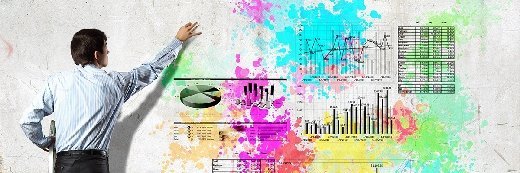
Sergey Nivens - Fotolia
5 strategies to address the GDPR data management conundrum
As IT executives continue to wrap their heads around GDPR, strategies are emerging to ease its data management compliance burden. Here are five that are already proving effective.
The EU's General Data Privacy Regulation creates a host of compliance challenges for IT executives. While most enterprises have at least basic compliance framework in place, there are a host of GDPR data management challenges that are important to address. Here are five ways executives are streamlining these GDPR strategies and processes to ensure data management remains compliant.
1. Automate data removal
One of the GDPR data management challenges IT executives face lies in reducing the burden of personally identifiable information (PII) data removal from their various systems. Under GDPR, strategies are required to ensure data will be deleted correctly, within appropriate time periods and with mechanisms to prevent the PII from being readded. In the early stages, it may be feasible to manually remove records, but this places a new burden on employees who may miss some data.
One practice is to use a central system such as a compliant CRM to track data and, if possible, automate appropriate GDPR data management processes and communications with people in the system, said Sultan Saidov, CPO and co-founder of Beamery, a recruiting and marketing automation service.
If someone calls the business and asks for, but does not have, a valid email on record, the company needs a process to determine if the person's consent has been given, delete the requested data and notify the sender. Decisions on whether to contact, keep data or delete it require strict data governance and/or automation. There also needs to be a process in place to prevent people who have asked to be forgotten from being readded to the system as well.
2. Use the business process approach
Traditional data inventories are long lists with details about personal data elements or processing activities, along with other details on the volume of data. These lists are just snapshots in time, however. Business processes are constantly evolving, but these lists may not get updated as frequently as business processes -- and GDPR strategies -- change.
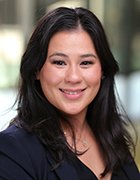
"The data manager does not have a full view on how the same data can be used in different ways by different systems and lacks details on how the data is being used, which is needed to ascertain risk," said Nicole Sroka, senior product marketing manager at TrustArc Inc., a compliance and security analytics provider.
For example, a traditional inventory would record the fact that an ERP system is used, but it would not consider the different risk levels of the data stored used for an HR process that includes social security numbers versus a marketing process that uses email addresses.
"One best practice is to use a business process-based approach instead of a traditional IT systems-based approach," Sroka said. This involves recording how and why data is used so that high-risk processing gets flagged for additional analysis such as a data protection impact assessment.
3. Create an app interface for data models
Enterprises are starting to use deep learning data for activities like probability of default modeling, ad serving and hundreds of other consumer applications. Executives may want to consider finding ways to streamline the way different people involved in GDPR strategies, communications and compliance management activities interface with the data behind these deep learning models.
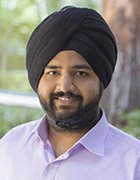
"Inferring what happens in those models is basically impossible for a human to comprehend, and revealing what algorithms and what weights were applied does not meet the standard of the law," said Gurjeet Singh, CEO and co-founder of Ayasdi Inc., an AI platform service.
"There are few best practices here as this is a requirement and technology," he explained. "There are, however, emerging approaches that can shine a spotlight into this otherwise black box."
One emerging practice is to use techniques like topological data analysis (TDA), an approach that discerns the behavior and learning patterns of neural networks. This also makes it easier to understand how a model changes over time. Techniques like TDA also make it easier to debug learning models to better understand the impact of deleted data.
These techniques are available to managers today -- the key is making them simple and accessible, despite the complexity that underlies them. Further, they need to be accessible by consumers. This requires an application interface.
"Thinking about these problems from an application perspective forces the enterprise to consider how they can fulfil these requests from the consumer's viewpoint," Singh said.
4. Create a data transparency team
Enterprises need a way to respond to a variety of requests under GDPR, including those to delete someone's information and explain how their data is used. The challenges to deliver on these rights and requests include confirming the identity of the requestor, tracking down all their data in multiple, disparate databases and providing a secure way to deliver it.
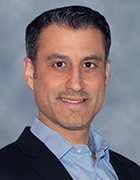
Enterprises must also be able to delete an individual's data but still maintain a method to show that the data was held, said John Brangaccio, director of digital marketing at ACI Worldwide Inc., the banking and payment giant.
This can be difficult when complex payment technologies are linked to third-party systems. Instead of just being required to identify where the data lives, enterprise leaders must figure out if it's the company's or a third party's obligation to erase the data under GDPR.
Addressing these challenges takes commitment and collaboration across the organization. It is beneficial to have methods such as automated ticketing systems to track and manage the requests, as well as to maintain visibility and provide access to all the different databases where the data is stored.
"In many cases, a full-time dedicated team may be needed, instead of adding these additional complex tasks to a current team's workload," Brangaccio said.
5. Model privacy threats
The GDPR data management rules expand the definition of PII to include things like IP addresses, location data and images of a person. Enterprises need to look at their systems to ensure they are not inadvertently collecting data they don't need but that might constitute a privacy threat. They may also want to model different threat models that may result in loss of PII.
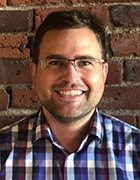
User-generated content continues to be a gray . Imagine a photo-sharing site where an individual uploads their own photos and content. The sharing of the image is performing the exact function of the site that the user agreed to. But, if geolocation data is not stripped from the image, an individual's location could be disclosed inadvertently, something the user generating the content likely did not consent to, said Travis Ruff, CISO at Amperity Inc., a customer data platform.
Executives may consider brainstorming possible privacy threat modelling sessions that could explore the ways in which the content in the enterprise's possession could be used to identify users and customers.
"Build in processes upstream and downstream to prevent unnecessary data from entering your services, and detect when it is trying to leave," Ruff said.