The future of trust will be built on data transparency
In a digital economy fueled by AI, data transparency is the new currency. Learn what IT leaders must do to bring trust and transparency to the enterprise, now and into the future.
At all levels of society, the foundations of trust are being tested like never before. Under siege by a deadly pandemic, people are questioning the trustworthiness of hallowed institutions, from governments and law enforcement agencies to scientific research organizations, media outlets and schools. Enterprises know they are not immune to the prevailing wave of distrust.
Now, perhaps more than ever before, businesses in every industry are looking at trustworthiness as a competitive differentiator. But what does the future of trust look like in a marketplace where digital data -- its collection, representation, analysis and application -- increasingly drives business models?
At present, experts point to privacy legislation like GDPR and other new regulations as having a significant impact on corporate data practices.
"Organizations have shifted from focusing on collecting as much individual data as possible to enable personalization toward minimizing the level of individual information they have to maintain, because data now represents risk exposure," said Brian Burke, research vice president for technology innovation at Gartner and lead author of the firm's Hype Cycle for Emerging Technologies.
But the task ahead for business and IT leaders in providing data transparency and trusted data is bigger than complying with regulations like GDPR or limiting data collection, Burke and others argue.
"Trust is more than just about mitigating harm but also maximizing return, creating a differentiated impact on revenue, expenses and shareholder value," wrote Frank Dickson, program vice president and IDC's global lead for Future of Trust research.
IDC predicted that by 2023, 50% of the Global 2000 will name a chief trust officer, who orchestrates trust across business functions including security, finance, HR, risk, sales, production and legal. And by 2025, two-thirds of the Global 2000 boards will ask for a formal trust initiative with milestones aimed at increasing an enterprise's security, privacy protections and ethical execution.
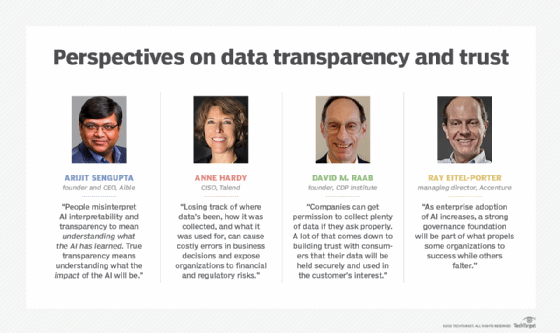
Tackling data trust and transparency
For CIOs and other IT leaders, getting enterprises to this future state will require an immediate focus on the trustworthiness and transparency of their enterprises' data. Gartner's Burke predicted that in 2021, IT leaders will be asked to explore such thorny areas as confidential computing, a catch-all term for technologies designed to maximize security, limit the use of personal data and give individuals control over their data.
The push will increase for explainable AI, or AI that is programmed to describe itself in terms an average person can understand. And as AI becomes fundamental to understanding and capitalizing on data, companies will also have to ensure trustworthy AI, the concept of AI systems that are resilient in the face of change, protect people's privacy and are secure.
Enterprises will need to approach trust in ways that go beyond vetting a single aspect of data management, such as data provenance, governance or compliance. They will have to win over consumers by demonstrating how exactly their data is being used. Likewise, employees, management and regulators will need to be convinced that the data collected and used to make important business decisions is trustworthy. Down the road, successful companies will engage consumers and employees as partners in developing new data-driven business models and new work models.
As with IT security, the chain of trust for enterprise data initiatives is only as strong as its weakest link. In addition to the human element, establishing a chain of trust for data will involve the use of encryption to securely share analytics in AI models, along with a raft of new technologies -- and buzzwords -- including homomorphic encryption, trust fabrics and federated deep learning.
This article explores a paradigm change in the way enterprises -- and ultimately all of us -- manipulate, manage and get the most out of data. It begins with the need for enterprises to get a better handle on AI and ends with a glimpse of a future where our digital identities have agency and certain unalienable rights.
AI's role in the future of trust
The growing use of AI to improve decision-making is driving the need to understand how algorithms work, why they came to a decision and what their limitations are. This is required not only to build trust with business managers about the value and reliability of the AI, but also to get approval from regulators. Unlike for the early enterprise deployments of AI used for low-stakes applications like translating languages, the bar is higher now, said Gartner's Burke.
"Now that AI is being applied to more consequential applications, explainability becomes a big deal," he said.
Bias is the biggest practical challenge in implementing AI algorithms, argued Sachin Vyas, vice president of data, AI and automation at LTI, a digital transformation consultancy. Unless stakeholders can peer inside these algorithms, they won't be able to tease apart whether bias exists, what might be causing it and how it can be mitigated.
"It is essential that we question how and why the AI recommended what it recommended; hence, transparency and impartiality in AI is essential to building trust," Vyas said.
CIOs and other IT leaders must pay attention to the purpose and goals of the AI system and not fall back on a common but specious line of defense: namely, that the algorithm is highly sophisticated, but the data is biased. Real-world data will be always biased, Vyas said, because it is situational. It is the responsibility of technology experts to fully understand the context behind the data and then design algorithms that support human values, he said.
Knowing the real-world impact of AI
It's important to go beyond the mechanics of the algorithms to understand their real-world impact.
"People misinterpret AI interpretability and transparency to mean understanding what the AI has learned," said Arijit Sengupta, founder and CEO of Aible, an AI platform. "True transparency means understanding what the impact of the AI will be." Knowing how AI will affect the business or customers is as important as knowing what the AI has learned.
Another challenge in AI is tracing the source of degradation in model performance, said Justin Silver, manager of data science at Pros, an AI-powered sales management platform vendor. When the performance of an AI model drops, transparency -- understanding how the algorithms, data and usage connect to the model's results -- can help to identify the cause and guide data scientists in building a better model.
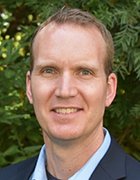
Transparency becomes even more of a challenge with algorithms designed to continually learn in response to new data using reinforcement learning techniques. If the AI was not built to allow data scientists to see why it changed, they will not understand why it may be producing divergent results or be able to figure out the best way to restore it to an earlier, more accurate version, said Veera Budhi, assistant vice president and global head of cloud, data and analytics at technology consultancy Saggezza.
Indeed, an effective AI transparency program consists of much more than integrating a few ethical checks and black box decoder algorithms, said Josh Poduska, chief data scientist at data science platform vendor Domino Data Lab. It requires thinking about the end-to-end data science pipeline. CIOs need to consider project management, data lineage, experiment tracking, reproducible research, validation and explainability routines, model monitoring and more.
For example, Poduska worked with one medical executive whose team had built a model for automatically triaging patients based on current symptoms. A skilled ER technician noticed that the triage system was not recommending that diabetics with advanced flu-like symptoms be admitted to the ER, despite the fact that a combination of diabetes and the flu can be quite serious and often requires hospitalization. It turned out that in the data used to train this new system, every diabetic with the flu was sent directly to a special clinic rather than the ER -- a decision that was not properly captured in the training data. This could have led to serious problems if the technician had not spotted the issue.
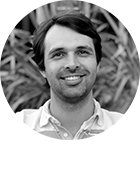
But explainable and transparent AI raises issues of its own, according to experts -- and finding a balance between AI accuracy and explainability is not easy. Saggezza's Budhi said that data scientists will have to weigh the tradeoff between the quality of the AI results and the requirement to explain how the AI reached those results.
Juan José López Murphy, technical director and data science practice lead at Globant, a digital transformation consultancy, pointed to another issue with explainable AI: Once something is explained, people are more likely to take the result as valid, even if the explanation is wrong. As a result, people may be less likely to challenge the AI when they should.
Building trusted data pipelines: Provenance, quality and governance
AI models are only as trustworthy as the data they are built on. However, with the growth in the volume, velocity, types and sources of data, it is becoming more difficult to establish the provenance and lineage of each piece of data available. It falls to technology executives to develop processes that can track data provenance and lineage to improve trust in AI and analytics models.
There are a variety of places where CIOs can run into challenges ensuring data provenance, said Minesh Pore, CEO and co-founder of BuyHive, a supply chain sourcing service. They include the following:
- Data collection overhead. There are a huge number of online streaming data sets that are used in big data analytics. These data sets increase the collection overhead.
- Large workflows. The user-defined functions (UDFs) in databases and other repositories can run into the millions, thus making recorded data much larger than original data. This data will need to be reduced in size without compromising quality by, for example, culling UDFs that are no longer necessary.
- Difficulty reproducing the execution. It's also important to capture data about the settings and versions of the execution environment in order to correlate changes in configuration settings with any data quality problems that arise.
- Integrating and storing distributed data provenance. Storage and integration of data provenance information is complicated. Enterprises normally save the provenance of UDFs running on big data systems. They need to stitch together the collected information and update it as new analysis takes place.
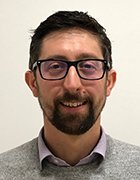
"Providing clear information on data's provenance to everyone who interacts with that data, or [uses] the insights that are generated from it, is fundamental to making successful decisions," said Peter Baudains, head of solutions and analytics innovation at The ai Corporation, a fraud detection tools provider.
It's important, because sometimes bad data can look like it is clean, complete and relevant. When a company cannot distinguish between good and bad data, it can encourage false confidence, which leads to bad outcomes.
Provenance issues impact data quality
Solid data provenance and data lineage practices lead to better decisions. But the task is not trivial. When data sets were small, it was practical to manually provide the required provenance and lineage information. But as data sets have grown and continue to grow, this places a huge burden on data experts. Baudains said that automated systems and tools for building and maintaining metadata are becoming more widely available. These tools need to be supplemented with workplace initiatives to promote transparency and data sharing across the organization through workshops or by encouraging different teams to collaborate on common problems.
With so many sources of data available and circulating through a single organization, it's essential to set up the right technologies, policies and processes that will help manage and track data like any other asset, agreed Anne Hardy, CISO at data integration vendor Talend.
"Losing track of where data's been, how it was collected and what it was used for can cause costly errors in business decisions and expose organizations to financial and regulatory risks," she said. Hardy recommends that CIOs begin to think about data as an asset and use best practices from asset management in IT or finance to create a data governance practice that incorporates provenance and lineage.
The ongoing challenge of data quality will only get worse if data provenance is not addressed, said Bharath Thota, partner in the data science and advanced analytics practice of Kearney, a global management consulting firm. He is seeing increased interest in open source tools like Kepler and CamFlow for managing data provenance. According to Gartner's Burke, interest in using blockchain and distributed ledger technologies for managing data provenance is also picking up, particularly on data that flows across enterprises. But it's important to recognize that distributed ledgers only keep track of the exchange of data and not its underlying quality.
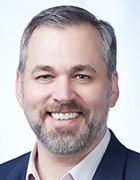
Down the road, CIOs might want to keep an eye on the development of trust fabrics to facilitate data confidence, said Jason Shepherd, vice president of ecosystem at Zededa, an edge computing tools provider. Trust fabrics, like the Data Confidence Fabric from the Linux Foundation's Project Alvarium, promise a framework for orchestrating trusted AI models with measurable confidence. The fabrics layer different capabilities across the data lifecycle, spanning devices, data exchange and computation. CIOs will need to think about how to build these fabrics in a way that balances the need for privacy, IP protection and regulatory compliance with data-sharing partnerships.
Interplay between governance, efficiency and trusted data
CIOs will have to work with executives across the organization to build out data governance practices that address the demands of new regulations like the EU's GDPR and California's CCPA to ensure that intentions match up to results. For example, GDPR has specific mandates stipulating that automated decision-making, which includes the use of AI models, requires enterprises to do the following:
- give individuals information about the processing of their personal data;
- introduce simple ways for them to request human intervention or challenge decisions; and
- carry out regular checks to make sure the systems are working as intended.
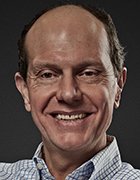
Ray Eitel-Porter, managing director and global lead for responsible AI at consulting firm Accenture, argued that new privacy-focused regulations are not only driving businesses to be more diligent about how they collect, store and use data, but also more efficient in how it is to be used. "As enterprise adoption of AI increases, a strong governance foundation will be part of what propels some organizations to success while others falter," he said.
Enterprises have long focused on automating a wider variety of data sources than they could process manually. Pros' Silver said that CIOs now need to turn their attention to streamlining aspects of data governance, such as security, data quality and ensuring the right data is used to train AI models.
"Governance processes need to work at scale so that data quality can be ensured for large volumes of varying data types from disparate sources," Silver said.
Taking computation private
Some of the most potentially useful data also happens to be the most regulated. For example, regulations like HIPAA place strict limits on the use of medical data that might help identify the spread of COVID-19 or identify the efficacy of a new medical intervention. In addition to the regulations limiting the use of such data, people are often reluctant to share fine-grained behavioral data out of concern for how it might be used.
A new approach referred to as confidential computing promises to address these limitations by enabling the development of more accurate algorithms while mitigating the risks of data leakage.
Confidential computing helps application developers tightly control what data the application processes. A healthcare AI developer, for example, can set a policy of taking data only from trusted hospitals and authenticating the applications accessing the data. This gives the developer the ability to control and verify the chain of custody of the data.
Another aspect of confidential computing lies in finding new ways to maintain the privacy of data collected from multiple sources, combine it in a secure and encrypted location, and do analysis or train algorithms without violating privacy laws.
"This opens up new AI/ML opportunities in finance, healthcare and retail industries that were not possible before," said Ambuj Kumar, co-founder and CEO of Fortanix, a cloud security platform.
Dr. Chirag Shah, associate professor in the online Master of Science information management program at the University of Washington, expects to see a category of confidential computing known as federated deep learning increasingly used to build AI systems that don't run afoul of data privacy regulations. Federated deep learning trains models on endpoint devices. The on-device machine learning uses local computation to build AI models of fine-grained behavior without sharing actual user data.
"There are limitations to this technique, and it doesn't work for all kinds of problems, but it's a good start," Shah said.
Automating encrypted computing
As Shah and others noted, confidential computing techniques are still very new and most of the conversations at this point are about the theoretical aspects of such platforms. But at some point that will change, experts said, and confidential computing techniques will become the industry standard for all data processing.
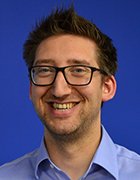
A big part of the field will revolve around encryption, said Oliver Tearle, head of innovation technology at The ai Corporation. The techniques range from simply processing unencrypted data on secured enclaves to a cutting-edge technique like homomorphic encryption -- the conversion of data into ciphertext that can be analyzed and worked with as if it were still in its original form, a process that requires special servers.
The downside is that encrypted computing does add some overhead, Tearle said, but he expects to see new hardware developed to perform the encryption efficiently, automatically and privately, leaving developers free to focus on data processing rather than confidentiality. This will mirror the development of other types of silicon for dedicated AI processing.
"The technology will become a standard hardware feature in all servers and will be an extremely important feature for cloud processing, where data is most vulnerable to attack," Tearle said.
CIOs will need to work with developers, security teams and testers to ensure that no data is readable in memory or on disk in unencrypted form at any time. CIOs may also want to ensure the data is encrypted for as much of the process as possible and develop encryption tools for any identified gaps.
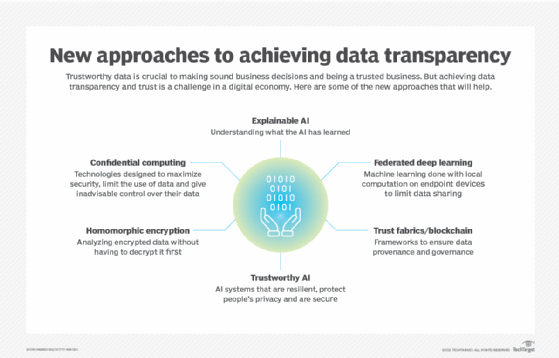
Building online trust
In parallel with the various privacy regulations, technology companies that build browsers and mobile phones are starting to create new barriers that make it harder to track consumer behavior online. This runs the gamut from restrictions on the use of tracking cookies to new controls and permissioning schemes regarding whether and how data can be collected from apps.
For example, in spring 2021, developers will have to adhere to Apple's App Tracking Transparency (ATT) policy, which requires third-party apps to ask Apple device users for their permission to track them. Apple, Google and Firefox are also working on privacy-focused updates to the most popular browsers. These include Safari's Intelligent Tracking Protection, Firefox's Enhanced Tracking Protection, and Google Chrome's announcement to phase out third-party cookies by January 2022.
The so-called "post-cookie" future has roiled the marketing and advertising industry, creating uncertainty about what happens next, said Tido Carriero, chief product development officer at customer data platform (CDP) vendor Twilio-Segment.
"One thing we know for certain is that first-party data -- data collected directly from a company's audience of customers, site visitors and social media followers -- is really the only future-proof option," he said.
David M. Raab, president of marketing technology consultancy Raab Associates Inc. and founder of the CDP Institute, believes big tech's anti-tracking efforts may shift advertising toward walled gardens that have rich first-party data stores, such as Google, Facebook and Amazon. He also sees larger companies like Walmart and Kohl's building their own smaller universes or "walled flowerpots," as he called them, which could reintroduce some competition.
The larger question for the advertising industry, he said, is what constitutes trust in an era when consumers increasingly care about data privacy. Raab argued that consumer trust in a brand is tied in large part to the brand's ability to deliver a seamless customer experience, which in the digital age requires a technical prowess. Good customer service comes down to operational capabilities like processing orders with one click, making returns very easy and having customer records available to call center agents.
"It's not about personalized advertising, even though marketers love to use that as a reason why customers should give them data," Raab noted.
The challenge brands face is that customers are reluctant to share data but expect services based on the data they didn't share. This lack of data makes some analytics harder, but Raab believes there's still plenty of data available -- especially about customer transactions, which drives the most powerful analytics and predictions of customer interests and behaviors.
In Raab's view, technologies that allow surreptitious tracking and skirt privacy rules will get shut down by regulators over time. What will survive are services that require sign-in by users, whether through logins needed to access a website, devices that require user registration (e.g., smart TVs) or apps that require users to identify themselves. There will also be services that cross-link the IDs of these systems based on common information, such as the email address. Privacy rules may constrain how such linking works, but presumably it will be allowed with consumer consent built into the terms of service.
"There's plenty to be done without violating privacy, and companies can get permission to collect plenty of data if they ask properly," Raab said. "A lot of that comes down to building trust with consumers that their data will be held securely and used in the customer's interest."
Data transparency and employee trust
CIOs and other executives also need to think about how to build trust in the adoption of new work-tracking technology. On the one hand, AI can help to spot patterns and opportunities for improvement that humans may miss. But the flip side is it can lead to fears that Big Brother is watching out, which may discourage employees from using the tools or encourage them to find creative ways to sabotage them.
"Businesses will need to carefully explain the intentions in terms of how it protects employees and customers, rather than simply addressing an organization's needs," said Andrew Pery, AI ethics evangelist at ABBYY, a digital intelligence tools provider.
For example, new task-tracking tools can use AI to find ways for employees to work more efficiently. Pery observed that companies often see better adoption when they make present these technologies in a way that inspires employees to improve their productivity, rather than as an added burden or a threat to their jobs.
Putting people first
At the core of this changing landscape is the relationship between individuals, the data about them, and how the data is used by businesses and governments in ways that can be easily understood and managed. That will require consumers -- all of us -- to come to terms with and manage our digital identities, said Gartner's Burke. Digital identity is defined as the information used to represent a person, role or organization. This could be as simple as using Google, Apple or Facebook to manage your passwords, shared data and relationships with multiple services.
In the future, a person's digital identity could be used to create agents that act on his or her behalf, a concept Gartner has dubbed the digital me. These agents could be deputized to do our shopping, manage our online relationships or identify how our data is being used.
Forrester predicted that renewed interest in privacy will lead to the consumer adoption of personal digital twins. Fatemeh Khatibloo, principal analyst at Forrester, described the concept as "an algorithm owned by an individual, optimized for his or her personal objectives. It filters content that is counterproductive to the individual's goals and identifies opportunities that support achieving them."
She predicted these opportunities will be driven by paid business relationships rather than the freemium model employed by many online services today. For example, Amazon might create a "PrimePlus" service to automatically shop for people. Banks might offer personal digital twins to help consumers reach financial goals. Password management apps might extend to helping consumers keep tabs on how their data is being used.
Meanwhile, web pioneer Tim Berners-Lee is hoping to build consensus around Solid, a new standard that makes it easy for consumers to share and revoke access to their data with fine-grained permissioning features. The core idea is to provide a decentralized alternative to Facebook, Apple and Google that allows individuals to stipulate what kind of data is shared with third parties.
He has also launched Inrupt, a commercial service to help businesses operationalize the technology. It is currently being tested by NatWest Bank, the BBC and the U.K. National Health Service. Berners-Lee is imagining a world that makes it easy for people to allow their current apps to talk to each other, "collaborating and conceiving ways to enrich and streamline your personal life and business objectives." This would give consumers some control over the ways AI uses data to make decisions on their behalves or that affect their interests.
It's still early, and only time will tell how willing consumers are to take a deep dive into tuning the privacy settings across the apps that use their data. CIOs will nonetheless need to prepare now for a future where data transparency is vital to enterprise success.
Measuring the value of trust
To what degree does trust affect the value organizations derive from data?
A new study from the Open Data Institute set out to answer that question. The study, conducted by London consultancy Frontier Economics, is premised on the view that organizations understand how data has economic value, but they do not always possess the data needed to realize that value. Doing so requires working with other organizations and individuals.
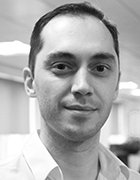
In particular, the study examined the impact of trust on data sharing, collection and use of data -- and the economic value that can be attributed to trust. "We wanted to get a sense of the multiplying factor of trust and how much it matters," said Olivier Thereaux, head of research and development at ODI.
"And yes, trust does matter."
But not that much. The study calibrated that, on average, a 1-point increase on a 5-point trust scale leads to a 0.27-point increase on a 5-point data-sharing scale.
Thereaux said the result would explain why consumers have been so willing to share data with social media companies, which sometimes have some of the lowest trust scores. "Even though the reported trust is low, we see convenience in it," he said, and so we are willing to share data.
The study's findings suggest that other factors besides trust -- including data infrastructure and data access mechanisms -- affect data sharing and should be studied.
For more on the research, how it was conducted and possible next steps, go to the "Economic impact of trust in data ecosystems."
--George Lawton