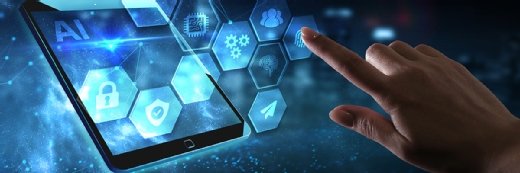
putilov_denis - stock.adobe.com
Benefits and drawbacks of AI in cloud computing
AI and cloud computing work hand in hand to improve upon various processes, but the combination of these technologies comes with a price.
The era of artificial intelligence is already upon us, and it's found its home in the cloud.
Artificial intelligence (AI) assists in the automation of routine activities within IT infrastructure, which increases productivity. The combination of AI and cloud computing results in an extensive network capable of holding massive volumes of data while continuously learning and improving.
With tools available from top public cloud providers, such as IBM Watson, Google Cloud Vertex AI, Microsoft Azure AI and the AWS AI services portfolio, advanced AI and machine learning capabilities are more readily available to enterprises. Learn how AI can benefit your enterprises, as well as areas to watch out for.
The benefits of AI in cloud computing
AI has changed the cloud landscape in the following ways:
Lower costs
A big advantage of cloud computing is that it eliminates costs related to on-site data centers, such as hardware and maintenance. Those upfront costs can be prohibitive with AI projects, but in the cloud enterprises can instantly access these tools for a monthly fee, making research- and development-related costs more manageable. Additionally, AI tools can gain insights from the data and analyze it without human intervention.
Intelligent automation
Enterprises use the power of AI-driven cloud computing to be more efficient, strategic and insight-driven. AI can automate complex and repetitive tasks to boost productivity, as well as perform data analysis without any human intervention. IT teams can also use AI to manage and monitor core workflows. IT teams can focus more on strategic operations while AI performs the mundane tasks. For example, IBM Cloud Pak for Automation provides prebuilt workflows for AI-powered automation.
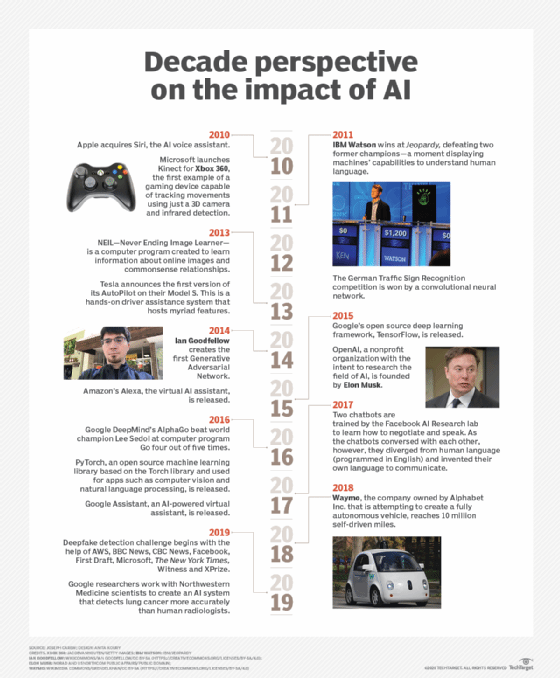
Deeper insights
AI can identify patterns and trends in vast data sets. It uses historical data and compares it to the most recent data, which provides IT teams with well-informed, data-backed intelligence. On top of that, AI tools can perform data analysis fast so enterprises can rapidly and efficiently address customer queries and issues. The observations and valuable advice gained from AI capabilities result in quicker and more accurate results. For example, an app developer can use Amazon Personalize to give customers real-time personalized recommendations.
Improved data management
AI plays a significant role in processing, managing and structuring data. AI can significantly boost marketing, customer care and supply chain data management with more reliable real-time data. AI tools streamline how data is ingested, modified and managed. For example, IT teams can imbed AI tools into Google Cloud Stream analytics to get real-time personalization, detect anomalies and predict maintenance scenarios.
Increased security
As enterprises deploy more applications in the cloud, intelligent data security is crucial to keep data safe. IT teams can use AI-powered network security tools to track and evaluate network traffic. AI-powered systems can raise a flag as soon as they find an anomaly. This proactive approach helps prevent any damage to critical data. For example, Amazon GuardDuty is an intelligent threat detection tool that uses AI and machine learning to find potential risks.
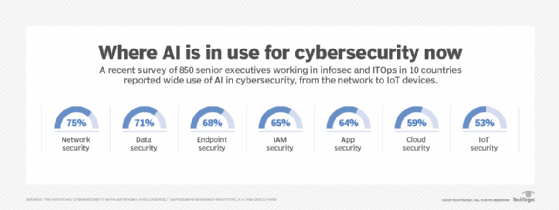
The downsides of AI in cloud computing
While combining AI with cloud computing provides numerous benefits, there are drawbacks as well. For example, while it can lower costs, AI is complex and may require a well-trained staff, which can cost more money upfront. The following are the other downsides to using AI in cloud computing environments:
Connectivity concerns
Cloud-based machine learning systems need consistent internet connectivity. IT teams use the internet to send raw data to the cloud service and recover processed data. Poor internet access can hinder the advantages of cloud-based machine learning algorithms.
While processing data in the cloud is quicker than conventional computing, there is a time lag between transmitting data to the cloud and receiving responses. This is a significant issue when using machine learning algorithms for cloud servers, where prediction speed is one of the primary concerns.
Data privacy
AI applications require a large amount of data, which can include consumer and vendor information. For example, Amazon provides recommendations based on purchase history. While some data can be anonymous and can't be tied to personally identifiable information, knowing who the data belongs to makes it more valuable. When sensitive information is used, data protection and compliance is a major concern. Enterprises need to create privacy policies and secure all data when using AI in cloud computing.