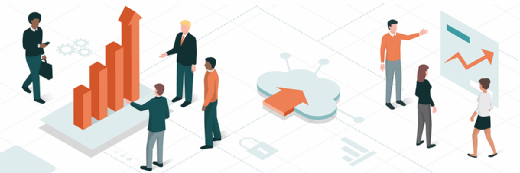
Turning data into actionable insights with machine learning
To help make better use of data and analytics, we're diving into how companies can infuse ML-produced actionable insights into their decision-making workflows.
It's no secret that businesses are data-driven these days. With the rise of data collection, analytics has become one of the biggest drivers of business value. However, an analytics program is only as good as the workflows underneath it.
While companies are collecting more data than ever before, many struggle to figure out what to do with it. Businesses need to use actionable insights to drive decisions, but what is an actionable insight, and how exactly is data used to create one?
What are actionable insights?
An actionable insight is a process that is derived from raw data analysis. For example, business leaders could track a customer's behavior on a platform that can indicate their sentiment regarding a product. These data points are fed into an analytics platform, from which users can derive conclusions.
Business leaders might notice that customers aren't as keen on a product feature that was popular in the past and that they're asking for a new feature or enhancement. Social media listening strategies also help to gather raw data and turn them into actionable insights.
Every industry could use actionable insights. "Actionable insights arising from analytics and AI are no longer a luxury, but a necessity for achieving competitiveness," said Eitan Sofer, head of developer platform at Sisense, an analytics provider.
Information by itself isn't an actionable insight. If the information a company receives enables stakeholders to make decisions, modify current processes or overhaul them, then that's an actionable insight.
These days, analytics platforms rely on AI and machine learning (ML) algorithms to drive actionable insights. For example, a financial company can use an ML algorithm to quickly assess a loan applicant's credit-worthiness and provide a human agent with a recommendation to approve or disapprove the loan.
While the human agent still has discretion over the process, the algorithm makes their job easier and more efficient.
Preparing data for analysis: Structured and unstructured data
While ML algorithms offer great insights, they're fully dependent on the data they're fed during their learning and maturity stages.
"Analytics must cope with both structured and unstructured data to achieve optimal results," Sofer said.
One of the main challenges of generating actionable insights is the need to deal with both structured and unstructured data.
Analyzing structured data is straightforward. This data can be customer or user-generated and is present in predefined templates. For example, customer information forms are a source of structured data since they have predefined fields that customers enter information into. Structured data is housed in a traditional database and as a result, is highly schema-dependent.
Unstructured data is more challenging. Examples of this data include raw text, social media comments, log files and call transcripts. Unstructured data needs to be stored in a NoSQL database or a data lake, is free-form and doesn't adhere to schemas.
Unstructured data, by some estimates, comprises 80% of all available data, and using a powerful business intelligence (BI) platform can simplify turning this data into actionable insights. A platform that connects to multiple sources will make it easier to import disparate data and create efficient workflows.
Turning data into actionable insights
Actionable insights aren't the only kind of insight an analytics platform can provide. For example, a company may receive confirmation of a business strategy that requires no further action. These insights are just as valuable as actionable ones.
It's important to formulate business questions before turning to an analytics platform to determine which insights are actionable and which ones are not. Which issues are you trying to solve, and how will you measure success? It's also helpful to establish the context of the dataset before accepting the insight as valid.
Every data collection method has bias within it. For example, log files could indicate that customers spend a lot of time on one particular function within the platform. The company may conclude that this is the most important feature and work to enhance it. However, the customers could be spending time there because the feature is inefficient or unintuitive.
Always pay attention to the context in which the data was collected. "Tunnel vision is a problem across a variety of fields, from sports to industry," said George Walker, managing director at Novotek U.K. and Ireland. "It is easy to fixate on a single goal and forget to take a step back for a wider view of the situation. Yet taking this step back can provide invaluable insight and understanding of the reality of a situation."
Once the data's context is established, construct hypotheses to test. Many organizations measure data and then wander through the results looking for some magical insight. Establishing a hypothesis and testing it for accuracy will deliver actionable insights much faster.
Infusing insights into workflows to assist in decision making
"Modeling complex and multidimensional data parameters in innovative ways and presenting those insights within workflows will change the future of every industry," Sofer said.
However, the future can change only if insights are used to drive decision-making.
Many companies derive insights but neglect to act on them. To take full advantage of a BI analytics platform, an organization must create an optimization plan. A Six Sigma action plan can help integrate new ideas and findings into workflows, define the problem and measure the effectiveness of the current process.
Use the BI platform to analyze the root causes of these issues and make improvements to processes. Once implemented, measure and control the new process for effectiveness.
Integrating insights into workflows is a cultural matter as well. Educate employees and let them know that analytics augments their jobs and isn't intended to replace them. That way, an organization can see greater adoption of data-driven insights into everyday business processes.
It's also important to democratize the BI platform so that everyone, including business users, can run ad hoc reports to derive insights. The company will increase the number of actionable insights exponentially by breaking down silos like these.
Negating all those guesses
Actionable insights directly affect an organization's bottom line by increasing conversions and as a result, sales. Lower churn will accompany higher revenue numbers since business leaders will have greater insight into what drives the customers' value question.
The biggest benefit is an organization's ability to move from hunches and "feelings" to backing up decisions with hard data. No more will business leaders have to take shots in the dark and hope a new process works.
Creating actionable insights is a process like any other, and it's perhaps the most important factor for a business' overall success.