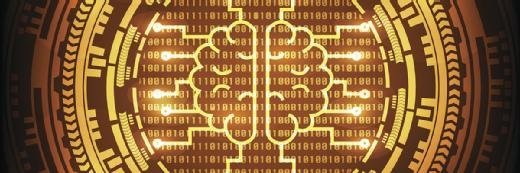
Getty Images/iStockphoto
6 ways AI and automation could improve process mining
Creating a digital twin of your enterprise, identifying automation candidates for RPA and connecting isolated tasks into processes are a few ways CIOs can advance process discovery.
Digital innovation requires enterprises to learn how to understand, manage and change increasingly complicated processes. A new generation of process mining tools promises to make it easier to automatically interpret the digital exhaust of modern enterprises to help improve decision-making, drive innovation, and offer new products and services.
"By understanding how processes really operate, companies can create operational fluidity to drive more efficient and productive operations that create better customer experiences," said Alexander Rinke, CEO and co-founder of Celonis, a process mining platform based in Germany. "Instead of simply identifying areas of friction, AI will further evolve process mining by allowing businesses to implement recommended changes with employees, enhancing productivity while also saving resources."
The core idea of process mining lies in finding new ways to create and calibrate models of how things work with event logs. The formal work started by looking at classic business applications, like ERP, to make sense of business processes, such as quote-to-cash. Now, though, process mining techniques are being in a variety of ways across business, technical and human processes. This innovation is fueled, in part, by better AI and machine learning algorithms. But it's more significantly fueled by the discovery of new tools like digital twins that make it easier to visualize complex processes.
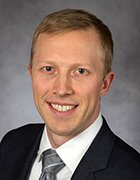
Modern process mining originated from the field of business process modeling notation (BPMN), which was the earliest of technology-focused attempts to identify processes -- and particular steps within those processes -- that held opportunity for improvement, said John Cottongim, co-founder and COO of Roots Automation, a New York-based automation-as-a-service company focused on delivering intelligent automation at scale. Cottongim recently lead automation efforts at Mars, the candy giant.
"The fact that we now see several players from this space entering the intelligent automation market should be no surprise, as there is clear value in the creation of digital twins that provide the ability to test and validate how system changes will impact a large ecosystem," Cottongim said.
The logical next step would be to automate those opportunities where available. Here are six steps where AI and automation can improve process mining.
1. Connecting discrete tasks into processes
Process mining tools are getting better at analyzing log data collected from applications and desktop clients to piece together complex processes that span multiple applications, or even across enterprises. This can help bridge the gap between an individual user's view of a task and more complex workflows and processes. Process mining has traditionally relied upon log data collected from the applications.
Now, vendors are creating bots that run on a desktop and use machine vision to record different views of a process to understand a process with higher granularity, such as FortressIQ's virtual process analyst.
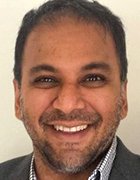
"It's easy to think of tasks as the individual actions, which can often be generic, that make up an overall process," said Pankaj Chowdhry, founder and CEO of FortressIQ, based in San Francisco.
A simple example would be sending an email. That's a task that, in context -- such as responding to a complaint or updating a salesperson with a new shipping date -- would be part of a process. Processes are composed of tasks and decisions and are usually across multiple systems and user groups. Workflows are typically the technical view of a process, which are implemented in BPMN systems.
"We've seen aha moments from clients when they see how a process spans multiple systems and sometimes even extends outside their enterprise," Chowdhry said.
Access to accurate, up-to-date process information changes the way a business is run. This is evolving from simple log analysis to "Fitbit for the enterprise," allowing the same simplicity in software activity tracking and analysis.
2. Understanding why processes are happening
The next step lies in trying to understand why a process is taking place to make sense of how individual components could be composed more efficiently or reimagined.
"Process cognition involves not only the 'What is happening?' but the 'Why is it happening?' across all applications and personas, including nonlinear processes," Chowdhry said.
These cause-and-effect relationships can be deciphered using new combinations of natural language processing, computer vision, sequence modeling, anomaly detection and machine learning.
In the future, these kinds of capabilities could be integrated with causal deep learning techniques to understand why changes to a process might cause things, rather than just be correlated with them.
3. Make process recommendations
Machine learning capabilities are being embedded into process mining tools to interpret existing processes and make recommendations. For example, the Celonis Action Engine analyzes data autonomously and continuously across modules, systems and processes. An AI module communicates valuable and personalized recommendations for action to human and digital workforces and can execute preapproved actions directly in an organization's source systems.
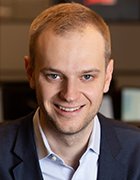
"It's an important start to identify process bottlenecks, but accelerated enterprise performance happens when you can use that information to recommend the best course of action to remove friction to create a more fluid process flow," Rinke said.
Tools for making personalized recommendations for guiding action and driving change to business processes will help further increase the value of process mining analytics. For example, machine learning could help identify patterns and anomalies to improve specific outcomes, such as delivery time.
4. Identify automation candidates
The process of creating robotic process automation (RPA) bots is much faster than more technical approaches, like API integration. However, there is still an overhead in identifying candidates for automation and recording the bot logic. Now, RPA vendors are looking to bridge this gap by deploying bots with machine vision algorithms to automatically identify existing process logic using tools like Kryon's Process Discovery.
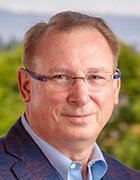
"On average, our customers are telling us that 60% of the time and often upward of 70% of the cost is accumulated while finding the right processes to automate," said Richard French, chief revenue officer at Kryon, based in Tel Aviv, Israel.
Process discovery can find these processes within just a few days. Automated discovery can also capture variations from employees, which can reduce the need for reworking the RPA logic. In addition, data about the process and variants can be used to generate bot scripts, as well.
5. Create a digital twin of an organization
A digital twin typically provides a model of something in the physical world that is calibrated and updated by new data. These have traditionally focused on physical products, like power plants and cars. Process mining techniques could also help create what Gartner is calling a digital twin of the organization (DTO), which focuses more on business processes, rather than physical products, to help enterprises improve performance, customer experience and business process outsourcing initiatives.
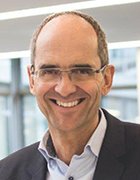
"In the future, DTOs will enable us to optimize all processes in Siemens and to measure the improvement in a more efficient way," said Lars Reinkemeyer, global process mining lead at Siemens and guest lecturer at the University of California, Santa Barbara. "DTOs are expected to be a significant contributor on the journey toward digital transformation."
Reinkemeyer has been working with other enterprises to bring DTO techniques into procurement, production, logistics and finance.
Siemens has been using DTOs to visualize millions of process steps and, thus, represent interactions between people and IT to identify opportunities for automation. For example, Reinkemeyer's team created a model of their order-to-cash process and developed metrics for measuring and improving process digitalization. In 2018, this approach reduced manual rework by 11% and increased the automation rate of the order-to-cash processes by 25%.
A DTO model captures and represents processes involving over 30 million different line items ordered each year as they progress through as many as 60 different process steps. Process mining is used to represent this data as a process graphic in real time as a digital twin of the actual processes.
Managers can look at the process from a global view, as well as an individual view that can be visualized. This enables comparisons between individual organizational units and makes changes to the actual process easier. One aim, for instance, may be to eliminate all but a few manual steps in a process.
6. Identifying deviations
One of the biggest challenges for process mining tools today lies in identifying deviations from a standard process. According to Cottongim, this is best done by human analysts. He said he believes current tool sets in the process mining space are primarily focused on identifying either the "happy path" of the process or identifying when individuals deviate from a prescribed, or standard, path.
While some technologies are making inroads to wrangle the complex web of alternate paths, there is still little light shed on whether these paths are casual deviations caused by user preference, actual deviations due to required exceptions or a workaround due to underlying system functionality.
"It is my opinion that we are at least several years away from these tools being able to both identify and explain process paths outside of the rudimentary straight-through happy path," Cottongim said.
The major challenge in this space is identifying off-screen business logic that is the root cause leading to the need for exception paths in the first place.
For the time being, practical process analysis continues to be the domain of valued business analysts with a firm grasp of the particular business context.
"While end-to-end process decomposition and, eventually, automated-automating seem possible in the future, given the current state of these technologies, process awareness and compliance seem much more at hand and will likely be where the value is derived in the short-term," Cottongim said.