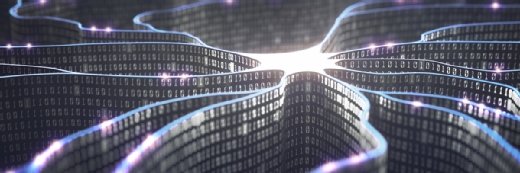
ktsdesign - stock.adobe.com
Understand AI and machine learning security impacts on cloud apps
Cloud-based machine learning can alleviate and aggravate security concerns. Review analysis and advice from our experts to stay on top of this emerging aspect of IT.
AI's security impacts on cloud computing are twofold: The technology has the potential to make workloads more secure but opens the door to new threats.
This dichotomy arose, in part, because AI and machine learning are increasingly infused into the major public cloud platforms. Cloud providers and third-party vendors offer a range of AI services that target novices and seasoned data scientists -- some of which could present new machine learning security challenges. In addition, some vendors offer security services that rely on AI to identify potential dangers.
This deluge of AI and machine learning security tools -- and potential threats -- can overwhelm users, especially since most organizations have only just begun to dabble in these technologies. Here are four expert tips to get users up to speed on the interplay among cloud computing, security and AI.
Cloud vendors add AI to security tools
Organizations can't directly access some of the most intriguing uses of AI in cloud computing because those algorithms are baked into managed security services.
These tools generally come in two forms. The first uses machine learning to scan user records to identify and classify sensitive information. The major cloud providers and third-party security companies, such as FixStream, Loom Systems, Devo and ScienceLogic, have some variant of this.
The second variant uses machine learning for threat detection. Cloud security services, like Amazon GuardDuty and Microsoft Azure Sentinel, take advantage of the providers' vast networks to identify common threats and alert admins of potential risks.
The tools from public cloud providers, however, have one major disadvantage: They don't work on other vendors' clouds. This can be a problem for organizations that want a uniform security posture across a multi-cloud architecture.
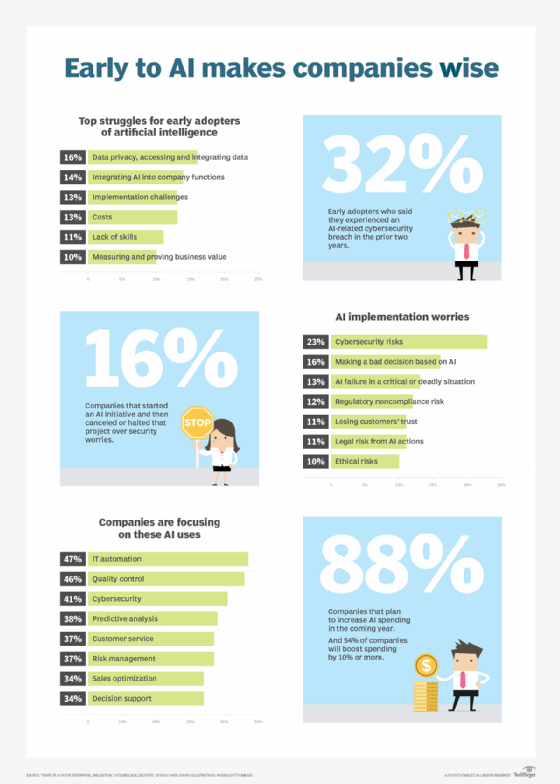
Threats from machine learning-backed attacks
Security experts have warned of an arms race between those that want to use AI and machine learning for good and those that plan to use it for nefarious purposes. As these technologies advance, bad actors could use machine learning to adapt to defensive responses and undermine detection models to find vulnerabilities quicker than they can be patched.
Machine learning requires massive amounts of data to be effective, which is why much of that work is being done on the cloud today. Companies can quickly provision resources to handle their computation demands. However, the volumes of data needed to train and run models can increase privacy concerns as companies collect data about user behavior. To mitigate these risks, companies can anonymize user data. They'll have to remain cognizant of data residency requirements and other transparency regulations.
Going forward, cloud vendors also may do more to build security features directly into their AI and machine learning tools as they become more popular. For example, Google recently added TensorFlow Privacy, a variant of its popular TensorFlow machine learning framework. It uses differential privacy techniques to improve a model's security.
Machine learning security practices through automation
Public clouds are great for scaling rapidly, but operations at a massive scale introduces complexity. Organizations can struggle to keep tabs on the large volumes of logs produced by their resources, as well as the scores of users they may have spread across their accounts. Failure to keep tabs on those activities can lead to vulnerabilities.
Organizations can get a better handle on this surge of information when they remove as many manual steps as possible. AI and machine learning can take those efforts a step further and automate the automation.
AI is no silver bullet
Of course, AI isn't a panacea for security threats, despite the fact that many organizations expect it to be. For starters, algorithm outcomes are only as good as the design and the data that goes into them. They can also be manipulated and misrepresented. Organizations need to be aware of the limitations of these technologies as they emerge.
More importantly, proper cyber-hygiene starts with the people in a company, not the technology. AI and machine learning can bolster security efforts, but companies should focus first on educating employees about proper security practices.