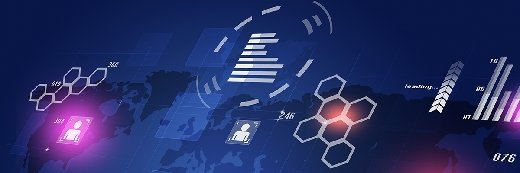
alex_aldo - Fotolia
Augmented data preparation the next step for self-service BI
Augmented data tools play a key role in expanding data use across organizations. Read on to find out how augmented data preparation tools democratize data in self-service BI.
Self-service BI promises to unlock the power of analytics to a much larger audience across the organization. These tools can be straightforward once data sources have been vetted and organized by data scientists upfront. However, they can struggle with incorporating data from new data sources, particularly unstructured ones such as documents, emails and contracts.
Augmented data preparation tools can automate the processes of extracting, transforming and loading data for data democratization projects. Augmented data preparation employs machine learning algorithms that automatically detect and analyze data usage to blend, find data relationships and recommend best actions to take for cleaning, enriching and manipulating data. This allows business users to spend more time analyzing data and less time getting it ready.
"Most personnel tasked with preparing data for analytics find themselves spending too much time on data preparation compared to building models and delivering analytic insights for their organization," said Todd Wright, head of data management solutions at SAS.
The use of data preparation tools has greatly reduced the time investment.
"The rise of augmented data preparation promises to take it even further, while adding benefits like low-code/no-code, easy data collection and integration, AI/[machine learning]-based data inspection and quality checking, and the ability to generate models from little data," Wright said.
Benefits of augmented data preparation for self-service
Augmented data preparation can decrease an organization's reliance on data engineering teams, allowing projects to move more quickly. It also makes it easier to build analytics that use the knowledge and intuition of the staff members closest to the problem, said Mike Chrzanowski, business intelligence expert at Senacea, a spreadsheet consultancy in the U.K.
Business users can also apply these tools to enhance their understanding of what data means.
"Only through truly working with the data can business users and analysts understand it as they will become intimately familiar with the fields, the values and the relationships within the data," said Eva Murray, technology evangelist at Exasol, an analytics database platform. This can lead to better, more meaningful analyses as the analytical process goes deeper than simply scratching the surface and visualizing results.
Augmented data preparation tools can also automate some of the repetitive work subject matter experts have of pulling important data out of long contracts, said Jesse Spencer-Davenport, marketing director at BIS, a document management platform.
Data can be automatically extracted by programming the understanding of a subject matter expert into an augmented data preparation tool. Humans can focus their attention on verifying the data and checking for exceptions.
Some businesses also find ways to use augmented data processing to reduce the post-processing aspects of BI, said Rico Burnett, global director of client innovation at Exigent, a legal outsourcing service provider. Because end users can be more directly involved, there tends to be fewer cycles of review and reduced effort to create focused data reports or bespoke insights for strategic areas within the business.
Adoption challenges
Enterprises face a variety of challenges in getting augmented data preparation projects off the ground, including data accuracy, improving data literacy, managing context and promoting trust -- business users are not as familiar with the technical complexities of data preparation.
"They tend to miss seemingly trivial details related to the new BI system setup, which may hinder the data validity," Chrzanowski said. Even merging data fields with date and time may lead to the creation of new discrepant fields because of the data formats determining background calculations.
The best remedy is clear and precise labeling of data. Keep technical abbreviations to a minimum. Unified terminology used consistently across columns names can work miracles, Chrzanowski said.
Another challenge is that the tools can make it too easy to work with data, even if users don't understand the statistical concepts underlying the results.
"The risk is that people provide outputs for decision-making without truly understanding the data, and they may therefore deliver insights that are not robust enough," Murray said. She recommends starting off by building data literacy across the organization rather than just adopting an augmented data preparation tool and hoping for the best. Everyone needs to understand data and be ready to challenge assumptions and results.
The context of data can be lost when it is moved between operational systems and BI/analytics systems.
"We need the full and correct context of the entity being moved," said Yuval Perlov, CTO at K2View, a DataOps platform. This problem is compounded with streaming data, which only includes a partial view. Data engineering teams can reduce this problem by modeling digital entities using a logical data schema. This can unify all the data for entities regardless of their structure and data transport method.
Managers implementing augmented data discovery need to build trust that employees won't lose their jobs and help them trust resulting insights.
"Organizations must address concerns that current jobs will change once augmented analytics projects are off the ground; otherwise, employees may find creative ways to slow the adoption of the tools or worse," said Wayne Connors, managing director of ACCL, a data cabling service in the U.K.
One big challenge is that the field of augmented data preparation is still in its early days, and there is no playbook to follow for best results.
"As such, we don't have an understanding of things like how bias would affect the prepping of data," said Clive Bearman, director of product marketing for data integration at Qlik. Consequently, there's a lot of uncertainty about the validity of the resulting prepared data sets. For example, a bias error could be unintentionally magnified during the analytics preparation pipeline.
Wright recommends organizations take a proactive approach and determine how the data augmentation process will play out with full team agreement. This includes determining what data will be used, what sources the data comes from and how it will all be fed to downstream systems. Procedures are also needed to check data to determine if the augmentation is effective.
Top vendors
Many major BI vendors are expanding into some aspects of augmented data preparation. Burnett said the larger providers, such as IBM Analytics, Microsoft's Power BI and SAP's Analytics offerings are key players.
"The user experience is exceptional, and the user's ability to jump into data discovery is a key benefit that attaches to larger providers," he said.
Chrzanowski said a good tool should provide a familiar look and feel that is easy to work with in order to keep users engaged. He found Power BI to be an excellent choice for users familiar with Excel and Power Query.
Bearman said some of the vendor categories chasing this market include standalone data preparation folks such as Trifacta, analytics pipeline vendors such as Alteryx, analytics leaders such as Qlik and traditional data providers such as D&B or Experian.
Murray said her augmented data preparation vendor shortlist includes Alteryx for designing a better data preparation process and Talend for cleaning and completing data to create a single source of truth. She also said that Tableau Prep can be a helpful tool for quick preparation of data for analysis and visualization in Tableau.