Deep learning algorithms power startup's beauty database
Deep learning algorithms are changing how we drive cars and navigate outer space. What about saving our skin? Silicon Valley startup Proven Beauty has a plan.
As someone who lives in Silicon Valley, Ming Zhao said it's hard not to notice how AI is being used to transform big industrial products like cars and rockets. But where was AI when it came to things that aren't quite so heavy-duty?
"What about products that I find absolutely essential to my everyday life, such as skin care?" Zhao said, referencing the largest segment of the $445 billion beauty industry. Surely, AI had a role to play in products that 90% of women claim to invest in.
Zhao is CEO and co-founder of the startup Proven Beauty. She and her business partner, Amy Yuan, have assembled what they claim to be the biggest beauty database in the world. It is composed of 14 different data sources such as peer-reviewed journals, skin care ingredients, customer reviews and community chat boards. It's currently a quarter of a terabyte in size, and it's using cutting-edge technologies such as natural language processing and deep learning algorithms to provide a personalized line of skin care products to its customers.
"We've nicknamed this beauty database the beauty genome project," Zhao said. "Our mission is to become the preeminent voice of truth in beauty."
AI in the age of the customer
Today's cosmetics customers don't shop like they used to. According to a recent global beauty and personal care trends report by the market intelligence firm Mintel Group Ltd., customers are doing more research than ever on cosmetic products before they make a purchase, increasingly seeking out companies that reflect their values when it comes to things like animal cruelty, climate change and social justice.
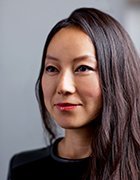
They also tend to be less brand loyal in general, according to a January report by Forrester Research on luxury retail. Zhao has found that attitude to be true with skin care products as well. Rather than rely on the advice of the sales associate behind the beauty counter at their favorite department store, customers are spending more time reading customer reviews, she said.
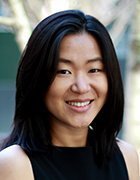
Indeed, Zhao said she and Yuan and most of their girlfriends purchase skin care products by conducting amateur R&D: They scour reviews, find testimonials from customers they relate to and make choices based on the information. The problem is the information is sprawling and potentially unreliable, according to Zhao.
"The idea that Amy and I had was why don't we use machines to read reviews so the average woman doesn't have to spend an hour to two hours before buying every single product," Zhao said.
Zhao said the Proven Beauty database includes 8 million reviews on 100,000 products and 20,000 ingredients. Proven Beauty is also capturing unique data through its customer quiz, which asks personal questions like how many cups of water the quiz-taker drinks a day and what a person's skin goals are. It uses the data to construct knowledge graphs, which are graphs of semantic data sets that highlight the relationships between the data.
Deep learning algorithms, semantic search, NLP to the fore
To do that, Proven Beauty uses what Zhao called the "three main pillars of technology." To collect the data, Yuan, co-founder and CTO at Proven Beauty and a computational physicist from Stanford, built millions of automated bots that continuously crawl and scrape data such as ingredient lists, product names and customer reviews from e-commerce platforms.
Next, Proven Beauty uses semantic search to extract information from customer reviews such as the product name, any skin concerns the customer may have and any data on the customer's experience with the product. The data is a mix of structured data such as timestamps and product information, as well as unstructured data such as text. To determine the sentiment captured in the text, Proven Beauty relies on natural language processing, that is, a program that's able to understand language. The data is then used to construct a consumer knowledge graph, which connects what reviewers say about a product to their personal characteristics, according to Zhao.
Advice from CEO Ming Zhao
Zhao's one piece of advice to CIOs who are planning to invest in artificial intelligence is "to find the best people you can find to get the job done." Proven Beauty's competitive differentiator is its beauty database, which took two years to construct. Zhao said it was her co-founder, Yuan, who did the yeoman's work of building the beauty genome project, often tapping experts at Google Brain and other tech companies to guide their progress.
"Semantic search is doing the same thing with the ingredients of the products that people are talking about," she said.
Finally, Proven Beauty is combining the consumer and the ingredient knowledge graphs and using machine learning and deep learning algorithms to determine the efficacy of an active ingredient within a product against skin concerns, demographic, geographic and genetic data.
"It's a pretty difficult problem," Zhao said. One aspect that makes the problem especially complex is that active ingredients don't operate within a vacuum. Instead, they require a network of inactive chemicals such as preservatives and other active ingredients to work. To pinpoint and understand the relationships between an active ingredient and a sub-segment of women, Proven Beauty is unleashing deep learning algorithms onto the data, she said.
"It is a major project for what seems to be a more everyday industry," Zhao said. "But I think that's exactly what is needed, especially for products that we women care about."
Based on the information provided from the Proven Beauty quiz, customers are sent a skin profile, which includes a visual breakdown of their skin type, as well as a list of customized skin care products.
The Proven Beauty database part of a trend
Proven Beauty, which plans to officially launch at the end of the month, is not alone in applying AI and deep learning algorithms to the cosmetics industry. Established players like Olay are also experimenting with it. Last year, the company launched a skin advisor that uses deep learning algorithms to analyze makeup-free selfies and make product suggestions. And new players such as ModiFace are bringing augmented reality to the beauty industry, enabling customers to digitally "try on" different makeup looks.
Indeed, in its 2018 global beauty and personal care trends report, Mintel advised the beauty industry to embrace cutting-edge technologies by developing apps that operate like digital assistants, experimenting with voice-related features, and utilizing biometric data such as eye tracking and body language to create a more personalized shopping experience. The report also warns that doing so will open the industry up to modern-day struggles such as data privacy and security.
Still, Forrester analyst Sucharita Kodali said Proven Beauty's approach is unique. "They're harnessing big data, kind of like what Stitch Fix is doing for apparel, to create a beauty genome," she said. Whether Proven Beauty survives is yet to be seen, she added, but the market segment is a healthy one.
"This is the selfie generation," she said. "And when you take selfies, you want to look good."