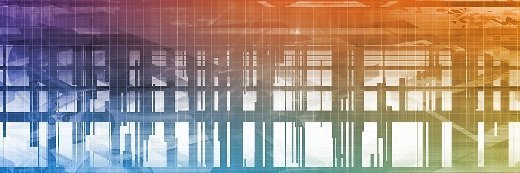
kentoh - Fotolia
Why hyper-converged edge computing is coming into vogue
Hyper-convergence helps enterprises better provision applications at the network edge and more efficiently process enormous amounts of data generated by IoT devices and smart sensors.
Enterprises face an epochal shift in their network traffic patterns and data flows akin to the monumental changes wrought by the confluence of smartphones and streaming video cord-cutters that struck broadband and wireless operators. The result -- in both cases -- is an enormous increase in the amount of network traffic generated and consumed at the network edge.
Just as phone users streaming Netflix stressed mobile networks into triggering significant changes in network architecture, the proliferation of IoT devices, smart sensors, remote worksites and mobile users is requiring enterprises to rethink how to provision enterprise applications and process data. For many organizations, the explosion of data generated at the network edge will necessitate -- if it hasn't already -- moving data processing and analysis out of central facilities and cloud locations to localized systems sized for the task.
While some of these could be embedded appliances combining IoT sensors with a local system on a chip (SoC), larger workloads and user populations require stand-alone compute and storage systems. Such scenarios are an ideal target for a new generation of hyper-converged, edge-optimized infrastructure products.
As the amount of remotely generated data increases, the operational efficiency of a hub computing architecture is outweighed by the cost and overhead of backhauling more and more data. Even adding a few edge locations can significantly reduce an organization's WAN link count, as well as network and computing bottlenecks.
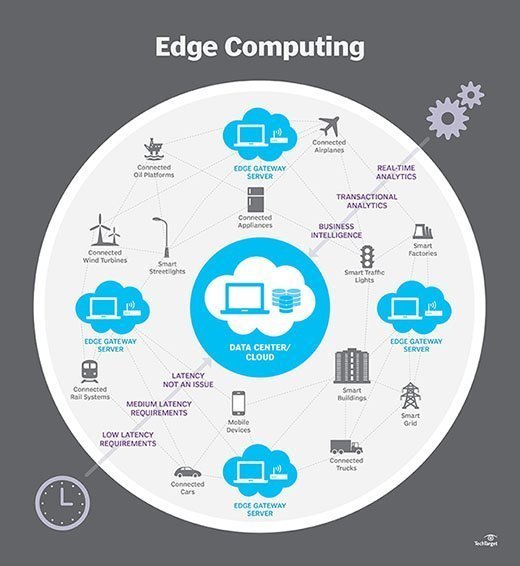
Why hyper-converged computing at the edge?
Edge computing architecture covers many different scenarios, workloads and capacity requirements. Organizations will find hyper-converged systems attractive options for a large swath of mainstream situations -- i.e., all but the largest and smallest capacity requirements. Here's why:
- high density of compute and storage resources in a self-contained, easily deployed platform;
- integrated hardware and software stack that can be preconfigured and deployed by minimally skilled personnel and remotely managed;
- support for many enterprise and service provider software stacks, including VMware, Microsoft Azure Stack, OpenStack, Red Hat OpenShift and bare-metal Kubernetes clusters;
- scalable system architecture user can expand both internally by adding compute nodes, memory and storage modules and horizontally by adding multiple hyper-converged infrastructure (HCI) units in a distributed, centrally managed cluster; and
- diversity of hardware options, including models designed for harsh physical and environmental conditions.
Let's explore some typical edge computing usage scenarios, along with some hyper-converged products appropriate for each.
Large installations
Perhaps the situation driving the most intense interest in edge computing is related to support of 5G wireless networks and mobile customer workloads. As wireless bandwidth scales to gigabit and faster speeds and the number of wireless devices continues to increase, centralized infrastructure won't be able to keep up with the demands from resource-intensive applications. Dropping mobile edge computing nodes in cellular base stations, office parks, and large office and apartment complexes is critical to offloading workloads and maintaining adequate performance.
Although some wireless installations will be large enough to warrant multiple racks of equipment, smaller cells -- of which there will be many given the limited range of 5G frequencies -- can be accommodated by hyper-converged systems. Other situations warranting one or more hyper-converged edge devices are remote worksites, such as manufacturing facilities, mines, oil production sites and warehouse distribution centers.
Most traditional IT server suppliers offer HCI products suitable for large installations. The products are similar -- typically, a 1U or 2U chassis housing one, two and, sometimes, four dual-socket compute nodes with between four and 16 small form-factor storage bays. Some examples include the following:
- Cisco HyperFlex Edge;
- DataCore HCI-Flex;
- Dell EMC XR2 Rugged Server;
- Dell EMC Micro Modular Data Centers;
- Dell EMC VxRail line running VMware software stack;
- Hewlett Packard Enterprise (HPE) Edgeline Converged Edge System;
- Nutanix (hardware available from many partners, including ruggedized devices from Crystal, HPE, Klas Telecom and Mercury Systems); and
- Scale Computing HC3.
Many OEMs, like Supermicro, Inspur, Quanta and others, also offer hyper-converged systems that can run any software stack users require. These are functionally identical to enterprise HCI products from Dell EMC, HPE and Nutanix but without the bundled virtualization platform.
Small sites: ROBO/remote manufacturing/hardened
Situations suitable for smaller, single or dual-node HCI hardware include remote offices/branch offices (ROBOs), smaller manufacturing or production sites, medical clinics and call centers.
HCI is particularly useful in locations using virtual desktop infrastructure workstations or with equipment and IoT devices generating copious amounts of data. It is more efficient to analyze and filter this data locally than send massive data streams to the cloud or an on-premises data center for analysis and filtering.
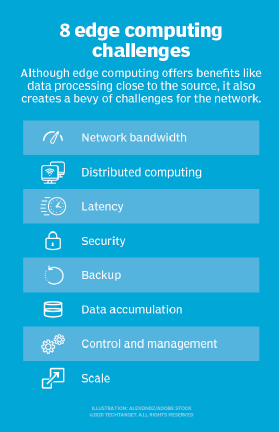
While organizations can appropriately size many of the hyper-converged products listed above for these uses, others in smaller form factors or packaged as ready-to-deploy appliances might be a better fit, including the following:
- Dell Edge Gateways and other Intel Atom-based small form-factor systems;
- Supermicro embedded/IoT solutions; and
- Nvidia Jetson hardware products with embedded GPUs on a SoC -- available from many OEMs, these are used for edge data analysis using machine learning and deep learning algorithms.
Recommendations
Organizations facing an explosion of data generated by remote sensors; smart, connected manufacturing equipment; and other sources will discover the wisdom of offloading data processing from central data centers and cloud infrastructure. Similarly, those with numerous remote locations and employees will find the ability to remotely manage hyper-converged systems an attractive option in situations with no local IT personnel and an improvement over backhauling network traffic every time users need to run an enterprise application or access a database.
Moving compute resources to the edge is proving more efficient and cost-effective than a centralized architecture in these situations and other emerging scenarios and use cases, such as the emergence of 5G infrastructure. The compactness, convenience and wide variety of hyper-converged hardware and software products make hyper-convergence an ideal platform for such edge deployments.