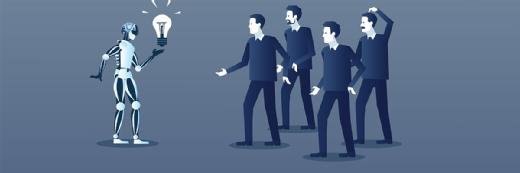
5 ways CIOs can take their AI project to the next level
We've covered the basics for CIOs getting started with AI -- now, we're taking a look at the potential challenges they may face and how they can prepare for and mitigate them.
A study by Deloitte suggests that in the coming years, AI will be a part of every brand and industry, as those that did not embrace the change will fall out of the race. Recently, we covered the AI mindset -- specifically a primer on how to get the right mindset to shift your company to an AI-driven business. Having covered the basics, we now have a look at the next steps: the real-world challenges and hurdles companies with AI need to consider in order to take their AI project to the next level.
1. Agree on the metrics
You should always have reasonable expectations from AI -- this cannot be stressed enough. Just recently, former employees at Engineer.ai revealed that the startup company had exaggerated its AI capabilities. There have also been numerous cases where Microsoft, Facebook, Google and Apple came under fire because their contractors listened to audio recordings from their users.
The reason for warranting these expectations is that current AI systems are not smart enough to operate at the levels advertised by those companies, which forces them to fill the gap with human labor. Setting realistic expectations is a key factor to ensure your AI project succeeds.
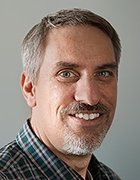
That being said, your goals must also be clearly defined. "The problem I often see is that the business side of the company and the AI side of the company may not agree on precisely what this value definition should be," explained Dean Abbott, co-founder and chief data scientist at SmarterHQ, a personalized interactions company. "For example, I've spoken with several AI teams about the failure that ensued the first time they built churn models," he said. They build an excellent predictive model in three months, but when they report back to the business stakeholder, the stakeholders discover that the data science team's definition of churn -- a shopper who canceled his or her membership -- is unworkable because the business needs a three-month lead time to try to recover the churning customers. "This operational disconnect happens very often with companies new to predictive modeling," Abbott concluded.
2. Agree on what to optimize
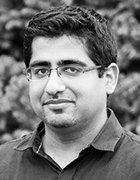
As with every new and particularly hyped technology, a common pitfall is constructing an AI project in search of a problem. "Many times, people start with the technology, but we strongly believe in starting with the problem and that helps to know exactly where to start," said Sid Bhambhani, CEO of Summatti, a customer management platform. "For example, one of our customers was seeing an increase in customer churn and there was a lot of finger-pointing going on in their organization. This customer was looking for ways to understand the root cause of the churn," he said. Using AI, they were able to get a data-driven and unbiased answer.
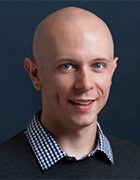
It's also worth paying attention to AI's sweet spot. "Most, if not all business processes, tend to include a number of simple, yet time-consuming tasks such as the identification of keywords in documents," explained Lane Lillquist, CTO and co-founder of InCloudCounsel, a legal technology company. "These activities are low value relative to the human resources normally staffed to facilitate such tasks," he said, making them an ideal target for AI automation.
3. Agree on what data to collect
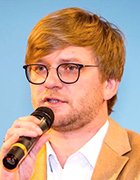
While data is the lifeblood of any AI application, it cannot be collected recklessly. As Ruslan Gavrilyuk, co-founder and president of TeqAtlas, summarized, data must meet three key characteristics:
- Data extraction must not break any law. Companies must adhere to privacy regulations and restrictions on how data may be obtained.
- CIOs should see value in the data and know how to utilize it.
- The data extraction and processing costs should not exceed potential revenue.
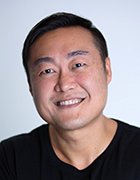
The reliability and storage of the collected data is also important, as malicious actors sometimes target the AI's underlying data system, injecting errors, bias or missed red flags into the algorithm. "Companies need to monitor the data entering the system and flag fraudulent or incorrect cases," said Cosmas Wong, CEO of GNY.io, a company that offers a machine learning platform for blockchain applications. By making use of blockchain, companies can effectively track who accessed or altered the data, thus being able to track down bad data and the root causes.
4. Test and retrain
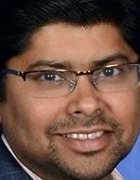
AI, by nature, offers little insight into what is going on under its hood. Therefore, it can be challenging to utilize for critical applications. "The risk can be mitigated to a certain degree through comprehensive testing to ensure that predictions are being made accurately," explained Sanjeev Pulapaka, the AI competency lead at REI systems. "Several organizations invest in months of parallel testing, comparing results obtained by an AI model with actual results, and tuning the model to improve results," he said.
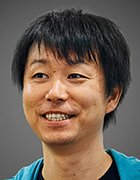
Testing the AI is particularly important, as an algorithm that worked in the lab might not work as well once it's rolled into production. "AI is all about trial and error, so often times what seemed like a good idea [in] the beginning turns out to have little real-world value," said Dr. Ryohei Fujimaki, founder and CEO of DotData. "That's why rapid iteration with automation is key." To cope with this challenge, Fujimaki stressed on the significance of being able to retrain the AI model.
There are cases where problems with the AI's decisions do not reveal themselves long after the system has run into production. "One of the biggest issues lies in the area of bias in algorithms," Pulapaka claimed. "Any data that is exposed by human beings inherently contains some bias that skews the results." Continuous testing and retraining can help to eliminate such misconfigurations.
5. AI development automation
Testing and retraining AI models, as well as data cleaning and feature extraction, are all time-consuming processes. To solve this, AI experts borrow from the development operations that are common in software development and they automate the processes. "DevOps focuses on continuous delivery by leveraging on-demand IT resources and by automating test and deployment of code," said Chris Bergh, CEO of DataKitchen, a consultancy and platform provider. "DataOps brings these same improvements to data analytics," he said.
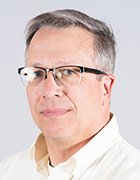
In simple terms, DataOps is about automating every step of the AI training and development pipeline by addressing problems quickly and early on.
"DataOps catches problems early in the data lifecycle by implementing tests at each pipeline stage," Bergh continued. "If an anomaly occurs, the data analytics team will be the first to know through an automated alert." As Wong proposed, machine learning can be used in this process to detect abnormalities.
Wrapping up
While AI can transform any business, it is an emerging technology that can often either meet unreasonable expectations or create problems that are underestimated. CIOs need to have the right expectations of what AI can do and understand the problems related to implementing and maintaining an AI project to properly transform their company to an AI-fueled business.