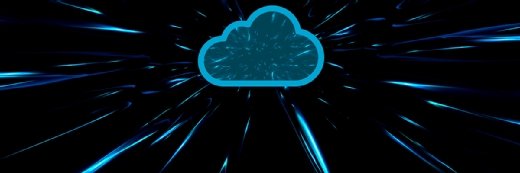
Getty Images/iStockphoto
How will AI apps impact workloads in the cloud?
Building AI apps in the cloud requires you to pay more attention to your cloud workload management because of how AI impacts performance, scalability and compliance.
AI and cloud are becoming more intertwined as enterprises embark on AI development initiatives. Examples might include an insurance company building an internal application to detect fraud, a recruiting firm building an AI tool to manage candidates with job requisitions or a manufacturer building a predictive maintenance system.
However, enterprises can't just adopt AI technology without any preparation -- AI can bring positive and negative changes. Here's a closer look at how AI will change how you manage cloud workloads.
Positive impacts of AI on cloud workloads
Cloud workloads offer AI applications several benefits, including the following.
Resource optimization
Resource optimization is critical to AI applications, and the major cloud providers, such as Amazon, Microsoft and Google, give you services to optimize resource usage by dynamically allocating compute power and storage based on workload requirements. For example, autoscaling is integral to resource optimization.
Workload efficiency
As your developers acclimate to AI development, they can apply their lessons learned to optimize compute power and storage to meet workload requirements for workload efficiency. Your cloud management platform provides the actionable data you need to know about the increased resources your AI applications might consume. Standard practices, such as reducing waste and improving overall performance, are even more critical than deploying traditional applications.
Reduced infrastructure costs
Building the on-premises infrastructure to support AI applications is quite an investment, even if your company has a big budget. Going with AWS, Microsoft Azure and Google Cloud for AI services is economical because the pay-as-you-go pricing model is well suited to the experimentation, learning and impending scalability requirements your organization will have for its AI applications in development.
Increased data analytics
The cloud has been responsible for putting enhanced data analytics in the hands of more corporations and government agencies without prompting an expensive infrastructure and tools buildout. AI in the cloud can process and analyze vast amounts of data quickly and accurately. Cloud-based analytics tools enable teams and stakeholders of varying skill levels to collaborate on projects from anywhere.
Negative impacts of AI on cloud workloads
While beneficial, AI integration into cloud workloads also brings several negative impacts that your organization must address proactively.
Environmental impact
AI workloads increase cloud providers' data center energy consumption and environmental impact because they require significant computational power, driving high energy consumption. The major providers are undertaking sustainability initiatives, including optimizing cooling systems and energy-efficient hardware to reduce their carbon footprint. The increased cooling requirements of data centers also lead to significant water consumption, which is increasingly concerning given global water scarcity issues.
Increased security risks
AI workload security requires tools and practices, including encryption and identity and access management. Major cloud providers bring all those security tools to one dashboard, but the shared responsibility model means teams in your organization are responsible for configuring and managing security tools properly. The large volumes of personal and corporate data that AI systems often require raise significant privacy concerns, so AI cloud applications must be designed to be secure against cyberattacks and data breaches.
AI workloads also raise the complexity of compliance, adding real or imagined concerns to meeting GDPR, HIPAA and other compliance programs. Depending on your third-party auditor, its AI risk mitigation expertise might only be a little bit ahead of your own developers working on their first AI project headed to production.
Increased costs
Being in cloud isn't enough to save money -- businesses need to evolve their cost management strategies. Even if your organization is fortunate enough to have mature FinOps practices, AI workloads will break from your previous spending trends unless you involve your FinOps team early in your AI initiatives. The increase in cloud spending that AI workloads bring may price some small companies out of AI development for the time being.
Bias concerns
Bias and ethical concerns about AI persist, especially in financial services and healthcare, where AI models may perpetuate discriminatory outcomes. California and Colorado have passed laws to prevent AI discrimination in hiring, and it's safe to expect more regulations in the future.
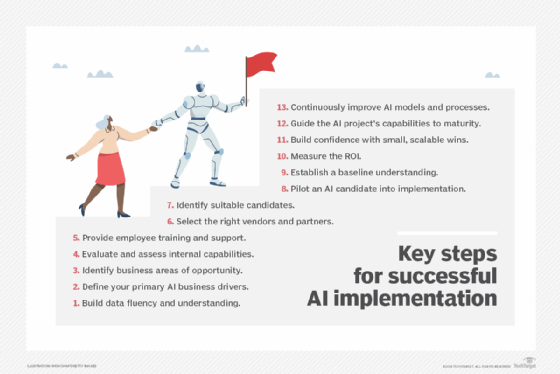
Prepare for AI apps
Preparing your cloud management and operations for the addition of resource-hungry AI applications and services may require taking a step back to revisit your corporate cloud strategy. Some core actions should include the following.
Educate staff
AI hype can be all-encompassing, from the most junior employee to your C-suite. Prepare to educate and set expectations about AI across your organization. You can do this by scheduling pilots or proofs of concept that tackle your problems where AI can be part of the solution. "AI needs to solve business problems" must become your mantra.
Prioritize collaboration
Your organization also needs to prepare for the increased compute power that AI requires, which may impact your current cloud architecture and spending. Bring your cloud and FinOps teams together early in the design process to address this question, which can save your organization's technical problems and sticker shock later. Additionally, evaluate cloud cost management platforms to ensure they support AI workloads.
Consider containers
Move your AI development efforts to containers to set your AI projects up for maximum flexibility. The increased portability enables you move AI workloads across providers to find the best platform to experiment with securely and within budget, all while not impacting your current cloud environment.
Review your security strategy
Thoroughly review the in-place security protocols and data protection strategy. Presumably, AI applications will bring more of your corporate data to the cloud, which will trigger changes to your security and data protection practices.
Will Kelly is a freelance writer and content strategist who has written about cloud, DevOps, AI and enterprise mobility.