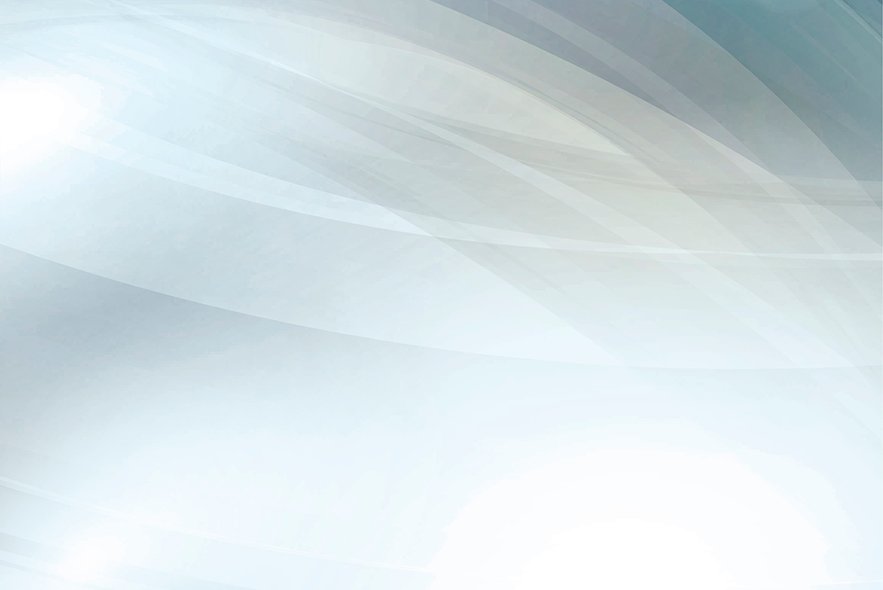
What is transfer learning?
Transfer learning is a machine learning (ML) technique where an already developed ML model is reused in another task. Transfer learning is a popular approach in deep learning, as it enables the training of deep neural networks with less data.
Typically, training a model takes a large amount of compute resources, data and time. Using a pretrained model as a starting point helps cut down on all three, as developers don't have to start from scratch, training a large model on what would be an even bigger data set.
ML algorithms are typically designed to address isolated tasks. Through transfer learning, methods are developed to transfer knowledge from one or more of these source tasks to improve learning in a related target task. Developers can choose to reuse in-house ML models, or they can download them from other developers who have published them on online repositories or hubs. Knowledge from an already trained ML model must be similar to the new task to be transferable. For example, the knowledge gained from recognizing an image of a dog in a supervised ML system could be transferred to a new system to recognize images of cats. The new system filters out images it already recognizes as a dog.
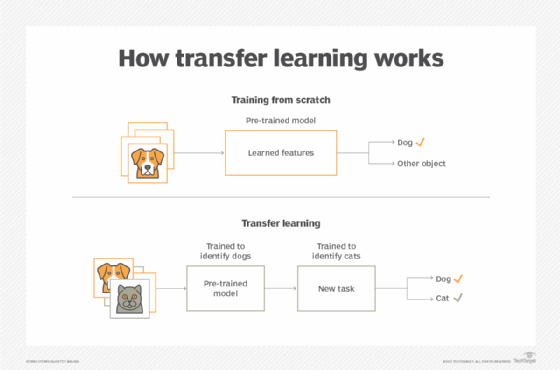
How to use transfer learning
Transfer learning can be accomplished in several ways. One way is to find a related learned task -- labeled as Task B -- that has plenty of transferable labeled data. The new model is then trained on Task B. After this training, the model has a starting point for solving its initial task, Task A.
This article is part of
What is machine learning? Guide, definition and examples
Another way to accomplish transfer learning is to use a pretrained model. This process is easier, as it involves the use of an already trained model. The pretrained model should have been trained using a large data set to solve a similar task as task A. Models can be imported from other developers who have published them online.
A third approach, called feature extraction or representation learning, uses deep learning to identify the most important features for Task A, which then serves as a representation of the task. Features are normally created manually, but deep learning automatically extracts features. Data scientists must then choose which features to include in the model. The learned representation can be used for other tasks as well.
Transfer learning theory
During transfer learning, knowledge is used from a source task to improve learning in a new task. If the transfer method decreases the performance of the new task, it's called a negative transfer. A major challenge when developing transfer methods is ensuring positive transfer between related tasks while avoiding negative transfer between less related tasks.
When applying knowledge from one task to another, the original task's characteristics are usually mapped onto those of the other task to specify correspondence. A human typically provides this mapping, but there are evolving methods that perform the mapping automatically.
The following three common indicators can be used to measure the effectiveness of transfer learning techniques:
- The first indicator measures if performing the target task is achievable using only the transferred knowledge.
- The second indicator measures the amount of time it takes to learn the target task using knowledge gained from transferred learning versus how long it would take to learn without it.
- The third indicator determines if the final performance of the task learned via transfer learning is comparable to the completion of the original task without the transfer of knowledge.
Types of transfer learning
Transfer learning methods fall into one of the following three categories:
- Transductive transfer. Target tasks are the same but use different data sets.
- Inductive transfer. Source and target tasks are different, regardless of the data set. Source and target data are typically labeled.
- Unsupervised transfer. Source and target tasks are different, but the process uses unlabeled source and target data. Unsupervised learning is useful in settings where manually labeling data is impractical.
Transfer learning can also be classified into near and far transfers. Near transfers are when the source and target tasks are closely related, while far transfers are when source and target tasks are vaguely related. If the tasks are closely related, this means they share similar data structures, features or domains.
Another way to classify transfer learning is based on how well the knowledge from a pretrained model facilitates performance on a new task. These are classified as positive, negative and neutral transfers:
- Positive transfers occur when the knowledge gained from the source task actively improves the performance on the target task.
- Negative transfers see a decrease in the performance of the new task.
- Neutral transfers occur when the knowledge gained from the source tasks has little to no impact on the performance of the target task.
Benefits of transfer learning
Transfer learning offers numerous benefits in creating an effective ML model. These advantages include the following:
- Reduces data needs. By using pretrained models that were already trained on their own large data sets, transfer learning enables developers to create new models even when they don't have access to massive amounts of labeled data.
- Speeds up the training process. Transfer learning speeds up the training process of a new model, as it starts with pre-learned features, leading to less time required to learn a new task.
- Provides performance improvements. In cases where the target task is closely related to the source task, performance can improve due to the knowledge it gains from training on the first task.
- Prevents overfitting. Overfitting occurs when a model fits too closely to its training data, making the model unable to make accurate generalizations. By starting with a well-trained model, transfer learning helps prevent overfitting, especially when target data sets are small.
- Reduces computational cost. Transfer learning reduces the costs of building models by enabling them to reuse previously trained parameters. This process is more efficient than training a model from scratch.
- Provides versatility. Retrained models consist of knowledge gained from one or more previous data sets. This can potentially lead to better performance on different tasks. Transfer learning can also be applied to different ML tasks, such as image recognition and natural language processing (NLP).
Key use cases for transfer learning
Transfer learning is typically used for the following key use cases:
- Deep learning. Transfer learning is commonly used for deep learning neural networks to help solve problems with limited data. Deep learning models typically require large amounts of training data, which can be difficult and expensive to acquire.
- Image recognition. Transfer learning can improve the performance of models trained on limited labeled data, which is useful in situations with limited data, such as medical imaging.
- NLP. Using transfer learning to train NLP models can improve performance by transferring knowledge across tasks related to machine translation, sentiment analysis and text classification.
- Computer vision. Pretrained models are useful for training computer vision tasks like image segmentation, facial recognition and object detection, if the source and target tasks are related.
- Speech recognition. Models previously trained on large speech data sets are useful for creating more versatile models. For example, a pretrained model could be adapted to recognize specific languages, accents or dialects.
- Object detection. Pretrained models that were trained to identify specific objects in images or videos could hasten the training of a new model. For example, a pretrained model used to detect mammals could be added to a data set used to identify different types of animals.
Transfer learning examples
In machine learning, knowledge or data gained while solving one problem is stored, labeled and then applied to a different but related problem. For example, the knowledge gained by an ML algorithm to recognize cars could later be transferred for use in a separate ML model being developed to recognize other types of vehicles.
Transfer learning is also useful during the deployment of upgraded technology, such as a chatbot. If the new domain is similar enough to previous deployments, transfer learning can assess which knowledge should be transplanted. Using transfer learning, developers can decide what knowledge and data is reusable from the previous deployments and transfer that information for use when developing the upgraded version.
In NLP, for example, a data set from an old model that understands the vocabulary used in one area can be used to train a new model whose goal is to understand dialects in multiple areas. An organization could then apply this for sentiment analysis.
A neural network might be used to search through medical images with the goal of recognizing potential illnesses or ailments. In this case, transfer learning could be used to help identify these ailments using pretrained models in cases where there's insufficient data to train the network on.
Future of transfer learning
The future of transfer learning includes the following trends, which might further shape ML and the development of ML models:
- The increased use of multimodal transfer learning. Models are designed to learn from multiple types of data simultaneously. These can include text, image and audio data sets, for example, which leads to more versatile ML and artificial intelligence (AI) systems.
- Federated transfer learning. This combines transfer and federated learning. Federated transfer learning enables models to transfer knowledge between decentralized data sources but does so in a way that keeps local data private. This enables multiple organizations to collaborate to improve their models across decentralized data sources while also maintaining data privacy.
- Lifelong transfer learning. This creates a model that can continuously learn and adapt to new tasks and data over time.
- Zero-shot and few-shot transfer learning. Both methods are designed to enable ML models to perform well with minimal or no training data. Zero-shot revolves around the concept of predicting labels for unseen data classes, and few-shot learning involves learning from only a small amount of data per class. Using this practice, models can rapidly learn to make effective generalizations with little data. This practice has the potential to reduce the reliance organizations have on collecting large data sets for training.
In addition, if the initial pretrained models were trained using data that was fairly obtained, then transfer learning might also be able to solve one of the prominent issues facing AI and ML -- where large amounts of data are collected without the data originator's permission.
Transfer learning will likely see broader adoption in the future, as it lessens the dependency on large collections of training data. It also makes ML and AI more adaptable and efficient.
Learn how convolutional neural networks and generative adversarial networks compare and how both deep learning models are used.