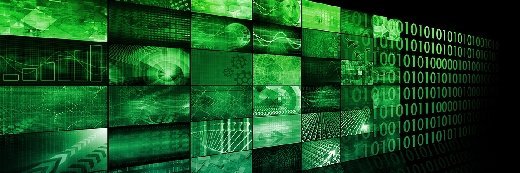
kentoh - Fotolia
Analytics demands add loftier goals to data warehouse strategies
As the concept of storing data and the technologies needed to do it evolve, companies with set goals in mind are building their data warehouses to maximize analytics outcomes.
When running through the gamut of storage options and what each one offers, data warehousing has a multitude of descriptions attached to it.
For that reason, many are still trying to define exactly what a data warehouse is and how it can be applied to their architectures. Among these inquisitive souls is Fernando Tadakuma, data engineer at S4, a technology services company that provides risk management for the food industry. S4 manages and analyzes more than 200 petabytes of data, and his team has struggled to determine how data warehouse strategies can provide them with the most value.
Tadakuma and his team noticed that the concepts of database, data mart, data warehouse and data lake are viewed differently in academia compared to the business world. This gap between theory and reality, he said, has been fueled partly by the ignorance, omissions or marketing strategies of warehousing vendors. As a result, Tadakuma realized he and his team needed to adopt a wide range of tools as part of their data roadmap.
Applications that require high levels of interaction are considered data warehouses. But data sources can be transitioned back and forth between data warehouses and data lakes. "The passage between one and the other currently has a low degree of automation and requires 'human' criteria that we are moving to autonomous tools linked to commercial objectives," Tadakuma explained.
S4's data ingestion process involves collecting data from multiple channels such as satellite imagery sources, weather stations, drones, farming machinery, banks, government data sets and users. That information pours unfiltered into their data lake. Based on the company's current and existing data plans, filters move some of this data from a relatively slow and cheap data lake infrastructure to a more performant one called "le base" -- the data warehouse.
Maintaining cost levels is a key focus. "We work hard so that this warehouse has purpose-built views through which the different technical profiles can easily look up relevant data for their needs," Tadakuma said. But sometimes data managers require faster response times or particular domain joins that a user can't perform. These tasks are implemented in a data mart with a specific database engine to create custom algorithms for various risk modeling scenarios.
"All the time we invest to explaining data, models, technologies and theory to the people who consume or participate in some way in our data process is time that then ends up being beneficial for the entire organization," Tadakuma noted.
Filling seats at all costs
Sports teams are starting to employ data warehouse strategies to help strike a balance between ticket sales and filling stadium seating capacity, said Al Cordoba, chief data officer and scientist at Qualex Consulting Services. "The sports teams that we support want to squeeze every last drop of revenue out of the seats in their stadiums at the same time they maximize the fan positive experience," he said.
It's therefore important to set the price of the ticket at a level that puts fans in the seats and maximizes revenue. Cordoba's team of data scientists developed a dynamic pricing algorithm that takes in historical data from the data warehouses and monitors fan behavior. The data warehouses store and provide modeling data with built-in variables that affect ticket buying decisions like weather, the team's opponent or the team's performance, along with weekly sales.
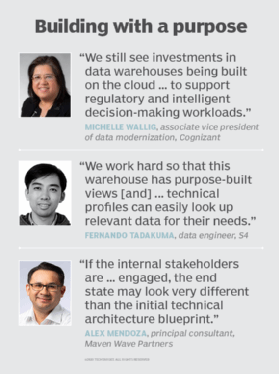
Based on this data, the price of tickets may be increased or decreased depending on whether the algorithm predicts high or low ticket demand for a particular game. Analytics recommendations on ticket pricing are output to standard formats and integrated with ticketing operations. "Making certain tickets available for specific fans also increases fan satisfaction," Cordoba noted.
Boosting BI
Data warehouses collect data generated by operational, ERP, HR, analytics and financial systems that is used to support predefined reports and analytics such as general ledgers, executive dashboards and marketing campaigns.
"Data warehouse data is typically consumed within business intelligence dashboarding and reporting tools," said Craig Kelly, vice president of analytics at cloud consultancy Syntax. "It's sort of like a car dashboard providing high-level aggregated data, except in this case, when you click on the check engine light, you can actually see what the underlying problem is rather than having to go to the shop to find out."
When formulating data warehouse strategies and optimizing warehouse performance, data managers need to weigh the true cost of ownership beyond simply the cost of data warehousing hardware. Kelly suggested that the investment in extract, transform and load tools; planned software applications; and associated database licensing fees also need to be considered.
It's important to incorporate sizable horsepower into the warehouse architecture as well as newer technologies such as columnar storage. "The quickest way to kill user adoption of your data warehouse," Kelly added, "is to have your dashboards and reports take time to run."
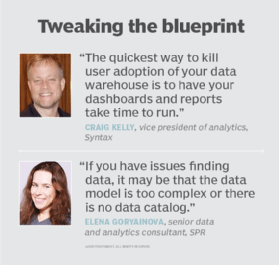
The quality of data stored in a warehouse is also a concern. "Data warehouses provide advantages when data needs to be aggregated and logically organized for efficient analytics," said Elena Goryainova, senior data and analytics consultant at SPR, who primarily uses warehouses for BI and analytics applications.
Her biggest challenge is data quality issues such as missing source data at a specific point in time. "If you have issues finding data," Goryainova said, "it may be that the data model is too complex or there is no data catalog."
Another concern is performance degradation when a data warehouse can't handle concurrent workloads. A good approach to resolving that problem is working with data warehouse teams to change processing or consumption patterns.
Data warehousing's timeline
Though the spotlight most recently has been focused on storage in the era of big data and the cloud, warehousing of data has a long track record. Many companies have invested in data warehouses dating as far back as 30 years, according to Michelle Wallig, associate vice president of data modernization at AI and analytics consultancy Cognizant. She categorized three distinct periods in the evolution of data warehousing:
- Solve for schema -- late 1990s to 2003. Most warehouses were built to organize data across the data continuum from acquisition to preparation to aggregation.
- Solve for performance -- 2004 to 2015. The focus shifted to performance constraints resulting from the limitations of infrastructure and the need for increased access to data. Appliance-based data warehouses dominated because they delivered solid performance for queries of large data sets.
- Solve for elasticity -- 2016 to 2020. While performance and scalability have been solved, the convergence of the cloud with data and analytics led to the simplification of data warehouses to emulate data lake architectures. Failure of large data lake projects -- second-generation big data projects -- demanded cloud-based data warehouses to support unstructured data.
Wallig sees a strong interest in data warehouses even as enterprises move to the cloud. "We still see investments in data warehouses being built on the cloud with Azure, AWS, Snowflake and Google to support regulatory and intelligent decision-making workloads," she said. Data warehousing technology has also matured to support autoscaling, while becoming more efficient and less expensive to operate.
It's more about purpose than perfection
In the end, the true value of data warehouse strategies is measured by purpose not perfection. "If the internal stakeholders are understood and engaged, the end state may look very different than the initial technical architecture blueprint for a data warehouse," said Alex Mendoza, principal consultant at Maven Wave Partners LLC.
A business data warehouse user, for example, may only anticipate five metrics from a profit and loss perspective. But in some cases, there are over 50 in the data source that need to be calibrated and aligned, which can often create a challenge when IT teams don't want to release the warehouse until it's perfect.
For the sake of expediency, sometimes it's better to get the storage system out the door than try to build the perfect data warehouse, Mendoza reasoned. "In a perfect scenario," he said, "a data warehouse can also be used as a mechanism to surface data quality issues and allow discussions to happen with hopes of fixing the root cause instead of masking them with complex data exception rules."