Rooting out racism in AI systems -- there's no time to lose
A confluence of events has made this the right time for enterprises to examine how racism gets embedded in AI systems. Is your leadership up to the challenge?
How will AI strategy in the enterprise be changed by the widespread attention to systemic racism?
Like a lot of complicated topics, the discussion of racism in AI systems tends to be filtered through events that make headline news -- the Microsoft chatbot that Twitter users turned into a racist, the Google algorithm that labeled images of Black people as gorillas, the photo-enhancing algorithm that changed a grainy headshot of former President Barack Obama into a white man's face. Less sensational but even more alarming are the exposés on race-biased algorithms that influence life-altering decisions on who should get loans and medical care or be arrested.
Stories like these call attention to serious problems with society's application of artificial intelligence, but to understand racism in AI -- and form a business strategy for dealing with it -- enterprise leaders must get beneath the surface of the news and beyond the algorithm.
"I think that racism and bias are rampant in AI and data science from inception," said Desmond Upton Patton, associate professor of sociology at Columbia University. "It starts with how we conceive a problem [for AI to solve]. The people involved in defining the problem approach it from a biased lens. It also reaches down into how we categorize the data, and how the AI tools are created. What is missing is racial inclusivity into who gets to develop AI tools."
A confluence of events has laid the groundwork for a meaningful examination of how AI systems are developed and applied. The killing of George Floyd and the Black Lives Matter movement have shined a light on how racial biases are woven into the fabric of the modern power structures that define communities, governments and businesses. In response to the growing public awareness of how racism gets institutionalized, corporations have started to make changes -- for example, stopping the sale of facial recognition technologies to governments and removing terms like slave and blacklist from IT systems.
Understanding how AI's many techniques are used by businesses and governments in ways that perpetuate racism will be a longer and harder effort.
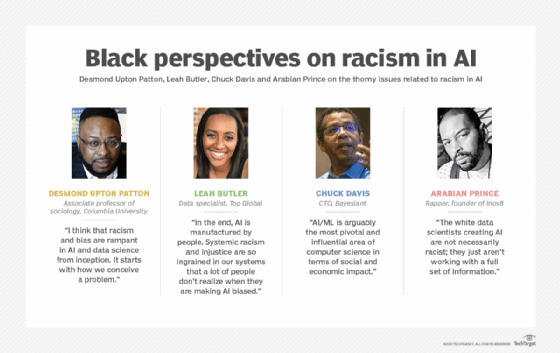
Speed and scale of AI bias
Bias in technology long predates the enterprise's current rush to adopt AI techniques. As Princeton Associate Professor of African American Studies Ruha Benjamin laid out in her 2019 book Race After Technology: Abolitionist Tools for the New Jim Code, racism was baked into camera film and processing, web cams, medical diagnostic equipment and even soap dispensers. But AI bias presents a singular problem, experts interviewed for this article said, because of the speed and scale with which AI can alter business processes.
As businesses increase their use of AI to streamline business processes, the fear is they may also be streamlining racial inequality. Chuck Davis, CTO of Bayesiant, an analytics software vendor that is using AI to quantify COVID-19 risks in specific areas and populations, argued that there's not a moment to lose in effecting changes to how AI is developed and applied.
"There is not a facet of life that will not be touched by AI and machine learning over the next five to 10 years," he said. "Practically every decision that can be made about access to capital to start or expand a business, buy a house, a car, and even the type of medical care you receive, will be based on AI to drive that assessment. If you don't have enough people of color working in this domain, you will perpetuate systemic bias."
Davis said he is hopeful that the coronavirus pandemic has moved people to be more empathetic to the ways in which certain populations are constrained due to forces outside their control, making this the right time to delve into how racism is baked into technology.
In addition, the lessons we learn from unwinding systemic racism in AI technologies, according to Benjamin, could give us insight into how other forms of institutionalized oppression get inadvertently baked into algorithms in the name of efficiency, progress or profit.
"The plight of Black people," she wrote in Race after Technology, "has consistently been a harbinger of wider processes -- bankers using financial technologies to prey on Black homeowners, law enforcement using surveillance technologies to control Black neighborhoods, or politicians using legislative techniques to disenfranchise Black voters -- which then get rolled out on an even wider scale."
Social context, not just statistics
It may be tempting to begin conversations about unwinding systemic racism in AI by looking at the raw numbers. But some evidence suggests that using a quantitative approach to mitigate racial bias can sometimes generate more systemic racism.
In their 2018 paper "The Numbers Don't Speak for Themselves: Racial Disparities and the Persistence of Inequality in the Criminal Justice System," sociologists Rebecca Hetey and Jennifer Eberhardt showed that presenting statistics on racial disparities in the criminal justice system paradoxically "can cause the public to become more, not less, supportive of the punitive criminal justice policies that produce those disparities." White voters in California, for example, were "significantly less likely" to support efforts to lessen the severity of California's harsh three-strikes law when presented with photographs depicting Black people as accounting for 45% of prison populations than when shown photos representing them as 25% of inmates, the authors reported.
Rather than focus on the shocking statistics when analyzing racial disparities, Hetey and Eberhardt argued it's more useful to look at the forces that shaped the statistics: e.g., to explore the social and technical processes that may have led to unequal treatment of people; to challenge associations by showing how implicit linking of Black people with a particular outcome, like crime, can affect perceptions, decision-making and actions; and to delve into the role institutions play in guiding policies and preferences that perpetuate racial inequities.
Leah Butler, data specialist at PR and marketing services firm Top Global LLC, said that as enterprises look to root out AI bias in their systems, they may benefit by bringing in sociologists like Hetey and Eberhardt.
"A lot of the research on this is being done by academics that know how to conduct social science research, and this needs to be brought to the forefront [as enterprises] build policies and add transparency [to AI systems]," said Butler, who recently earned a doctorate in criminology. "In the end, AI is manufactured by people. Systemic racism and injustice are so ingrained in our systems that a lot of people don't realize when they are making AI biased."
Role of the algorithm in AI bias a matter of hot debate among AI elite
A big debate among experts interested in rooting out systemic racism from AI is how much to focus on the algorithms versus the individuals, businesses and governments that shape the way the algorithms are built.
This issue came to a head recently in a widely publicized debate over the cause of bias discovered in an AI technique developed at Duke University to upgrade low-resolution images. The debate erupted after someone found that the algorithm, called PULSE, turned a photo of President Obama into an image of a white man. Two prominent AI experts -- Yann LeCun, chief AI scientist at Facebook and a pioneer of deep learning, and Timnit Gebru, technical co-lead of the ethical AI team at Google and co-founder of Black in AI, an advocacy group for promoting diversity in AI -- clashed over the case on Twitter, and fireworks ensued.
LeCun argued that the problem was a simple matter of data set bias that was easily corrected by adding more black faces to the training set. But Gebru argued that LeCun's analysis missed the underlying problem. "You can't just reduce harms caused by ML [machine learning] to dataset bias," tweeted Gebru, who has championed Benjamin's approach of examining the structural and social problems that feed AI bias.
Andrew Ng, founder of online training company Deeplearning.ai, noted in a blog post about the debate that even unbiased algorithms can lead to biased outcomes. For example, creating a better algorithm for helping lenders optimize interest rates for payday loans disproportionally hurts the Black community because of the nature and use of these high interest rate loans.
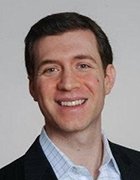
"Even if an algorithm itself is unbiased, that does not mean it couldn't be used in unethical ways," said David Snitkof, vice president of analytics at Ocrolus, which is developing AI for loan underwriting. For example, if a lender were to offer a financial product with misleading terms or deceptive pricing, that could still be unethical, regardless of the unbiased nature of underlying data. "What matters is how humans decide to apply it," he said.
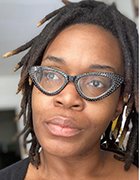
Sydette Harry, AI and algorithms policy lead at UserWay, a website accessibility compliance service, said the biggest issue in unraveling AI bias is that enterprises and institutions are barely thinking about systemic bias. "When it's thought of at all, it's being approached from the perspective of fault with the algorithms, instead of how people are actually affected by it," she said.
Facial recognition software: Facing the limits of AI
The problem of bias in facial recognition systems and the misuse of these systems by police have been at the forefront of public debates around systemic racism in AI. IBM, Microsoft and Amazon have all taken a stance against the use of their facial recognition software for mass surveillance. In June 2020, IBM CEO Arvind Krishna wrote a letter to Congress stating the company's desire to work with Congress on racial justice reform issues, including the responsible use of technology policies by police.
Critics, however, have argued that the retreat of megavendors like IBM, Microsoft and Amazon may actually hurt the cause by opening the field to smaller, less visible -- and allegedly less well-intentioned -- companies.
One issue is that the actual algorithms used for facial recognition are less accurate for Black faces than white ones. The result is that these algorithms can misidentify suspects. The American Civil Liberties Union (ACLU) found in 2018 that Amazon's Rekognition computer vision platform erroneously matched 28 of the 535 members of Congress with a database of criminal mugshots; 11 of the 28 mismatched Congressional leaders, or nearly 40%, were people of color, who then represented only 20% of Congress.
Amazon, an advocate for federal legislation of facial recognition technology, has disputed the ACLU's representation of its Rekognition software. In a blog post on machine learning accuracy, the company stated that the default 80% "confidence threshold" used by the ACLU, as opposed to the 99% confidence threshold recommended by Amazon for public safety use cases, is "far too low to ensure accurate identification of individuals; we would expect to see false positives at this level of confidence." In addition, Amazon said the cloud-based machine learning software is "constantly improving."
Though errors in facial recognition software aren't confined to misidentification of people of color, the fact remains that these kinds of surveillance tools tend to be employed more often against non-white people than other populations. This combination of questionable accuracy and higher usage is a recipe for disaster, as seen in the false arrest of a 42-year-old man near Detroit in January 2020.
Police departments are not the only organizations having to reassess their use of AI-powered surveillance software. Rite Aid recently announced it was shelving the use of facial recognition in its stores after it was called out by a Reuters story for primarily deploying the technology in lower-income and non-white areas of New York and Los Angeles. Facebook has agreed to pay $650 million in a class-action lawsuit over its use of automatic facial recognition in the wake of a new law in Illinois.
The new scrutiny has also encouraged researchers to take a deeper look at the data sets used to generate facial recognition algorithms. For example, MIT researchers discovered systemic racism baked into a data set of 80 million tiny images created in 2006. They issued a statement in June 2020 asking others to audit AI algorithms that may have used this data set since applications for analyzing photos may inadvertently use racist and sexist terms in image descriptions.
Arabian Prince, a rapper and founder of Inov8 Next, a product innovation incubator that aims to empower people in the inner city, said the global Black Lives Matter protests of recent months have ignited concern across the business world over the misuse of AI tools, including facial recognition software.
"With COVID and the movement, a lot of big companies are stepping up and trying to create the change," Prince told SearchCIO. "You are seeing companies speaking up that have never spoken up before."
AI is only as smart as the information fed into it. "The white data scientists creating AI are not necessarily racist; they just aren't working with a full set of information," Prince said. They are basing things on their experience, he said, voicing the common call for more diverse AI teams. "If you have people of color on your team, if you have all the races on your team, sharing information, then people don't get left out."
AI harnessed for COVID-19
A tragic effect of systemic racism has been the impact of COVID-19 on the Black community in terms of the higher percentage of cases and mortality rates. Bayesiant's Davis discovered these inequities after pivoting his company to focus on building AI and analytics tools to improve the detection of and response to the COVID-19 outbreak.
He had previously built tools that used AI to see who was likely to develop conditions like diabetes, cardiovascular disease and hypertension, mapping these at-risk populations geographically and demographically. Several healthcare organizations were interested in exploring the use of Bayesiant technology to improve treatment for these common afflictions when COVID-19 came along and the focus turned to using the technology to predict the impact of the virus on populations.
"Early on we were seeing there were huge inequities and disparities within communities of color," Davis said. He said he views the phenomenon as linked to systemic racism. For example, African Americans tend to live in larger households but smaller living spaces, which makes it more difficult to socially distance and quarantine at home, he said.
"It is magical thinking that we all have the same opportunities and are equal, and COVID-19 shows it's not true, with disproportional mortality rates," Davis said. "Anyone that looks at systems that use AI and ML needs to look at inclusion and equity."
His team has used AI to develop a COVID-19 risk score, which is a prediction of someone's likelihood to development symptomatic COVID-19 that will require hospitalization. "If you know that, you can do certain things like prioritize vaccinations among those people."
The flip side of this is that vaccination trials are finding it difficult to recruit enough Black volunteers. "You will be hard-pressed to find a Black person that wants to be part of these trials. Older generations, who are more susceptible to the virus, remember things like the Tuskegee experiments," Davis said, referring to the unethical U.S. Public Health Service Syphilis Study at Tuskegee conducted between 1932 and 1972. "They are especially suspicious of any kind of dangerous study, [believing that] if it is dangerous, they will give it to us because our lives are less valuable."
Natural language processing tools that don't recognize Black voices
Another growing concern is that Black voices are literally being left out of the algorithms used to train AI. The lack of Black voices in training data could not only result in AI being unable to understand Black speakers, but also put Black people in harm's way if speech patterns are misinterpreted. For example, early AI tools identified the usage of the n-word as hateful language, but among Black people, the term has more nuance and is sometimes used in an innocent exchange.
AI untrained on Black voices could also make it harder to prioritize interventions for at-risk youth, a population of interest to Columbia University's Patton. To address the software gap in speech recognition patterns, Patton's research team focused on how to train AI using social media posts, after discovering that social media communications by gang-involved youth were often misinterpreted in ways that had legal and mental health consequences. Patton asked his students to think about how AI could be used to interpret and annotate social media posts of this population as they did their research, and then think about the ethics of using these tools in various communities.
His team, working with youth groups in the high-crime Brownsville neighborhood in Brooklyn and with the Teaching Systems Lab at MIT, developed a natural language processing tool to capture issues of racism and bias in the interpretation of the social media language used by low-income youth of color, with the goal of correcting misinterpretations that could penalize them.
"We realized this was an opportunity for not only creating better tools, but could also be a new pipeline for [getting] Black people involved in the creation of AI," Patton said.
The trust in technology divide
Trust in AI also breaks down along racial lines, according to Fabian Rogers, community advocate for the Atlantic Plaza Towers Tenants Association in Brooklyn. The Black communities he represents are more likely to mistrust the intentions of the organizations rolling out new technologies and services in general, he said.
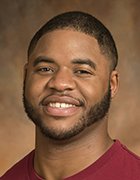
Rogers, who is also a distribution coordinator for on-demand content distributor InDemand LLC, cited a campaign he helped organize against the use of facial recognition technology in his apartment complex. In recent years, the Atlantic Plaza Towers complex has attracted new residents who typically pay almost twice the rent of existing residents, he said.
Facial recognition technology is generally seen as a selling point by the upwardly mobile populations coming into New York, since it provides an extra layer of security on top of key fobs for building access. But the existing residents of the complex did not see it that way, according to Rogers. "There is a reactive relationship with technology -- we were reacting to the fact there is something coming to the neighborhood we never asked for," he said. The communities he represents are more sensitive to the adverse ways in which technology can affect them, including the use of facial recognition software, which has a track record of misidentifying black faces.
"Black people need privacy in a different way than others; the various microaggressions and micro-prejudices eat at you," he said. "It eats at your mental state and makes you question, 'Why me?' And there is no logical reason for it except that you are Black."
Using facial recognition technology at the apartment complex seemed like a recipe for disaster to Rogers. "In the U.S., the approach is to break things first and ask questions later," he said, explaining that he became a "reluctant advocate" for the apartment complex, because there were no government policies in place to protect existing tenants.
Enterprise tech and the dearth of Black professionals
As enterprises devise a strategy for rooting out racial biases in their systems and tools, they must confront a troubling reality in their ranks: the dearth of Black executives, engineers and sociologists in tech disciplines, AI in particular. Bayesiant's Davis observed that he could count on one hand the number of Black people at Disrupt SF 2019, the last TechCrunch conference.
"AI/ML is arguably the most pivotal and influential area of computer science in terms of social and economic impact, so of course I'm concerned when it looks more like an exclusive club than a representative science," he said.
In the development process in particular, he said, there are not enough eyes that are sensitive to Black concerns. Quite often, he sees data scientists pulling in data out of convenience rather than aiming for a representative sampling of populations affected by an algorithm. This is a major concern in systems for credit scoring or early parole recommendations.
Davis was involved in the audit of an AI system introduced by a private firm and designed to reduce the backlog of cases for granting early parole in a county. Even though the data scientists involved removed all obvious bias from the data, such as race and ZIP code, it still returned biased results. The whole reason for introducing the system was that the county had felt that people of color were incarcerated for too long. But in practice, the new system would have amplified the effect, Davis said.
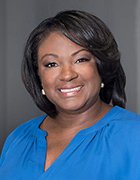
"Not having people with diverse backgrounds and experiences to build and implement technology opens the door to systemic racism being perpetuated by computers in even more dangerous ways than human decision-making because it can be harder to trace and delete," said criminal justice advocate Teresa Hodge, CEO and co-founder of SaaS startup R3 Score and fellow at Harvard Kennedy School.
Hodge began looking at how technology helps and hurts people who have been imprisoned after serving a 70-month federal prison sentence. Her company's namesake technology is a risk-underwriting tool that assesses the strengths and capabilities of people with criminal records with the aim of improving their employment prospects and ability to achieve financial stability.
Using AI to detect bias
So how do enterprises and educational institutions detect and correct the racial biases inherent in AI algorithms for all the reasons discussed above? Researchers are developing a variety of techniques to help detect and quantify bias -- and are formulating KPIs to measure the impact of various interventions to correct biases:
- The city of Oakland worked with Stanford researchers to develop AI that could analyze the respectfulness police officers demonstrated when speaking with white and Black citizens during routine traffic stops. They found that officers' language was less respectful when directed at Black people than at white people.
- Vendors like UserWay are developing commercial services for detecting bias in the language used on websites. These kinds of tools can be a first step for enterprises to explore how systemic racism is already showing up on their digital doorsteps. "We need tools that have the ability to truly examine legacy content at scale," UserWay's Harry said. The company has been working with the W3C, which is responsible for web standards, to develop new HTML code to warn users about potentially disturbing language or content that may cause distress. An automated scan of over 500,000 business websites done by UserWay's rules engine revealed that 22% have some form of biased language, with more than half of that coming from racial bias and about a quarter from gender bias. Some of these characterizations were a bit nuanced and included words like whitelist, blackmail, black sheep, blacklist and black mark, as well as other, more offensive terms. Those most often flagged for gender bias included chairman, fireman, mankind, forefather and man-made. Racial slurs appeared on about 5% of the sites.
- Many enterprises -- including JP Morgan, Twitter and GitHub -- are also starting to audit their various systems to remove troublesome language from IT systems, such as the practice of using the terms master and slave to describe hard drives or coding branches.
Getting to inclusive AI: New standards
While these measures are certainly a start, more substantive changes are needed, said Bayesiant's Davis.
"I think, to a certain extent, we are getting sensitive on things that are not important when we should be focusing on things that make a lasting change," he said. "Instead of changing the names of things, enterprises should be focused on what they are doing to change people lives."
He offered three substantive changes that can be made right now:
1) track the provenance of data;
2) quantify the utility of models; and
3) implement algorithm review programs.
Some specific tools that organizations could adopt include the following:
Data sheets. Experts like Google's Gebru have called for the creation of data sheets for data sets. These would be akin to the data sheets used for characterizing electronic components. These sheets would describe things like recommended usage, pitfalls and any guidance on the level of bias in the data set.
The data sheets would help organizations make better decisions about how they use data, said Top Global's Butler. In the absence of policy guidance, she said databases that are supposed to help society end up contributing to a problem. She pointed to the publicly available database Uniform Crime Report, which is used to analyze crime rates.
"Criminologists know that the UCR is flawed in its collection strategy and causes underreporting for certain crimes and certain demographics," Butler said. But they use it anyway because that is what's available: "In the end, it takes a culture shift, policy shift and everyone coming together for the common goal of equality among people."
Model cards for machine learning models. Google recently introduced the Model Card Toolkit, which provides a structured framework for reporting on machine learning "model provenance, usage, and ethics-informed evaluation."
Previous versions of model cards required a lot of manual work to gather the information needed for a detailed evaluation and analysis of both data and model performance. Google said its new toolkit helps developers compile information for models and provides "interfaces that will be useful for different audiences."
Algorithm review boards for proactive audits
Still, regardless of how much care is put into developing the model or how well the algorithm performs in the lab, there are countless cases of bias being found long after an algorithm is deployed.
For example, a team of researchers at UC Berkeley last year stumbled upon racial bias in an algorithm widely used by doctors to recommend treatments. They found that Black patients were not being given adequate treatment for their conditions based on a flaw in the algorithm that equated health costs with health needs. Less money is spent on Black patients than white patients for the same level of need, leading the algorithm to falsely conclude that Black patients were healthier than equally sick white patients and reducing the number of Black patients identified for extra care by more than half. Correcting the algorithm would increase the number of Black patients that received additional care from 17.7% of the total to 46.5%, the Berkeley researchers determined, and they worked with the developer to mitigate this bias.
The tragedy is that this discovery required a heroic effort by a third party long after the algorithm was deployed. In the long run, enterprises will need to include proactive auditing programs similar to what companies already do for software quality, with ongoing reviews to detect how well algorithms perform after they are released into the wild.
Bayesiant's Davis said he realized he had to pivot after discovering that some of the company's algorithms for quantifying insurance risk might lead to redlining. "I had to reconcile how my system could conceivably have done more harm than good," he said.
He argued that unwinding systemic racism in AI will require the creation of a process like institutional review boards that are used in medicine. "If I put out a clinical trial of a drug, I have to go through an institutional review board, but there is none of that for AI and machine learning," Davis said. Although industry leaders have talked about proposed standards, at the moment, it's just talk, he said. And none of the plans include measures for submitting data for review or ensuring inclusion.
Operationalizing diversity
Many companies create a values-based statement that includes the idea that their products will do no harm. But, as R3 Score's Hodge pointed out, coming up with a values statement is the easy part.
"While it is useful for large companies to go on what we call 'learning journeys,' the real work begins after the statements and in-house awareness has been raised, and this is where there is often less clarity about next steps," Hodge said.
Operationalizing diversity and inclusion doesn't have to be hard, she said. She recommended finding very specific data points that codify things like improving hiring outcomes, increasing the length of tenure among minority employees and shifting corporate attitudes about race. While this is not especially complicated, it can be uncomfortable and may feel like a distraction from just doing your job, she said. But progress won't be made without this kind of effort.
"Systemic racism is systemic for a reason; it is our status quo," Hodge said. Identifying and rooting it out will often compete with business as usual.
It may mean, for example, not delivering technologies and products because no one can figure out why people of color are not having the same outcomes. It could mean shelving money invested in R&D, staff time and marketing. It will mean having the courage to not deploy and not release until the playing field is level along race and income indicators.
"That is hard for a company but, in my opinion, that is what it will take to finally address racism in technology," Hodge said.
The work environment is no different from the whole of society, in which people are finally waking up to a conversation that is hundreds of years in the making in America, Hodge said.
"It is going to be messy, uncomfortable and, at times, painful. Many people are going to get it wrong, and some people are just not interested in being antiracist. These people get up and go to work somewhere every day, and some of them work in tech and build algorithms," she said. Progress will require leadership at the highest levels and sustained effort throughout the enterprise.
"Companies must navigate all of this while still operating. Real change, even while it is being discussed at the high level, will happen in small, everyday decisions," she said.