacturers, third-party administration tool providers can focus on automating data infrastructure support activities. BMC's TrueSight Automation for Servers is an excellent example of how third-party product providers use augmented data management features to reduce administration time and improve support quality.
Data analysis and presentation
In the early stages of data analytics, it became readily apparent to everyone in the IT industry that data scientists spent too much time collecting, storing and preparing data for analysis -- time that could be spent analyzing that information to generate business insights.
Analytics vendors quickly understood offering automation, AI and machine learning features to help data scientists prepare data for analysis and guide them through data exploration would provide their products with a distinct competitive advantage.
The current industry buzzword to describe these features is augmented analytics.
Augmented analytics uses AI, machine learning and automation to improve data ingestion, preparation and analysis capabilities with the goal of enabling data scientists to generate and distribute high-quality insights. Augmented analytics also assists expert and citizen data scientists to more effectively explore data by generating recommendations that guide them through the data analysis process.